- 1Department of Behavioral Neuroscience, Oregon Health and Science University, Portland, OR, United States
- 2Portland Alcohol Research Center, Oregon Health and Science University, Portland, OR, United States
- 3Veterans Affairs Portland Health Care System, Portland, OR, United States
- 4Bioinformatics and Biostatistics Core, Oregon National Primate Research Center, Oregon Health and Science University, Beaverton, OR, United States
- 5Division of Genetics, Oregon National Primate Research Center, Oregon Health and Science University, Beaverton, OR, United States
- 6Integrated Genomics Laboratory, Oregon Health and Science University, Portland, OR, United States
- 7Division of Neuroscience, Oregon National Primate Research Center, Oregon Health and Science University, Beaverton, OR, United States
Male rhesus monkeys (n = 24) had a biopsy of prefrontal cortical area 46 prior to chronic ethanol self-administration (n = 17) or caloric control (n = 7). Fourteen months of daily self-administration (water vs. 4% alcohol, 22 h access/day termed “open-access”) was followed by two cycles of prolonged abstinence (5 weeks) each followed by 3 months of open-access alcohol and a final abstinence followed by necropsy. At necropsy, a biopsy of Area 46, contralateral to the original biopsy, was obtained. Gene expression data (RNA-Seq) were collected comparing biopsy/necropsy samples. Monkeys were categorized by drinking status during the final post-abstinent drinking phase as light (LD), binge (BD), heavy (HD) and very heavy (VHD drinkers). Comparing pre-ethanol to post-abstinent biopsies, four animals that converted from HD to VHD status had significant ontology enrichments in downregulated genes (necropsy minus biopsy n = 286) that included immune response (FDR < 9 × 10−7) and plasma membrane changes (FDR < 1 × 10−7). Genes in the immune response category included IL16 and 18, CCR1, B2M, TLR3, 6 and 7, SP2 and CX3CR1. Upregulated genes (N = 388) were particularly enriched in genes associated with the negative regulation of MAP kinase activity (FDR < 3 × 10−5), including DUSP 1, 4, 5, 6 and 18, SPRY 2, 3, and 4, SPRED2, BMP4 and RGS2. Overall, these data illustrate the power of the NHP model and the within-subject design of genomic changes due to alcohol and suggest new targets for treating severe escalated drinking following repeated alcohol abstinence attempts.
Introduction
AUD is diagnosed as a pattern of excessive alcohol consumption with repeated cycles of abstinence followed by resumption of heavy drinking, which in turn leads to negative psychological, physical, and social consequences [1]. This phenotype has been captured in a rhesus monkey model of alcohol self-administration using repeated cycles of chronic voluntary ethanol drinking and prolonged abstinence [2]. In this protocol, subjects are first induced to drink water and then ethanol (4% w/v) under a schedule-induced polydipsia (SIP) procedure. After induction, the monkeys given “open access” conditions for 22 h/day to choose between 45 ethanol. Given the voluntary nature of self-administration, the monkeys show a wide spectrum of individual differences in their daily intake ranging from an average of 0.3 g/kg/day up to 4 g/kg/day [3]. Based on mathematical modeling procedures and using data from six cohorts of monkeys with daily 22 h access to ethanol, four categorical levels of drinking were identified: light drinker (LD), binge drinker (BD), heavy drinker (HD) and very heavy drinker (VHD) [3, 4]. When the drinking protocol was extended to include three cycles of approximately 4–5 weeks of alcohol abstinence followed by 12–14 weeks of post-abstinence open-access conditions, alcohol intake generally increased following the reintroduction of alcohol (i.e., modeling resumption of heavy drinking) and gradually returned to pre-abstinence levels in all but a few monkeys [2]. The biological and behavioral features of this repeated abstinence protocol are described elsewhere [2, 5–7]. Overall, this repeated abstinence protocol has translational value for understanding the effects of cyclical chronic drinking and abstinence on subsequent drinking patterns.
The dorsolateral prefrontal cortex (DLPFC) is implicated in the return to heavy drinking in subjects with AUD, particularly in terms of volumetric analyses with magnetic resonance imaging (MRI) predicting sustained abstinence [8]. Indeed, subjects who returned to heavy drinking after 4 weeks of abstinence showed recovery of frontal cortical volume compared to subjects who maintained abstinence [8]. This suggests that atrophy of the DLPFC seen in relapsing AUD is due to underlying aberrant neuroadaptation to chronic heavy drinking. To address the molecular adaptations of the DLPFC in abstinence and just prior to resumption of heavy drinking, the present study utilized a biopsy of PFC area 46 before and following the protocol described above that ended with a final abstinence period (i.e., at necropsy). Rodent, nonhuman primate (NHP) and human postmortem studies have all clearly established the alignment of the brain transcriptome with ethanol consumption [9–13]. Rodent studies have been helpful in untangling risk genes and gene networks from those associated with the consequences of excessive ethanol exposure, as well as short-term abstinence (72 h) [14–18]. However, relatively little attention has been focused on the effects of protracted and/or repeated abstinence following chronic consumption. In addition, the rodent prefrontal cortex is underdeveloped compared to the primate brain. Thus, the current study offers a unique dataset addressing primate brain cortical adaptations and remodeling in abstinence from chronic alcohol consumption and just prior to resumption of heavy drinking. Finally, the data presented here offer a novel opportunity to examine how chronic ethanol exposure and repeated abstinence affect the NHP cortical transcriptome over a critical period of synaptic maturation, i.e., from late adolescence to young adulthood.
Methods and materials
Animals
Two replicate cohorts, named cohorts 10 and 14 in the Monkey Alcohol and Tissue Research Resource [MATRR] database [19], each containing 12 young adult male rhesus macaques were housed in quadrant cages (0.8 m × 0.8 m × 0.9 m) with constant temperature (20°C–22°C), humidity (65%), and an 11/13-h light/dark cycle. At the time of assignment, animals were between the ages of 4 and 6 years. Animals had visual, auditory, and olfactory contact with other animals in the protocol and were physically paired with another monkey for 1–2 h/day. All animals were maintained on positive caloric (i.e., weight gain) and fluid balance throughout the experiment, and body weights were recorded weekly. All procedures were conducted in accordance with the Guide for the Care and Use of Laboratory Animals and the NIH PHS Policy on Humane Care and Use of Laboratory Animals and approved by the Oregon National Primate Research Center (ONPRC) Institutional Animal Care and Use Committee (Protocol #0756). Animals in both cohorts were chosen from the ONPRC breeding colony to avoid common parents or grandparents. Cohort 10 contained 4 controls and 8 ethanol drinkers, and cohort 14 contained 3 controls and 9 ethanol drinkers. Detailed drinking and physiological data are available for these cohorts online.1
Ethanol subjects
Monkeys were trained to use operant drinking panels and underwent induction of ethanol self-administration in daily 16-h sessions using the SIP protocol as previously described [20]. Following induction, “open-access” conditions (22 h/day of a concurrent choice of drinking ethanol (4% w/v diluted in water or water) were scheduled every day for 60 weeks.). The details of the open-access protocol are discussed in Grant et al. [20], and the data modeling for drinking categories are presented in Baker et al. [3]. Ethanol and water intakes were recorded daily with a 0.5 s resolution, 22 h/day.
Control subjects
Yoked control subjects were housed in the same room as the ethanol-drinking subjects and participated in all experimental manipulations. For the controls, the SIP and self-administration conditions were identical, with the exception that both spouts dispensed water. A maltose dextrin solution (10% in water) was given to the controls in a volume calorically matched to intake by a weight-matched ethanol monkey. The dextrin solution was given at the beginning of each daily session by attaching a bottle to the front of the housing cage.
Abstinence
After daily open-access to ethanol, the first abstinence phase began. All independent variables remained constant except that the ethanol bottle was replaced with a second water bottle. Yoked controls no longer received daily maltodextrin during the abstinence phase. Abstinence 1 lasted for a total of 34 days, which included additional time for structural MRI scans (2 monkeys/day were scanned over the last week). At the end of the first abstinence, ethanol was again made available for the ethanol monkeys, and yoked maltose dextrin was reinstated for the control monkeys for approximately 11 weeks. Abstinence 2 followed and was 4 weeks for cohort 10 but extended to 7 weeks for cohort 14 in order to schedule and acquire fMRI (Table 1). After reinstating ethanol consumption for 14 weeks, abstinence 3 followed and was 34 days.
Biopsy/necropsy samples
MRI was used to obtain the stereotaxic coordinates for prefrontal cortical area 46 biopsies from the contralateral dominant hemisphere (determined by handedness). Tissue samples (35–50 mg wet weight) were taken under anesthesia (ketamine HCl 15 mg/kg; intramuscular) prior to ethanol exposure (hereafter noted as the Biopsy samples) and at necropsy; the necropsy samples were taken contralateral to the baseline Biopsy samples and were taken immediately before perfusion. Necropsy occurred at the end of the third abstinence period. Samples were immediately flash frozen in liquid nitrogen in a 2 mL cryovial and stored at −80°C for future processing. The pre-alcohol and necropsy biopsy samples were processed simultaneously using a standard Qiagen AllPrep DNA/RNA/miRNA Universal Kit protocol.
RNA-seq
Libraries were prepared using either the TruSeq RNA Sample Preparation kit (Illumina) or the TruSeq Stranded mRNA sample preparation kit (Illumina). Sequencing was performed on either a HiSeq 2500 (Illumina) or a NovaSeq 6000 (Illumina) at the Oregon Health and Science University Massively Parallel Sequencing Shared Resource. The quality of the raw sequencing files was evaluated using FastQC2 combined with MultiQC3 [21]. Trimmomatic [22] was used to remove any remaining Illumina adapters. Reads were aligned to Ensembl’s mmul10 along with its corresponding annotation, release 100. The program STAR [23] (v2.7.3a) was used to align the reads to the genome utilizing these parameters: --outFilterMismatchNmax 3 --outFilterMultimapNmax 1. STAR has been shown to perform well compared to other RNA-seq aligners [24]. Since STAR utilizes the gene annotation file, it also calculates the number of reads aligned to each gene. RNA-SeQC [25] and another round of MultiQC were utilized to ensure that alignments were of sufficient quality.
Gene-level differential expression (DE) analysis was performed in open-source software R (R Core Team4). Gene-level raw counts were filtered to remove genes with low counts in many samples following published guidelines [26], normalized using the trimmed mean of M-values method (TMM) [27], and transformed to log-counts per million with associated observational precision weights using the voom [28] method. Gene-wise linear models suited to the experimental design were employed for differential expression analyses using limma with empirical Bayes moderation [29] and false discovery rate (FDR) adjustment [30]. More specifically, in Necropsy vs. Biopsy Comparisons, separate models comparing Necropsy vs. Biopsy all adjust for Cohort and account for correlation within animals. In biopsy-only comparisons, because of the expected high biological variation (unlike the necropsy vs. biopsy comparisons), these comparisons do not have each animal as its own control. Surrogate variable analysis (SVA) [31] was performed to identify and adjust for any unknown sources of variation. Separate models comparing specified groups all adjust for cohort and surrogate variables identified through SVA.
The details of how the data were analyzed and the discrete sample sizes have been integrated into the results (see below). DE genes are commonly defined using statistical thresholds; because the degrees of gene expression profile change are expected to be different between the comparisons, different practical statistical thresholds were used to define DE genes to facilitate the identification of biological implications through pathway analyses (see below). Here, we note that the ontologies for various groups of differentially expressed genes were determined using the GOrilla algorithm [32] with human annotations; transcripts meeting the threshold of one transcript per million were used as the background reference. The significance level was set at FDR (q) < 0.05. Pathway analyses were performed in IPA [33] (QIAGEN Inc.5). Additional analysis details are found in the Supplementary Datasheet S1 as “Cohort 10 and 14 RNA-seq DE analysis, v9.”
Results
Sample characteristics
Twenty-four male rhesus macaques (7 controls and 17 drinkers) were entered into the analysis. Subjects were equally derived from 2 cohorts (10 and 14), separated experimentally by approximately 3 years. Biopsy/necropsy samples were obtained for all of the controls and 13 of the 17 drinkers. Four of the drinkers in cohort 10 underwent sham biopsy surgery; thus, only necropsy samples were obtained. The timeline for the progression of the subjects is illustrated in Figure 1. Additional details are found in Table 1. The data shown for the end of the initial 60 weeks of chronic ethanol access are average daily intakes over the last 30 days of open-access. The ethanol monkeys at this time point, were categorized as 3 LD, 5 BD, 4 HD and 5 VHD. MATRR subject IDs are found in the legend to Figure 1. There were no significant age differences among the groups. The drinking patterns after the second abstinence period revealed the following transitions from the end of the initial 60 weeks of open-access intakes: 4 BDs → HDs (# 10208, 10209, 10211, 10251), 2 LDs → HDs (#10213 10246) and 4 HDs → VHDs (# 10212, 10244, 10247, 10249). Five VHDs remained VHDs (#10214, 10215, 10242, 10248, 10252). (Note: the subject IDs in italics are the subjects for whom only a necropsy sample was available.)
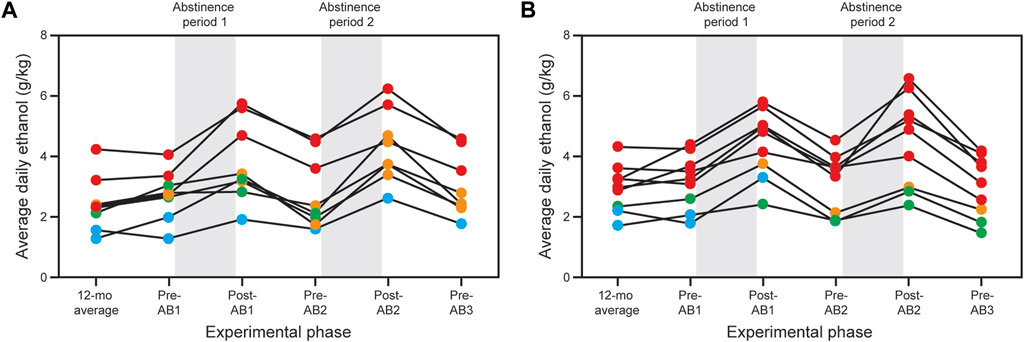
Figure 1. Effects of abstinence on ethanol consumption in cohort 10 (A) and cohort 14 (B). Both cohorts consumed alcohol in a choice paradigm for 12–14 months as described in the Methods and Grant et al. [20]. The final month of choice consumption was used to designate the subjects as light (blue), binge (green), heavy (orange) and very heavy (red) drinkers [see Baker et al. (2012) for detailed definitions of the drinking categories]. Both cohorts underwent three 1-month abstinence periods; the first two were followed by 3 months of standard choice consumption. Animals were sacrificed at the end of the third abstinence period (not illustrated in the figure). Additional details are found in Table 1.
Biopsy and necropsy independent comparisons
Three planned comparisons were made for the biopsy-only gene expression data: drinkers vs. controls, HD+VHD vs. LD+BD and VHD vs. HD. Drinking categories are those observed at the end of the period of voluntary consumption. The DE results for these comparisons are found in Supplementary Table S1. No significant DE genes were detected at the commonly used threshold of FDR < 0.2, suggesting minimal gene expression differences between these groups at biopsy. These data are also illustrated in Supplementary Data Sheet S1 as volcano plots and heatmap plots. Although this drinking protocol will not be repeated in future cohorts, biopsies from cohorts with different drinking protocols will be combined with these to increase sample sizes for a biopsy-only analysis in future studies. The drinkers vs. controls were also compared at necropsy; as indicated in Supplementary Table S1, there were no significant differences.
Necropsy vs. biopsy comparisons
The following comparisons were made for the necropsy vs. biopsy data: controls, controls vs. drinkers, HDs → VHDs, VHDs and BDs → HDs. The necropsy drinking categories are those seen after the second abstinence period. The comparison for the subjects who remained VHDs was included since, as noted in Figure 1, abstinence also increased consumption in this group. The DE results for the necropsy vs. biopsy comparisons are also found in Supplementary Table S1. For the drinker comparisons and given the small sample sizes, the FDR was relaxed to 0.2. Volcano plots, heatmaps and venn diagrams are provided in Supplementary Data Sheet S1.
Control comparison
The control (N = 7) data were collected over the period that spans late adolescence to early adulthood. Over this period, 269 genes were downregulated (necropsy < biopsy), and 456 were upregulated (necropsy > biopsy). The Gene Ontologies (GO) of these DE expressed genes are summarized in Supplementary Table S2. For the downregulated genes, the ontologies included the following: process: cell migration (FDR < 2 × 10−8); signal transduction (FDR < 5 × 10−5); cell adhesion (FDR < 1 × 10−4); function: transmembrane signaling receptor activity (FDR < 7 × 10−5); and component: plasma membrane part (FDR < 8 × 10−11). A schematic showing the relationships of the ontologies in the Process category is shown in Figure 2A. For the purpose of the latter discussion, we note here the detection of a weak (FDR < 0.01) immune response ontology (Figure 2A); genes in this ontology include Icam1, Prkcz, Hyal1 and 3, and Tlr7. Genes involved in signaling receptor activity included P2ry13, P2ry12, Tlr7, Grm3 and Htr6. However, note that P2ry12 and 13 are located in microglia and can be considered immune related genes. For the upregulated genes, the ontologies included the following: process: RNA processing (FDR < 4 × 10−6); function: nucleic acid binding (FDR < 2 × 10−8); and component: nuclear part (FDR < 1 × 10−7).
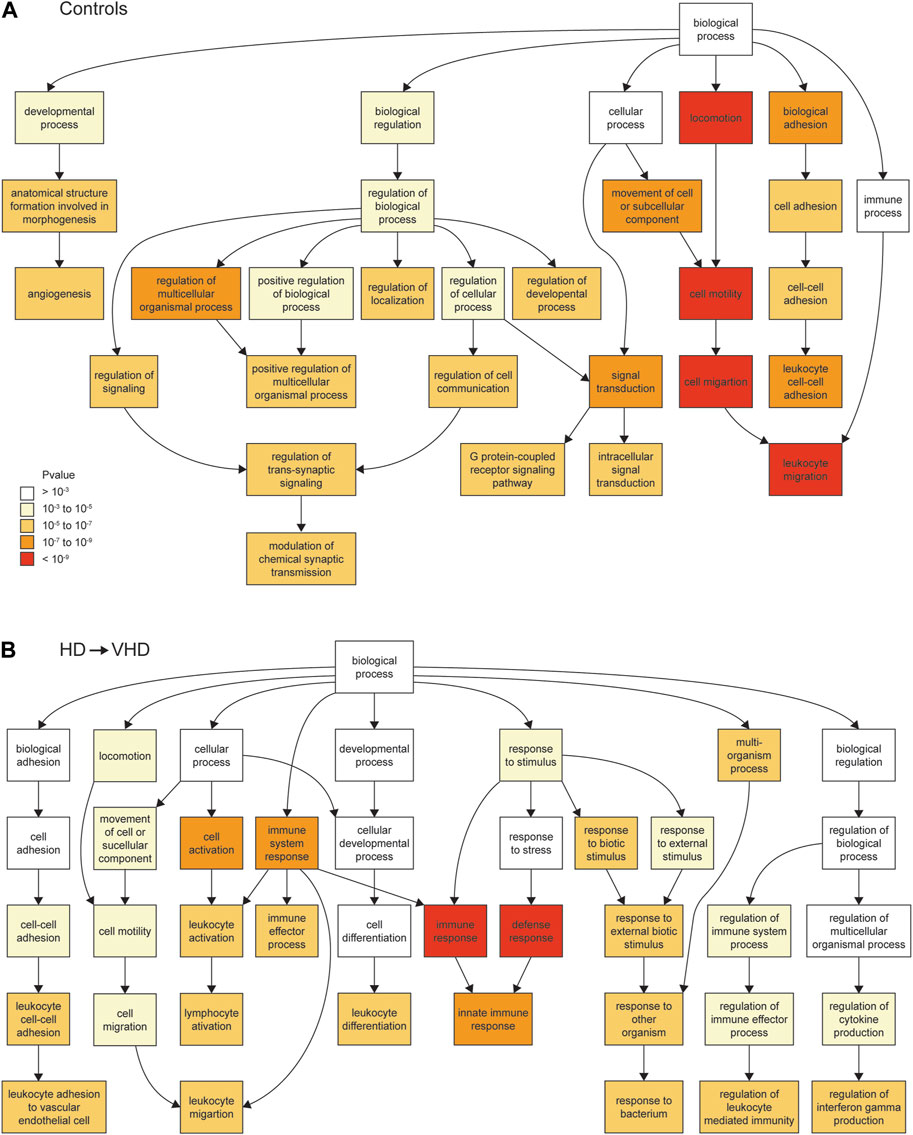
Figure 2. Biopsy/necropsy comparisons for controls [N = 7, (A)] and for HD → VHD [N = 4, (B)]. The ontologies in the figures are for the downregulated genes and in the ontology process category. The Gorilla algorithm (Aden et al. 2009) was used to detect the ontologies. The biopsy and necropsy samples differed temporally by approximately 3 years. The ontology categories are color coded according to the strength of the ontology (A). Note the difference in the strength of the immune response ontology between the controls and the HD → VHD subjects.
HD → VHD comparison
For the animals (N = 4) that converted from HD to VHD status during the abstinence protocol, 286 genes were downregulated and 388 were upregulated. Ontologies (Supplementary Table S2) for the downregulated genes included the following. Process: immune response (FDR < 9 × 10−7); and Component: plasma membrane part (FDR < 1 × 10−7). Genes in the immune response category included Il16 and 18, Ccr1, B2m, Tlr3, 6 and 7, Sp2 and Cx3cr1. Genes in the plasma membrane category included the purinergic receptors noted above. Figure 2B provides a schematic of the process ontologies; a comparison of Figures 2A, B illustrates the marked downregulation of immune-related genes in the HD → VHD group. Enriched ontologies for the upregulated genes included the following. Process: regulation of RNA metabolic process (FDR < 3 × 10−5) and negative regulation of MAP kinase activity (FDR < 3 × 10−5); Function: transcription regulatory region DNA binding (FDR < 2 × 10−3) and Component: glutamatergic synapse (FDR < 4 × 10−2). The negative regulation of MAP kinase activity is a new ontology not seen in the control group. Genes in this group included Dusp 1, 4, 5, 6 and 18, Spry 2, 3, and 4, Spred2, Bmp4 and Rgs2. Genes in the glutamatergic synapse category largely encoded accessory synaptic proteins but did include Grik3.
VHD → VHD comparison
As noted above, there were 5 VHDs that remained VHDs at the end of the abstinence protocols. One member of this group had only a necropsy sample that was included in the analyses. Fifty-eight genes were downregulated, and 94 were upregulated. For the downregulated genes, there were no significant (FDR < 0.05) GO terms. For the upregulated genes, significantly enriched ontologies included the following: Process: the negative regulation of MAP kinase activity (FDR < 2 × 10−6); and Function: protein kinase inhibitor activity (FDR < 1 × 10−2). Genes in the negative regulation of MAP kinase activity category were similar to those noted above and included Dusp4, 5 and 6, Rgs2, Spry2 and 3, Spred2 and Bmp4.
BD → HD comparison
There were 4 BD subjects who converted to HD at the end of the abstinence protocol. Biopsy samples were available for only 2 of these subjects. Four hundred ten genes were downregulated, and 252 genes were upregulated (necropsy vs. biopsy). For the downregulated genes, significantly enriched ontologies included the following. Process: signal transduction (FDR < 8 × 10−4), defense response (FDR < 2 × 10−2); Function: signaling receptor activity (FDR < 2 × 10−3); and Component: plasma membrane part (FDR < 3 × 10−11). Genes in the signaling receptor activity category included Arra2c, Ccr1, Gabrg1, Grin2c and Tlr7. For the upregulated genes, there was only one significant ontology. Process: regulation of RNA splicing (FDR < 4 × 10−2).
Controls vs. drinkers comparison
Controls and drinkers (at biopsy and necropsy) were compared. As illustrated in the venn diagrams and volcano plots (Supplementary Data Sheet S1), there were no significant differences between these groups.
Ingenuity pathway analysis (IPA)
IPA was used to examine enriched pathways in the control and HD→VHD biopsies to necropsy DE genes. Figures 3A, B compare the top canonical pathways in each group (Z score <−2 or >2). For the controls, 4/14 pathways had a Z score <−2, i.e., these pathways were predicted to be downregulated in the necropsy sample. These pathways included G-Protein Coupled Receptor Signaling, CREB Signaling in Neurons, Protein Kinase A Signaling and Phagosome Formation. The CREB signaling pathway is illustrated in Figure 4. The DE genes in this pathway included five adhesion G-protein coupled receptors (B2, E5, G1, L4 and V1), 3 alpha G-protein subunits (11, 14 and Z), 5 G-protein coupled receptors (6, 19, 34, 37L and 153), glutamate metabotropic receptor 3, 2 serotonin receptors (1E and 6) and the purinergic receptor Y12.
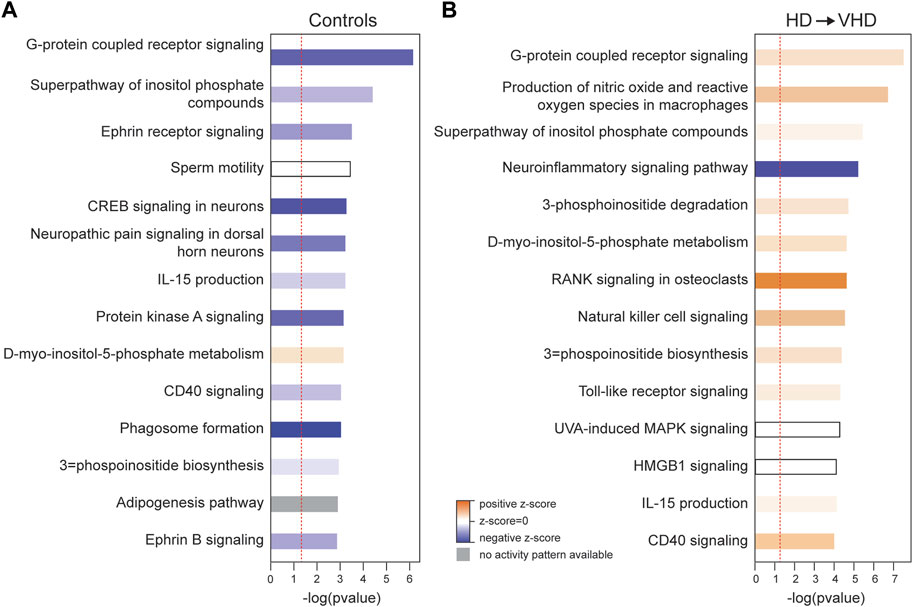
Figure 3. Ingenuity Pathway Analysis (IPA) of the biopsy/necropsy DE genes in the control (A) and HD→VHD (B) groups. Data entered into the analyses were both the up- and downregulated genes. The data presented are for the top 15 canonical pathways. Solid blue indicates pathways downregulated in the necropsy sample, and solid red indicates pathways upregulated in the necropsy sample. Similar to the data in Figure 2, IPA detected the downregulation of G-protein coupled receptor signaling in the controls and neuro-inflammatory signaling in the HD → VHD group.
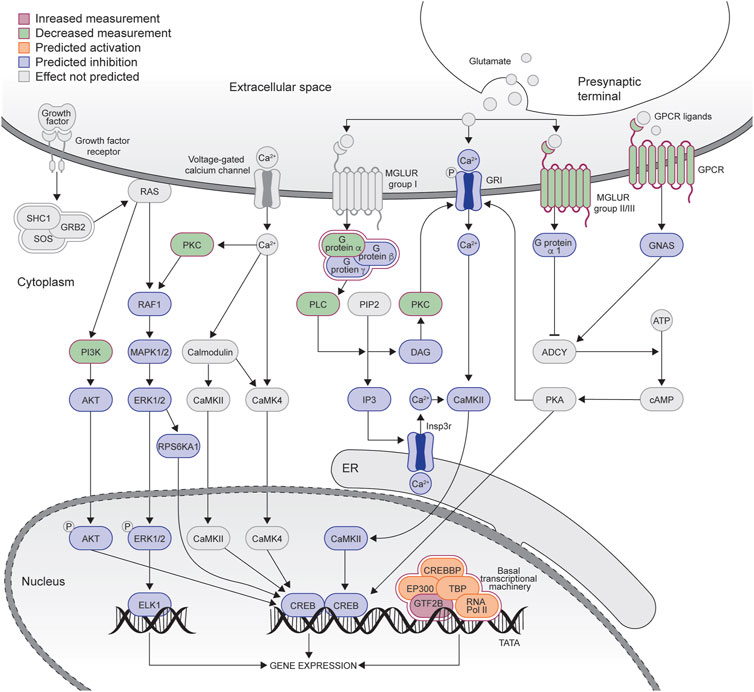
Figure 4. Graphical representation of the CREB signaling pathway downregulated in the control group (see Figure 3A). The graph was adapted from the graph generated by IPA. Blue coloring = downregulated genes, red coloring = upregulated genes. Green coloring = genes in the pathways that were not different between the biopsy/necropsy samples.
Figure 3B illustrates the marked effects of chronic ethanol consumption and repeated abstinence on the canonical pathways, e.g., the G-Protein Coupled Receptor Signaling pathway is now trending to a positive Z score. Emerging in the HD→VHD group is the neuroinflammation signaling pathway (Z < −2). Genes detected in this pathway included beta-2 microglobulin, interleukin 18, MAP kinases 13 & 14 and Toll-like receptors (3, 6 & 7). Figure 5 illustrates the co-expression patterns of these genes and closely related neuroimmune genes. The IPA generated neuroinflammation pathway is presented schematically in Supplementary Figure S1. It is important to note that the pathway includes elements from microglia, astrocytes and neurons.
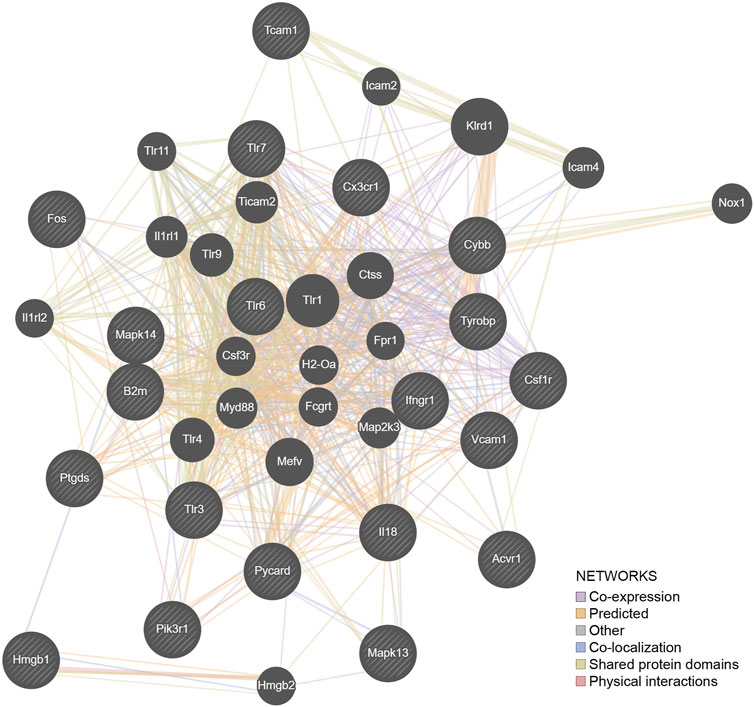
Figure 5. The genes (N = 21) detected by IPA as involved in the neuroimmune pathway were entered into a representation using the GeneMANIA algorithm. The hatched genes are these genes. The solid genes are those added by the algorithm to complete the pathways. Fifty percent of the network structure is associated with coexpression, and 20% is associated with predicted associations.
Another the top canonical pathways for the HD →. VHD group is RANK signaling in osteoclasts (Z > 2). There is no IPA pathway for brain RANK signaling. However, RANK signaling in the brain is well described [34], where it appears to serve multiple functions in the neuroinflammatory process, e.g., it is neuroprotective in TLR-mediated inflammation. DE genes in this pathway included mitogen-activated protein kinase kinases (3K4, 3K12, 3K13 and 3K14), mitogen-activated protein kinases (13 & 14) and NFKB-inhibitor delta.
Discussion
Walter et al. [35] were the first to use the within-subject biopsy/necropsy design to examine the effects of chronic open-access ethanol consumption on Area 46 gene expression. That study used cynomolgus macaques (6 controls and 17 drinkers), aged between 5 and 7 years, and the duration of the open-access phase was approximately 28 weeks, with no imposed abstinence. The key findings may be summarized as follows. Compared to the controls, a total of 675 genes were significantly downregulated following EtOH consumption; these genes were functionally enriched for immune response, cell adhesion, plasma membrane, and extracellular matrix. A total of 567 genes were significantly upregulated following EtOH consumption, were enriched in microRNA target sites, and included target sites associated with Toll-like receptor pathways. The differentially expressed genes were also significantly enriched in transcription factor binding sites. Discussion of these data emphasized the effects on the immune response genes, which were both pro- and anti-inflammatory, e.g., Il1r1 and Cd74 vs. Il6r and Il10ra. The overall inflammatory status of area 46 was not known, but the data suggested that in association with chronic ethanol consumption, there was a reset between the pro- and anti-inflammatory pathways. There is robust evidence of relationships between neuroimmune signaling and alcohol use disorder (e.g., [36–38]). Neuroinflammatory mechanisms are associated with both the risk for and individual variation in excessive EtOH consumption (e.g., [39, 40]). Furthermore, there is ample evidence that chronic EtOH consumption has a marked effect on the expression of cytokine ligands and receptors [41–45]. It should be noted that in Walter et al. [35] and the current study, the biopsy/necropsy samples were not perfused. Thus, the possibility must be considered that some of the gene expression signals, especially immune-related signals, are associated with blood cells. For example, Sureshchandra et al. [46] and Barr et al. [47], using gene expression profiling, found that in HD macaques, there was a disruption of innate immunity. However, the profiles of the blood genes affected included an enrichment in genes associated with wound healing and blood coagulation that was not seen in the brain data.
The possibility cannot be easily dismissed that the Area 46, which is part of the cognitive circuit involved in the control of alcohol consumption, does not fit the generally accepted model of the interaction between the neuroimmune system and alcohol consumption. However, we should also note that positron emission (PET) studies using 18F-deoxyglucose (FDG) during initial abstinence have revealed in humans that the prefrontal cortex is hypometabolic (Volkow et al. 1992). We assume, although unproven, that similar data would be obtained in the NHP model. It is possible that the neuroimmune genes are particularly sensitive to this metabolic malaise. The duration of the metabolic effect under the conditions of the present experiment is unknown.
The current study used the within-subject biopsy necropsy design, along with repeated cycles of abstinence, to examine transcriptional changes associated with ethanol intake and ADE. The study differs from Walter et al. [35] in several important aspects. 1) Male rhesus macaques rather than cynomolgus macaques were the study subjects; however, the age range was similar. 2) The initial open-access ethanol drinking phase was significantly longer (60 weeks vs. 28 weeks). An additional 52 weeks of open-access consumption (two post-abstinence open access periods of approximately 26 weeks each). 3) The necropsy sample was obtained after 34–38 days of withdrawal. Despite these differences, both studies detected an “immune response” signal. As noted above, in Walter et al. [35], the signal was detected in genes downregulated in drinkers but not controls. In the current study, the signal was detected in those subjects where the ADE moved the HD group to VHD status. The question naturally arises as to whether the immune response genes were similar. This is a hypothetical question that cannot be addressed satisfactorily given the differences in study design and given that the number of immune response genes detected in Walter et al. [35] was markedly larger (92 vs. 34) than the number detected in the current study. However, 13 of the genes were in common: C3, Csf1r, Ctss, Fgr, Ifi27, Il16, Irf8, Lyn, Ncf2, Pycard, Tyrobp, Vav1 and Was. With the exception of Lyn tyrosine kinase (Lyn), this core gene grouping would be considered proinflammatory. The colony stimulating factor 1 receptor (CSF1R) and its ligand (CSF1) are key to the maintenance and proliferation of microglia, the resident macrophages in the brain. Given the role(s) of immune systems in excessive ethanol consumption noted above, microglia are likely to have some role in this process. Fortunately, this hypothesis can be directly tested since CSF1R antagonists deplete brain microglia. Warden et al. [48, 49] examined this issue from two perspectives. They found that PLX5622-induced depletion of microglia did not affect the hypnotic or sedative effects of acute ethanol intoxication and did not affect the maintenance or escalation of voluntary ethanol consumption. However, microglial depletion blocked the poly (I:C)-induced escalation of ethanol consumption, suggesting that microglia have a role in ethanol consumption in the context of sufficient immune activation [48]. When they used PLX5622 to examine the role of microglia in chronic intermittent ethanol (CIE) vapor-induced escalation of ethanol consumption (2-bottle choice), microglia depletion blocked CIE-induced increases in ethanol consumption and blocked associated CIE-induced changes in gene expression [49]. The question naturally arises as to how to interpret the decrease in Csf1r expression observed in Walter et al. [35] and the current study. One can speculate that in macaques, the decreased expression is part of a compensatory mechanism, perhaps involving all of the genes noted above, in response to high levels of ethanol consumption.
Continuing the theme of compensatory mechanisms is the observation that the RANK signaling pathway was significantly upregulated in the necropsy vs. biopsy samples of the subjects that converted from HD to VHD. Key protein members of the pathway include receptor activator of nuclear factor-κB (RANK), the RANK ligand (RANKL), and osteoprotegerin (OPG), forming the RRO axis. OPG is a decoy receptor for RANKL. The involvement of the RRO axis in bone remodeling and peripheral immune responses is well established [50–53]. In the brain, the RRO axis tunes the neuroinflammatory response, depending on the molecular, cellular and pathological context. RANK/RANKL signaling is neuroprotective in TLR-mediated inflammation, which has been linked to ethanol-induced neuroinflammation (see, e.g., [54–57]). To our knowledge, this is the first report linking RANK signaling to excessive ethanol consumption. Given the large number of compounds and biologics (e.g., antibodies) that have been developed to affect bone remodeling via RANK signaling, some of these may be effective CNS therapies [34]. However, we also consider that the RANK signaling changes may be protective adaptations and not especially suitable as therapeutic targets. A similar argument could be raised for the changes in MAPK signaling (see below).
Given the widespread association of MAPK signaling with numerous brain mechanisms and pathways, it cannot be surprising to have detected an association between MAPK signaling and very heavy drinking. From a genomics perspective, Mulligan et al. [58], in a meta-analysis of microarray data, detected an association between MAPK signaling and ethanol preference. Subsequent studies (e.g., [59, 60]) have confirmed the association between Ras/MAPK pathways and preference consumption. Here, we focus on Ferguson et al. [10], who examined and reviewed 17 human postmortem studies involving a total of 230 cases and 233 controls; the data were derived from both microarray and RNAseq experiments and involved multiple brain regions. Upregulated KEGG pathways in cases included focal adhesion, MAPK signaling, cytokine-cytokine receptor interaction, cell adhesion and regulation of actin cytoskeleton. Downregulated KEGG pathways included oxidative phosphorylation, Parkinson’s disease and DNA replication. (See Table 2—Ferguson et al. [10]—for a complete list of up- and downregulated KEGG pathways). In the current study, we observed that VHD is associated with the upregulation of genes that negatively impact MAPK signaling. The signal was seen in both the HD→VHD and the VHD→VHD groups but not in the BD→HD group. Furthermore, the MAPK signal was not detected in Walter et al. [35], although the downregulation of immune-related genes was observed (see above). Overall, these data suggest that ADE leads to MAPK signaling. The question we cannot answer is whether there was an upregulation of MAPK genes [10] that preceded the upregulation of genes that would blunt such an effect. Is this another example of compensation? It is of interest that a core set of genes associated with the negative regulation of MAPK signaling was the same in the HD→VHD and VHD→VHD groups. The core set included dual specificity phosphatases, members of the sprouty/spred gene family, Rgs2 and Bmp4. Here, we note that the DUSP and SPRY/SPRED proteins have been suggested to be novel targets for CNS therapeutics [61, 62].
The current results provide new insights into the transcriptional changes that occur over the period from late adolescence to early adulthood (roughly 8–10 macaque years) and provide new information on how chronic ethanol consumption affects these transcriptional changes. Detailed information on the spatiotemporal dynamics of the developing NHP brain transcriptome is available (e.g., [63, 64]). However, these studies do not cover the period examined in the current study. The data presented here are clear that there are marked transcriptional changes that occur over young adulthood. The main caveat to these data is the procedures the animals encountered over the nearly 3-year duration of the study. The animals were anesthetized and underwent surgery. The pre-alcohol biopsy sample was removed in all but four low to binge drinkers, maltodextrin was administered chronically, and the animals were individually housed. The precise impact of these manipulations and circumstances are unknown. However, we observed significant downregulation of genes associated with cell migration, signal transduction and the plasma membrane in the control animals in biopsy compared to necropsy samples. Upregulated genes were associated with translation. In the BD→HD group, these ontologies were reduced, further reduced in the HD→VHD group and absent in the VHD→VHD group. Assuming that the transcriptional changes seen in the controls are at least, in part, signatures from late cortical developmental processes, ethanol plus the ADE reverses these changes in a dose-related fashion. What is not known is the persistence of the ethanol effects. That is, the data do not address if the absence of altered gene expression ontologies seen in ethanol drinkers compared to controls is representative of a “critical window” such that brain growth trajectories will not recover.
Given our understanding of the mechanisms associated with chronic ethanol intoxication and withdrawal in highly dependent individuals, the data presented here suggest that in the NHP brain, area 46 has relatively subtle adaptations, perhaps reflecting the relative homogeneity of the prefrontal molecular/granular layers examined here. The question then arises: do other brain regions drive excessive consumption? Previous studies (e.g., [65]) have suggested that the central nucleus of the amygdala (CeA) is key in regulating ethanol preference. Iancu et al. [11] aligned gene expression in Area 32 and the CeA with the drinking patterns in 31 rhesus macaques that had completed the standard 12-month open-access ethanol consumption protocol [20]. The CeA transcriptional data, compared to the cortical Area 32 data, aligned more significantly with the drinking pattern data. Membrane and synaptic genes, e.g., Chrm3, Chrna4, Chrna7, Glra2, Grm1 and Grm2, were especially enriched in the genes positively correlated with ethanol consumption. This signal was not observed in Area 46. Overall, the data presented here are part of a larger and ongoing effort [9, 11, 35, 66] to understand how chronic ethanol consumption affects the primate brain, including expanding the analyses to both males and females.
The data presented here have translational value for understanding the effects of voluntary chronic ethanol drinking, coupled with periods of involuntary abstinence, on subsequent drinking patterns. The data illustrate that across all levels of NHP ethanol consumption, imposed abstinence increased consumption when ethanol was reintroduced. The approximately 4 weeks of abstinence was modeled after the most common inpatient treatment protocol for severe AUD [8]. Escalated drinking, as seen in the return to heavy drinking following imposed abstinence, is a hallmark symptom of the addiction cycle [67] and is commonly modeled in rodents [68]. Furthermore, for many monkeys, the effects of repeated abstinence on ethanol intake escalated to higher steady state levels, for example, in the conversion of HDs to VHDs and the increase in consumption among the VHDs. As we hypothesize that the escalated drinking after abstinence cycles represent a model of the most severe AUD cases, we focused on the transcriptional effects of repeated abstinence to suggest or support new strategies for pharmacotherapeutic development that could be incorporated into abstinence-based therapies. As noted above, the within-subject DLPFC Area 46 transcriptional changes suggest that neuroinflammation mechanisms are prominent in the state of the brain about to return to heavy drinking (i.e., in prolonged abstinence just prior to resumed access to alcohol). Although neuroinflammation may be protective in some situations [69], recent data suggest that centrally acting anti-inflammatory drugs, such as the PDE4 inhibitor apremilast, could be beneficial in the treatment of AUD [70]. The data presented here suggest that drugs that affect MAPK and RANK signaling may also be effective, especially in therapeutic situations associated with involuntary abstinence.
Data availability statement
The raw fastq files for this dataset are available for download at NIH's Sequence Read Archive (https://www.ncbi.nlm.nih.gov/bioproject/PRJNA1050227/) under accession PRJNA1050227.
Ethics statement
The animal study was approved by the Oregon National Primate Research Center (ONPRC) Institutional Animal Care and Use Committee (IACUC). The study was conducted in accordance with the local legislation and institutional requirements.
Author contributions
RH and KG designed the studies and wrote the manuscript. NN, TC, and SG implemented the animal protocol; NN and RK were responsible for the tissue preparation; RS was responsible for library preparation and sequencing; LG, SF, KR, and RH analyzed the transcriptomics data; KV-C made the figures; TP and RC-J participated in analysis discussions and editing of the manuscript. All authors contributed to the article and approved the submitted version.
Funding
The Portland Alcohol Research Center, supported by NIH/NIAAA grant P60 AA010760, provided the majority of the funds for this research. Short read sequencing assays were performed by the OHSU Massively Parallel Sequencing Shared Resource. Bioinformatics support was provided by the Oregon National Primate Research Center Bioinformatics and Biostatistics Core, which is funded in part by NIH grant P51 OD011092. Additional support was received from AA 013484 (RH) and AA017134 (KG) and AA 013510 (KG), as well as the VA Research Career Scientist program, 15F-RCS-009 (TP).
Conflict of interest
The authors declare that the research was conducted in the absence of any commercial or financial relationships that could be construed as a potential conflict of interest.
Supplementary material
The Supplementary Material for this article can be found online at: https://www.frontierspartnerships.org/articles/10.3389/adar.2024.12528/full#supplementary-material
Footnotes
2http://www.bioinformatics.babraham.ac.uk/projects/fastqc/
5https://www.qiagenbioinformatics.com/products/ingenuity-pathway-analysis
References
1. Witkiewitz, K, Litten, RZ, and Leggio, L. Advances in the science and treatment of alcohol use disorder. Sci Adv (2019) 5(9):eaax4043. doi:10.1126/sciadv.aax4043
2. Allen, DC, Gonzales, SW, and Grant, KA. Effect of repeated abstinence on chronic ethanol self-administration in the rhesus monkey. Psychopharmacol (2018) 235(1):109–20. doi:10.1007/s00213-017-4748-9
3. Baker, EJ, Farro, J, Gonzales, S, Helms, C, and Grant, KA. Chronic alcohol self-administration in monkeys shows long-term quantity/frequency categorical stability. Alcohol Clin Exp Res (2014) 38(11):2835–43. doi:10.1111/acer.12547
4. Baker, EJ, Walter, NA, Salo, A, Rivas, PP, Moore, S, Gonzales, S, et al. Identifying future drinkers: behavioral analysis of monkeys initiating drinking to intoxication is predictive of future drinking classification. Alcohol Clin Exp Res (2017) 41(3):626–36. doi:10.1111/acer.13327
5. Jimenez, VA, Walter, NAR, Shnitko, TA, Newman, N, Diem, K, Vanderhooft, L, et al. Mifepristone decreases chronic voluntary ethanol consumption in rhesus macaques. J Pharmacol Exp Ther (2020) 375(2):258–67. doi:10.1124/jpet.120.000169
6. Salinas, AG, Mateo, Y, Carlson, VCC, Stinnett, GS, Luo, G, Seasholtz, AF, et al. Long-term alcohol consumption alters dorsal striatal dopamine release and regulation by D2 dopamine receptors in rhesus macaques. Neuropsychopharmacology (2021) 46(8):1432–41. doi:10.1038/s41386-020-00938-8
7. Sureshchandra, S, Raus, A, Jankeel, A, Ligh, BJK, Walter, NAR, Newman, N, et al. Dose-dependent effects of chronic alcohol drinking on peripheral immune responses. Sci Rep (2019) 9(1):7847. doi:10.1038/s41598-019-44302-3
8. Durazzo, TC, and Meyerhoff, DJ. GABA concentrations in the anterior cingulate and dorsolateral prefrontal cortices: associations with chronic cigarette smoking, neurocognition, and decision making. Addict Biol (2021) 26(3):e12948. doi:10.1111/adb.12948
9. Bogenpohl, JW, Smith, ML, Farris, SP, Dumur, CI, Lopez, MF, Becker, HC, et al. Cross-Species Co-analysis of prefrontal cortex chronic ethanol transcriptome responses in mice and monkeys. Front Mol Neurosci (2019) 12:197. doi:10.3389/fnmol.2019.00197
10. Ferguson, LB, Zhang, L, Kircher, D, Wang, S, Mayfield, RD, Crabbe, JC, et al. Dissecting brain networks underlying alcohol binge drinking using a systems genomics approach. Mol Neurobiol (2019) 56(4):2791–810. doi:10.1007/s12035-018-1252-0
11. Iancu, OD, Colville, A, Walter, NAR, Darakjian, P, Oberbeck, DL, Daunais, JB, et al. On the relationships in rhesus macaques between chronic ethanol consumption and the brain transcriptome. Addict Biol (2018) 23(1):196–205. doi:10.1111/adb.12501
12. Kapoor, M, Wang, JC, Farris, SP, Liu, Y, McClintick, J, Gupta, I, et al. Analysis of whole genome-transcriptomic organization in brain to identify genes associated with alcoholism. Transl Psychiatry (2019) 9(1):89. doi:10.1038/s41398-019-0384-y
13. Smith, ML, Lopez, MF, Wolen, AR, Becker, HC, and Miles, MF. Brain regional gene expression network analysis identifies unique interactions between chronic ethanol exposure and consumption. PLoS One (2020) 15(5):e0233319. doi:10.1371/journal.pone.0233319
14. Chen, WY, Chen, H, Hamada, K, Gatta, E, Chen, Y, Zhang, H, et al. Transcriptomics identifies STAT3 as a key regulator of hippocampal gene expression and anhedonia during withdrawal from chronic alcohol exposure. Transl Psychiatry (2021) 11(1):298. doi:10.1038/s41398-021-01421-8
15. Hashimoto, JG, Gavin, DP, Wiren, KM, Crabbe, JC, and Guizzetti, M. Prefrontal cortex expression of chromatin modifier genes in male WSP and WSR mice changes across ethanol dependence, withdrawal, and abstinence. Alcohol (2017) 60:83–94. doi:10.1016/j.alcohol.2017.01.010
16. Hitzemann, R, Bergeson, SE, Berman, AE, Bubier, JA, Chesler, EJ, Finn, DA, et al. Sex differences in the brain transcriptome related to alcohol effects and alcohol use disorder. Biol Psychiatry (2022) 91(1):43–52. doi:10.1016/j.biopsych.2021.04.016
17. Kozell, LB, Lockwood, D, Darakjian, P, Edmunds, S, Shepherdson, K, Buck, KJ, et al. RNA-seq analysis of genetic and transcriptome network effects of dual-trait selection for ethanol preference and withdrawal using SOT and NOT genetic models. Alcohol Clin Exp Res (2020) 44(4):820–30. doi:10.1111/acer.14312
18. van der Vaart, AD, Wolstenholme, JT, Smith, ML, Harris, GM, Lopez, MF, Wolen, AR, et al. The allostatic impact of chronic ethanol on gene expression: a genetic analysis of chronic intermittent ethanol treatment in the BXD cohort. Alcohol (2017) 58:93–106. doi:10.1016/j.alcohol.2016.07.010
19. Daunais, JB, Davenport, AT, Helms, CM, Gonzales, SW, Hemby, SE, Friedman, DP, et al. Monkey alcohol tissue research resource: banking tissues for alcohol research. Alcohol Clin Exp Res (2014) 38(7):1973–81. doi:10.1111/acer.12467
20. Grant, KA, Leng, X, Green, HL, Szeliga, KT, Rogers, LS, and Gonzales, SW. Drinking typography established by scheduled induction predicts chronic heavy drinking in a monkey model of ethanol self-administration. Alcohol Clin Exp Res (2008) 32(10):1824–38. doi:10.1111/j.1530-0277.2008.00765.x
21. Ewels, P, Magnusson, M, Lundin, S, and Käller, M. MultiQC: summarize analysis results for multiple tools and samples in a single report. Bioinformatics (2016) 32:3047–8. doi:10.1093/bioinformatics/btw354
22. Bolger, AM, Lohse, M, and Usadel, B. Trimmomatic: a flexible trimmer for Illumina sequence data. Bioinformatics (2014) 30(15):2114–20. doi:10.1093/bioinformatics/btu170
23. Dobin, A, Davis, CA, Schlesinger, F, Drenkow, J, Zaleski, C, Jha, S, et al. STAR: ultrafast universal RNA-seq aligner. Bioinformatics (2013) 29(1):15–21. doi:10.1093/bioinformatics/bts635
24. Engström, PG, Steijger, T, Sipos, B, Grant, GR, Kahles, A, Rätsch, G, et al. Systematic evaluation of spliced alignment programs for RNA-seq data. Nat Methods (2013) 10:1185–91. doi:10.1038/nmeth.2722
25. DeLuca, DS, Levin, JZ, Sivachenko, A, Fennell, T, Nazaire, M-D, Williams, C, et al. RNA-SeQC: RNA-seq metrics for quality control and process optimization. Bioinformatics (2012) 28(11):1530–2. doi:10.1093/bioinformatics/bts196
26. Chen, Y, Lun, ATL, and Smyth, GK. From reads to genes to pathways: differential expression analysis of RNA-Seq experiments using Rsubread and the edgeR quasi-likelihood pipeline. F1000Research (2016) 5:1438. doi:10.12688/f1000research.8987.1
27. Robinson, MD, and Oshlack, A. A scaling normalization method for differential expression analysis of RNA-seq data. Genome Biol (2010) 11(3):R25. doi:10.1186/gb-2010-11-3-r25
28. Law, CW, Chen, Y, Shi, W, and Smyth, GK. voom: precision weights unlock linear model analysis tools for RNA-seq read counts. Genome Biol (2014) 15(2):R29. doi:10.1186/gb-2014-15-2-r29
29. Ritchie, ME, Phipson, B, Wu, D, Hu, Y, Law, CW, Shi, W, et al. Limma powers differential expression analyses for RNA-sequencing and microarray studies. Nucleic Acids Res (2015) 43(7):e47. doi:10.1093/nar/gkv007
30. Benjamini, Y, Hochberg, Y, Benjamini, Y, and Hochberg, Y. Controlling the false discovery rate: a practical and powerful approach to multiple testing. J R Stat Soc B (1995) 57(1):289–300. doi:10.1111/j.2517-6161.1995.tb02031.x
31. Leek, JT, and Storey, JD. Capturing heterogeneity in gene expression studies by surrogate variable analysis. Plos Genet (2007) 3(9):1724–35. doi:10.1371/journal.pgen.0030161
32. Eden, E, Navon, R, Steinfeld, I, Lipson, D, and Yakhini, Z. GOrilla: a tool for discovery and visualization of enriched GO terms in ranked gene lists. BMC Bioinformatics (2009) 10:48. doi:10.1186/1471-2105-10-48
33. Krämer, A, Green, J, Pollard, JJ, Tugendreich, S, Pollard, J, Tugendreich, S, et al. Causal analysis approaches in ingenuity pathway analysis. Bioinformatics (2014) 30(4):523–30. doi:10.1093/bioinformatics/btt703
34. Glasnovic, A, O’Mara, N, Kovacic, N, Grcevic, D, and Gajovic, S. RANK/RANKL/OPG signaling in the brain: a systematic review of the literature. Front Neurol (2020) 11:590480. doi:10.3389/fneur.2020.590480
35. Walter, NAR, Zheng, CL, Searles, RP, McWeeney, SK, Grant, KA, and Hitzemann, R. Chronic voluntary ethanol drinking in cynomolgus macaques elicits gene expression changes in prefrontal cortical area 46. Alcohol Clin Exp Res (2020) 44(2):470–8. doi:10.1111/acer.14259
36. Coleman, LG, and Crews, FT. Innate immune signaling and alcohol use disorders. Handb Exp Pharmacol (2018) 248:369–96. \. doi:10.1007/164_2018_92
37. Cui, C, Shurtleff, D, and Harris, RA. Neuroimmune mechanisms of alcohol and drug addiction. Int Rev Neurobiol (2014) 118:1–12. doi:10.1016/B978-0-12-801284-0.00001-4
38. Harris, RA, and Koob, GF. The future is now: a 2020 view of alcoholism research. Neuropharmacology (2017) 122:1–2. doi:10.1016/j.neuropharm.2017.06.001
39. Iancu, OD, Colville, AM, Wilmot, B, Searles, R, Darakjian, P, Zheng, C, et al. Gender-specific effects of selection for drinking in the dark on the network roles of coding and noncoding RNAs. Alcohol Clin Exp Res (2018) 42(8):1454–65. doi:10.1111/acer.13777
40. Hitzemann, R, Oberbeck, D, Iancu, O, Darakjian, P, McWeeney, S, Spence, S, et al. Alignment of the transcriptome with individual variation in animals selectively bred for High Drinking-In-the-Dark (HDID). Alcohol (2017) 60:115–20. doi:10.1016/j.alcohol.2017.02.176
41. Erickson, EK, Blednov, YA, Harris, RA, and Mayfield, RD. Glial gene networks associated with alcohol dependence. Sci Rep (2019) 9(1):10949. doi:10.1038/s41598-019-47454-4
42. Montesinos, J, Pascual, M, Rodriguez-Arias, M, Minarro, J, and Guerri, C. Involvement of TLR4 in the long-term epigenetic changes, rewarding and anxiety effects induced by intermittent ethanol treatment in adolescence. Brain Behav Immun (2016) 53:159–71. doi:10.1016/j.bbi.2015.12.006
43. Montesinos, J, Alfonso-Loeches, S, and Guerri, C. Impact of the innate immune response in the actions of ethanol on the central nervous system. Alcohol Clin Exp Res (2016) 40(11):2260–70. doi:10.1111/acer.13208
44. Warden, AS, Azzam, M, DaCosta, A, Mason, S, Blednov, YA, Messing, RO, et al. Toll-like receptor 3 activation increases voluntary alcohol intake in C57BL/6J male mice. Brain Behav Immun (2019) 77:55–65. doi:10.1016/j.bbi.2018.12.004
45. Warden, AS, Azzam, M, DaCosta, A, Mason, S, Blednov, YA, Messing, RO, et al. Toll-like receptor 3 dynamics in female C57BL/6J mice: regulation of alcohol intake. Brain Behav Immun (2019) 77:66–76. doi:10.1016/j.bbi.2018.12.006
46. Sureshchandra, S, Rais, M, Stull, C, Grant, K, and Messaoudi, I. Transcriptome profiling reveals disruption of innate immunity in chronic heavy ethanol consuming female rhesus macaques. PLoS One (2016) 11(7):e0159295. doi:10.1371/journal.pone.0159295
47. Barr, T, Girke, T, Sureshchandra, S, Nguyen, C, Grant, K, and Messaoudi, I. Alcohol consumption modulates host defense in rhesus macaques by altering gene expression in circulating leukocytes. J Immunol (2016) 196(1):182–95. doi:10.4049/jimmunol.1501527
48. Warden, AS, Triplett, TA, Lyu, A, Grantham, EK, Azzam, MM, DaCosta, A, et al. Microglia depletion and alcohol: transcriptome and behavioral profiles. Addict Biol (2021) 26(2):e12889. doi:10.1111/adb.12889
49. Warden, AS, Wolfe, SA, Khom, S, Varodayan, FP, Patel, RR, Steinman, MQ, et al. Microglia control escalation of drinking in alcohol-dependent mice: genomic and synaptic drivers. Biol Psychiatry (2020) 88(12):910–21. doi:10.1016/j.biopsych.2020.05.011
50. Honma, M, Ikebuchi, Y, and Suzuki, H. RANKL as a key figure in bridging between the bone and immune system: its physiological functions and potential as a pharmacological target. Pharmacol Ther (2021) 218:107682. doi:10.1016/j.pharmthera.2020.107682
51. Ono, T, Hayashi, M, Sasaki, F, and Nakashima, T. RANKL biology: bone metabolism, the immune system, and beyond. Inflamm Regen (2020) 40:2. doi:10.1186/s41232-019-0111-3
52. Ono, T, and Nakashima, T. Recent advances in osteoclast biology. Histochem Cel Biol (2018) 149(4):325–41. doi:10.1007/s00418-018-1636-2
53. Udagawa, N, Koide, M, Nakamura, M, Nakamichi, Y, Yamashita, T, Uehara, S, et al. Osteoclast differentiation by RANKL and OPG signaling pathways. J Bone Min Metab (2021) 39(1):19–26. doi:10.1007/s00774-020-01162-6
54. Airapetov, MI, Eresko, SO, Bychkov, ER, Lebedev, AA, and Shabanov, PD. Prenatal exposure to alcohol alters TLR4 signaling in the prefrontal cortex in rats. Biomed Khim (2021) 67(6):500–6. doi:10.18097/PBMC20216706500
55. Crews, FT, Walter, TJ, Coleman, JLG, and Vetreno, RP. Toll-like receptor signaling and stages of addiction. Psychopharmacol (2017) 234(9–10):1483–98. doi:10.1007/s00213-017-4560-6
56. Mayfield, J, Ferguson, L, and Harris, RA. Neuroimmune signaling: a key component of alcohol abuse. Curr Opin Neurobiol (2013) 23(4):513–20. doi:10.1016/j.conb.2013.01.024
57. McCarthy, GM, Warden, AS, Bridges, CR, Blednov, YA, and Harris, RA. Chronic ethanol consumption: role of TLR3/TRIF-dependent signaling. Addict Biol (2018) 23(3):889–903. doi:10.1111/adb.12539
58. Mulligan, MK, Ponomarev, I, Hitzemann, RJ, Belknap, JK, Tabakoff, B, Harris, RA, et al. Toward understanding the genetics of alcohol drinking through transcriptome meta-analysis. Proc Natl Acad Sci U S A (2006) 103(16):6368–73. doi:10.1073/pnas.0510188103
59. Metten, P, Iancu, OD, Spence, SE, Walter, NA, Oberbeck, D, Harrington, CA, et al. Dual-trait selection for ethanol consumption and withdrawal: genetic and transcriptional network effects. Alcohol Clin Exp Res (2014) 38(12):2915–24. doi:10.1111/acer.12574
60. Colville, AM, Iancu, OD, Lockwood, DR, Darakjian, P, McWeeney, SK, Searles, R, et al. Regional differences and similarities in the brain transcriptome for mice selected for ethanol preference from HS-CC founders. Front Genet (2018) 9:300. doi:10.3389/fgene.2018.00300
61. Perez-Sen, R, Queipo, MJ, Gil-Redondo, JC, Ortega, F, Gomez-Villafuertes, R, Miras-Portugal, MT, et al. Dual-specificity phosphatase regulation in neurons and glial cells. Int J Mol Sci (2019) 20(8):1999. doi:10.3390/ijms20081999
62. Hausott, B, and Klimaschewski, L. Sprouty2-a novel therapeutic target in the nervous system? Mol Neurobiol (2019) 56(6):3897–903. doi:10.1007/s12035-018-1338-8
63. Bakken, TE, Miller, JA, Luo, R, Bernard, A, Bennett, JL, Lee, CK, et al. Spatiotemporal dynamics of the postnatal developing primate brain transcriptome. Hum Mol Genet (2015) 24(15):4327–39. doi:10.1093/hmg/ddv166
64. Bakken, TE, Miller, JA, Ding, SL, Sunkin, SM, Smith, KA, Ng, L, et al. A comprehensive transcriptional map of primate brain development. Nature (2016) 535(7612):367–75. doi:10.1038/nature18637
65. Dhaher, R, Finn, D, Snelling, C, and Hitzemann, R. Lesions of the extended amygdala in C57BL/6J mice do not block the intermittent ethanol vapor-induced increase in ethanol consumption. Alcohol Clin Exp Res (2008) 32(2):197–208. doi:10.1111/j.1530-0277.2007.00566.x
66. Farris, SP, Riley, BP, Williams, RW, Mulligan, MK, Miles, MF, Lopez, MF, et al. Cross-species molecular dissection across alcohol behavioral domains. Alcohol (2018) 72:19–31. doi:10.1016/j.alcohol.2017.11.036
67. Koob, GF, and Volkow, ND. Neurobiology of addiction: a neurocircuitry analysis. The lancet Psychiatry (2016) 3(8):760–73. doi:10.1016/S2215-0366(16)00104-8
69. Crews, FT, Robinson, DL, Chandler, LJ, Ehlers, CL, Mulholland, PJ, Pandey, SC, et al. Mechanisms of persistent neurobiological changes following adolescent alcohol exposure: NADIA consortium findings. Alcohol Clin Exp Res (2019) 43(9):1806–22. doi:10.1111/acer.14154
Keywords: rhesus macaque, abstinence from alcohol, prefrontal cortex, RNA-seq, ethanol
Citation: Hitzemann R, Gao L, Fei SS, Ray K, Vigh-Conrad KA, Phillips TJ, Searles R, Cervera-Juanes RP, Khadka R, Carlson TL, Gonzales SW, Newman N and Grant KA (2024) Effects of repeated alcohol abstinence on within-subject prefrontal cortical gene expression in rhesus macaques. Adv. Drug Alcohol Res. 4:12528. doi: 10.3389/adar.2024.12528
Received: 05 December 2023; Accepted: 12 April 2024;
Published: 26 April 2024.
Edited by:
Emmanuel Onaivi, William Paterson University, United StatesReviewed by:
Leon Coleman Jr., University of North Carolina at Chapel Hill, United StatesCopyright © 2024 Hitzemann, Gao, Fei, Ray, Vigh-Conrad, Phillips, Searles, Cervera-Juanes, Khadka, Carlson, Gonzales, Newman and Grant. This is an open-access article distributed under the terms of the Creative Commons Attribution License (CC BY). The use, distribution or reproduction in other forums is permitted, provided the original author(s) and the copyright owner(s) are credited and that the original publication in this journal is cited, in accordance with accepted academic practice. No use, distribution or reproduction is permitted which does not comply with these terms.
*Correspondence: Robert Hitzemann, aGl0emVtYW5Ab2hzdS5lZHU=; Kathleen A. Grant, Z3JhbnRrYUBvaHN1LmVkdQ==