- 1Department of Medical and Clinical Biophysics, Faculty of Medicine, Pavol Jozef Šafárik University, Košice, Slovakia
- 2Department of Infectology and Travel Medicine, Faculty of Medicine, Pavol Jozef Šafárik University and L. Pasteur University Hospital, Kosice, Slovakia
- 3Centre of Clinical and Preclinical Research—MEDIPARK, Faculty of Medicine, Pavol Jozef Šafárik University, Košice, Slovakia
- 4Department of Molecular Pathology and Biology, Faculty of Military Health Sciences, University of Defence, Hradec Kralove, Czechia
The immune response to SARS-CoV-2, the virus responsible for COVID-19, involves intricate interactions between immune cells and viral antigens. CD19+ lymphocytes play a critical role in driving the humoral immune response. In this study, high-throughput proteomic analysis was performed using tandem mass spectrometry to investigate the changes in proteomic profiles of CD19+ whole cell lysates from 6 healthy individuals and 6 acute COVID-19 patients. The volcano plot and heat map showed significant differences in proteomic profiles between these two groups, indicating a distinct molecular signature associated with acute COVID-19. Enrichment analysis, especially over-representation analysis (ORA) using the Reactome database, revealed that proteins involved in neutrophil degranulation and interferon alpha/beta signaling pathways were among the most affected, indicating alterations in key defense processes. These findings, therefore, provide new insights into the molecular mechanisms underlying CD19+ cell responses in acute COVID-19.
Introduction
The recent COVID-19 pandemic has posed major challenges to global health. As of 24 May 2023, nearly 770 million confirmed cases of COVID-19 (COronaVIrus Disease-2019) have been reported to the WHO, including 6,935,889 deaths. The binding of the virus to the angiotensin-converting enzyme 2 receptor, found in various tissues (Leyfman et al., 2020), leads to dysregulation of the immune response, resulting in a cytokine storm, platelet activation, and the initiation of a complement cascade. Various indicators are used to assess the severity of COVID-19, including the neutrophil-to-lymphocyte ratio (Lagunas-Rangel, 2020), IL-6 levels (Rutkowska et al., 2021; Santa Cruz et al., 2021), and the concentration of chemokines with C-X-C motif 13 (CXCL13) (Horspool et al., 2020). However, direct quantification of these indicators in serum by mass spectrometry is limited due to their low concentration (Huynh et al., 2022). For instance, the threshold of 35 pg/mL for IL-6 increased the risk of death and severity of pneumonia in COVID-19 patients (Guirao et al., 2020).
Despite advancements in understanding the pathophysiology of infection, technological enhancements, and the development of faster viral and antibody detection methods or new opportunities for effective therapy, there remains a significant challenge in accurately identifying patients with the highest risk of progressing to a severe clinical condition. The underlying mechanisms of disease progression also remain unclear, despite previous vaccination efforts. In addition, there is a lack of precise identification of COVID-19 patients who are at risk of life-threatening infectious complications, such as other viral, bacterial, or fungal infections, coinfections, or superinfections that are common in critically ill patients (Salazar et al., 2022).
However, it is widely recognized that infection events activate host factors that may exhibit some degree of specificity towards the invading pathogens. In view of the aforementioned studies, it becomes crucial to identify clinical characteristics with specific values that can determine the stage of COVID-19 and differentiate between mild to moderate and severe to critical infections. Lymphocytes play a central and pivotal role in immune defense against pathogens and in regulating immune responses, including protective responses against pulmonary pathogens (Delves and Roitt, 2000b; Delves and Roitt, 2000a; von Andrian and Mackay, 2000). The emergence of proteomics, and bioinformatics has facilitated the characterization of protein profiles in lymphocytes, which may help to better understand the pathogenesis of this infection and explore functional interactions between proteins and disease development (Wang and Liotta, 2011; Abraham et al., 2012). In this article, we examined changes in the proteomics profiles of CD19+ B cells in acute COVID-19 patients.
Methods
Study cohort and sample collection
A cohort of 6 healthy individuals and 6 acute COVID-19 patients, without any other comorbidities, were recruited for this study from 1 June 2022, to 10 May 2023, at the L. Pasteur University Hospital in Kosice, Slovakia. Patients with SARS-CoV-2 were identified as positive through an RT-PCR assay using nasopharyngeal swab specimens, following the WHO guidelines. Peripheral blood samples were collected from each participant.
Isolation of CD19+ B lymphocytes
Peripheral blood samples (10 mL) were diluted threefold with the isolation solution (PBS containing 0.1% BSA and 2 mM EDTA at pH 7.4 and lacking Mg2+ and Ca2+ ions). Following dilution, the blood was centrifuged at 600×g for 10 min at 4°C, resulting in the separation of plasma. The CD19+ B lymphocytes were isolated using magnetic bead-based cell sorting by following a recommended protocol provided by the manufacturer (Invitrogen™ Dynabeads™ CD19 Pan B). The magnetic beads with attached CD19+ cells underwent three washes with the isolation solution prior to further use.
Isolated CD19+ B lymphocyte lysis
The isolated CD19+ B lymphocytes were subjected to three cycles of sonication and vortexing to promote efficient lysis. Each cycle consisted of a sequence of the following steps: 15 min in an ultrasonic bath (Elma Ultrasonic P30h, sweep mode on, 37 kHz, 90% power, 21°C); 5 min of vortexing (Biosan, V-32 Multi-Vortex) in a refrigerator at 4°C; 15 min of sonication in a VialTweeter block (Hielscher Ultrasonic Homogenizer VialTweeter, ampl. 85%, 0.5 s pulse); 5 min of vortexing in a refrigerator at 4°C. Subsequently, the magnetic beads were collected using a magnet. The lysate was then carefully removed and ethanol was added in a 1:9 (v/v) ratio to facilitate protein precipitation. The mixture was then incubated overnight at −20°C to promote gradual protein aggregation and sedimentation. On the next day, the sample was centrifugated at 18,000 x g for 20 min at 4°C. The supernatant was discarded, and the protein pellet was dried. Subsequently, the pellet was resuspended in 50 mM NH4HCO3. To determine the protein concentration required for in-solution digestion, the Pierce BCA Protein Assay was employed.
Proteins digestion
Dithiothreitol was added to each sample, reaching a final concentration of 4.5 mM. The samples were then incubated in a thermomixer (Eppendorf ThermoMixer™ C) at 60°C and 850 rpm for 30 min. Subsequently, iodoacetamide was added at a final concentration of 11 mM and incubated in darkness at 21°C for 30 min. Finally, a 5 mM solution of CaCl2 and trypsin solution were added at a trypsin-to-sample ratio of 1:40 (w/w). The sample was incubated overnight at 21°C. On the next day, the samples were acidified using 20% formic acid to stop the protein cleavage, and the volumes of the samples were adjusted in a vacuum concentrator (Labconco SpeedVac System) at 55°C and 1,000 rpm to achieve the desired final protein concentration.
High throughput proteomics analysis
The prepared samples were loaded onto the trap column (PepMap™ Neo Trap Cartridge, Thermo Scientific™, 5 mm, C18, 5 μm) of the Vanquish Neo (Thermo Scientific™) nano UHPLC system equipped with a heated trap and eluted by backward flush at the flow rate of 60 μL/min. The peptides were then separated using an analytical C18 column (EASY-Spray™ HPLC Column; Thermo Scientific™; 75 μm × 50 cm; 2 μm; C18; 100 Å) by a two-step gradient at the flow rate of 250 nL/min; the mobile phases consisted of (solvent A) 0.1% formic acid, and (solvent B) 80% acetonitrile, 0.1% formic acid. In the first, the gradient rose from 2% to 24% solvent B (80% acetonitrile in 0.1% formic acid) in 100 min and from 24% to 40% over the next 20 min. Column wash followed for 10 min with 90% solvent B and the column was re-equilibrated with 2% solvent B for 15 min. MS data were acquired by the Orbitrap Exploris 480 mass spectrometer (Thermo Scientific™), equipped with a nanoelectrospray source. Full-scan spectra (350–1700 m/z) were recorded in the Orbitrap at a resolution of 120,000 at 200 m/z every 2 s. MS/MS data were recorded in a data-dependent manner, fragmenting as many of the most abundant ions (with charge states of +2 to +5) as possible between the two master MS scans using higher-energy C-trap dissociation (HCD) in the spectrometer’s ion routing multipole at 30% collision energy. MS/MS spectra were recorded with a maximum injection time set to 50 ms, and dynamic exclusion (60 s) to minimize repeated fragmentation of the same peptides.
Protein identification and relative quantification
The generated RAW files were analyzed using Proteome Discoverer (PD) software, version 2.5 (Thermo Scientific™). Protein identification was performed using the SequestHT search engine with the following settings: fixed modification of carbamidomethyl (cysteine), and variable modifications including oxidation (methionine), acetyl (protein N-terminal), Met-loss (methionine), and Met-loss + acetyl (methionine). The protein identification searches were conducted against the Uniprot Homo sapiens database (release 2023_02), which contained 182,026 sequences. Trypsin was specified as a proteolytic enzyme, cleaving after lysine (K) and arginine (R) except when followed by proline (P), and up to two missed cleavages were considered. Mass tolerance was limited to 10 ppm at the precursor and 0.02 Da at the fragment level. The identified spectra were rescored using Percolator (The et al., 2016), implemented in PD. Subsequently, filtering was applied at both the peptide spectrum match and peptide level, maintaining a false discovery rate (FDR) threshold of 1% for each match. For Label-Free Quantification (LFQ), the precursor abundance in the samples was compared based on the integration of peak areas under the curve of the identified peptides. This integration provided a measure of the relative abundance. To determine the statistical significance of the LFQ results, a t-test was employed, enabling the identification of proteins that exhibited significant differences in abundance (0.5 ≥ abundance ratio ≥2; adjusted p-value ≤0.05) within experimental conditions. The resulting abundance ratios, along with their corresponding p-values, can be found in the supplementary table, providing comprehensive information on the significant proteins identified in the analysis.
Bioinformatics analysis
The PD software facilitated the identification of proteins that were either up- or downregulated, as depicted in the heat map and volcano plot (Figures 1, 2, respectively). The heat map enables the identification of quantitative patterns across proteins and biological samples simultaneously. Additionally, the volcano plot combines a measure of statistical significance from a statistical test with the magnitude of the changes. The proteins that exhibited differential expression between the two groups were subjected to enrichment analysis. It was conducted to identify significantly enriched biological processes, molecular functions, and cellular components. All the MS proteomics data utilized in this study have been deposited to the ProteomeXchange Consortium via the PRIDE (Perez-Riverol et al., 2022) partner repository with the dataset identifier PXD042938.
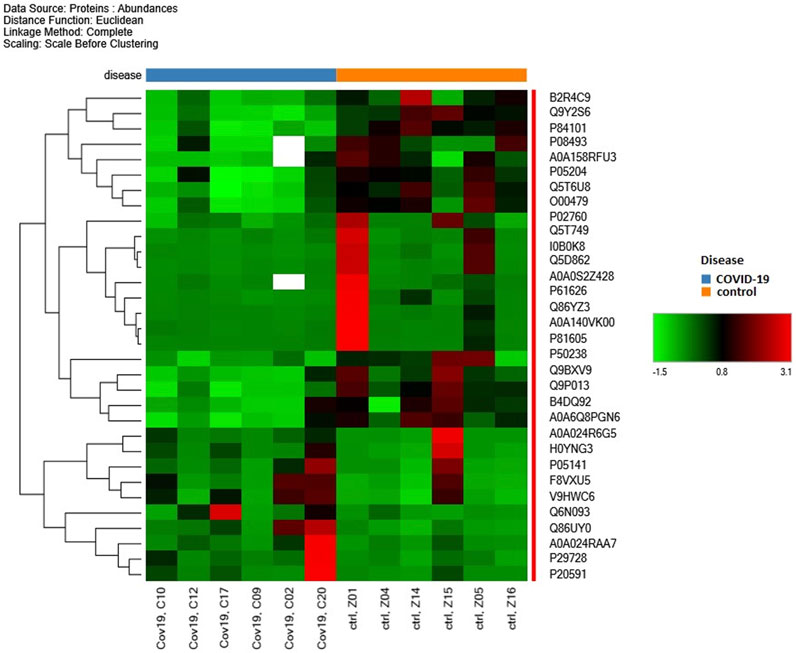
FIGURE 1. Heat map of significant alteration of proteins during acute non-severe COVID-19 infection of six human patients in contrast to six healthy individuals.
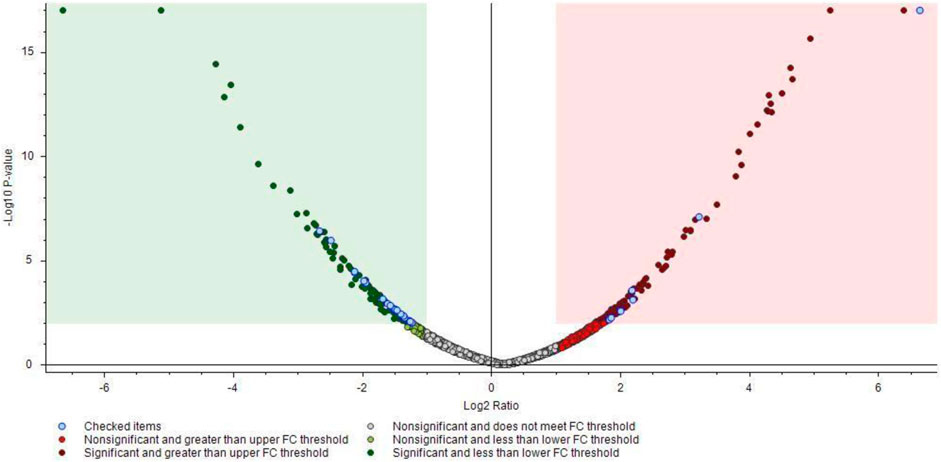
FIGURE 2. Volcano plot showing proteomics data. These points indicate different proteins that display both large magnitude fold-changes (Log2 Ratio, x axis) and high statistical significance (−log10 of p values, y axis). The green and red squares show down/upregulated proteins bellow the p values cutoff. Transparent points in the significant region mean these proteins do not satisfy some other conditions.
Results
In this initial study, we identified 4,849 proteins in CD19+ B lymphocytes in response to acute COVID-19 and investigated changes in their proteomics profiles. The heat map (Figure 1) shows the distribution of selected proteins from the isolated samples of 6 acute non-severe COVID-19 patients and 6 healthy individuals. At first glance, significant changes were observed between these two groups, especially from B2R4C9 to O00479 and then from P50238 to A0A6Q8PGN6 (Figure 1). Among them, cysteine-rich protein 1 (Crip1) helps transforming growth factor beta (TGF-β) inhibit type 1 T helper (Th1) and type 2 T helper (Th2) differentiation, is involved in angiotensin converting enzyme-2 (ACE2) expression, and is downregulated by SARS-CoV-2 (Hachim et al., 2020; Wang et al., 2020). Also, translation machinery-associated protein 7 (TAM7) was specifically downregulated in COVID-19 patients with persistent SARS-CoV-2 infection, i.e., long duration of viral shedding (LDs) (Yang et al., 2021). Moreover, in our study, Crip1 and TAM7 proteins were downregulated (approximately 3-fold) in patients with COVID-19 compared to the control group (Supplementary Table S1). The volcano plot further visualizes protein identifications with large and statistically significant fold changes (Figure 2). There were 32 differentially abundant proteins (>2-fold changes; p < 0.01) identified in the SARS-COV-2 positive group (Supplementary Table S1). Of these, 10 proteins were highly accumulated, and 22 proteins were downregulated. The changes in these proteomic profiles indicate B cell activation and shed light on the molecular mechanisms underlying the immune response to SARS-CoV-2 infection. Thus, these findings suggest a unique molecular signature associated with acute COVID-19 disease.
Discussion
Enrichment analysis revealed enrichment in two major pathways to combat microbial infections: neutrophil degranulation and interferon alpha/beta (IFN α/β) signaling. For instance, the interferon-induced GTP-binding protein Mx1 was accumulated by more than 3.5-fold. This protein is responsible for a specific antiviral defense against a broad spectrum of viral infections that are induced by type-I interferons (IFN-I) which play a critical role in the initiation of the adaptive immune response (Ng et al., 2016). It was shown that human viral infections with HIV, hepatitis B virus (HBV), and hepatitis C virus (HCV) are associated with elevated IFN-signaling (Bolen et al., 2013; Doyle et al., 2015). The hallmarks of HIV, HBV, and HCV, and persistent viral infection in mice with lymphocytic choriomeningitis virus (LCMV) include the generation of negative immune-regulating (NIR) molecules that suppress antiviral CD4 and CD8 T cells responses, resulting in decreased T cell function (T cell exhaustion). On the other hand, early clearance of acute viral infection was associated with enhancement of antiviral CD4 and CD8 T cells (Thimme et al., 2003). Therefore, it seems that IFN-signaling may play a deciding role in generating the hyperimmune environment accompanying viral infections.
In conclusion, more accurate knowledge was gained about the role of lymphocytes in the pathogenesis of the disease. The high-throughput proteomics analysis of CD19+ B lymphocytes in acute COVID-19 patients revealed significant differences in protein expression profiles compared to healthy individuals. Enrichment of proteins involved in neutrophil degranulation and interferon alpha/beta signaling pathways was noticed. They can be potential targets for therapeutic interventions. Nevertheless, further studies are needed to elucidate the functional consequences of proteomics alterations in the peripheral blood leukocyte subset in relation to disease prognosis, severity, and clinical outcomes. This study is ongoing.
Data availability statement
The datasets presented in this study can be found in online repositories. The names of the repository/repositories and accession number(s) can be found below: https://www.ebi.ac.uk/pride/archive/, PXD042938.
Ethics statement
The studies involving human participants were reviewed and approved by Ethics Committee of Medical Faculty UPJŠ, protocol code 16N/2022, date of approval 25 May 2022, Ethics Committee of L. Pasteur University Hospital code 2021/EK/06041, date of approval 21 June 2021 and code 2022/EK/03016, date of approval 21 March 2022. The patients/participants provided their written informed consent to participate in this study.
Author contributions
MM—sample preparation technique development, manual sample preparation, mass spectrometry data acquisition, bioinformatic data processing. OZ—suitable patient selection, sample collection, assessment of patients’ health status. MK—sample preparation technique development. MŠ—sample preparation technique development. MB-Ď—bioinformatic data processing, proteomic data analysis, finalization of the results. PŘ—bioinformatic data processing, proteomic data analysis, finalization of the results. PB—sample preparation. JF—suitable patient selection, sample collection, assessment of patients’ health status. PJ—suitable patient selection, sample collection, providing valuable clinical insights. JS—concept of the study, design of the experiments, preparation of the manuscript. All authors contributed to the article and approved the submitted version.
Funding
This publication was supported by grant AKARDIO COVID-19, ITMS: 313011AUB1, Operational Program Integrated Infrastructure, co-founded by European Regional Development Fund.
Acknowledgments
The authors thank the L. Pasteur University Hospital in Kosice, Slovakia for kindly providing the clinical samples.
Conflict of interest
The authors declare that the research was conducted in the absence of any commercial or financial relationships that could be construed as a potential conflict of interest.
Supplementary material
The Supplementary Material for this article can be found online at: https://www.frontierspartnerships.org/articles/10.3389/av.2023.11702/full#supplementary-material
References
Abraham, E., Marincola, F. M., Chen, Z., and Wang, X. (2012). Clinical and translational medicine: integrative and practical science. Clin. Transl. Med. 1 (1), 1. doi:10.1186/2001-1326-1-1
Bolen, C. R., Robek, M. D., Brodsky, L., Schulz, V., Lim, J. K., Taylor, M. W., et al. (2013). The blood transcriptional signature of chronic hepatitis C virus is consistent with an ongoing interferon-mediated antiviral response. J. Interferon Cytokine Res. 33 (1), 15–23. doi:10.1089/jir.2012.0037
Delves, P. J., and Roitt, I. M. (2000a). The immune system. First of two parts. N. Engl. J. Med. 343 (1), 37–49. doi:10.1056/NEJM200007063430107
Delves, P. J., and Roitt, I. M. (2000b). The immune system. Second of two parts. N. Engl. J. Med. 343 (2), 108–117. doi:10.1056/NEJM200007133430207
Doyle, T., Goujon, C., and Malim, M. H. (2015). HIV-1 and interferons: who’s interfering with whom?. Nat. Rev. Microbiol. 13, 403–413. doi:10.1038/nrmicro3449
Guirao, J. J., Cabrera, C. M., Jiménez, N., Rincón, L., and Urra, J. M. (2020). High serum IL-6 values increase the risk of mortality and the severity of pneumonia in patients diagnosed with COVID-19. Mol. Immunol. 128, 64–68. doi:10.1016/J.MOLIMM.2020.10.006
Hachim, I. Y., Hachim, M. Y., Talaat, I. M., López-Ozuna, V. M., Sharif-Askari, N. S., Al Heialy, S., et al. (2020). The molecular basis of gender variations in mortality rates associated with the novel coronavirus (COVID-19) outbreak. Front. Mol. Biosci. 17 (8), 728409. doi:10.3389/fmolb.2021.728409
Horspool, A. M., Kieffer, T., Russ, B. P., DeJong, M. A., Wolf, M. A., Karakiozis, J. M., et al. (2020). Interplay of antibody and cytokine production reveals CXCL-13 as a potential novel biomarker of lethal SARS-CoV-2 infection. mSphere 6 (1), e01324–20. doi:10.1128/mSphere.01324-20
Huynh, H. H., Delatour, V., Derbez-Morin, M., Liu, Q., Boeuf, A., and Vinh, J. (2022). Candidate high-resolution mass spectrometry-based reference method for the quantification of procalcitonin in human serum using a characterized recombinant protein as a primary calibrator. Anal. Chem. 94 (10), 4146–4154. doi:10.1021/ACS.ANALCHEM.1C03061
Lagunas-Rangel, F. A. (2020). Neutrophil-to-lymphocyte ratio and lymphocyte-to-C-reactive protein ratio in patients with severe coronavirus disease 2019 (COVID-19): a meta-analysis. J. Med. Virol. 92 (10), 1733–1734. doi:10.1002/JMV.25819
Leyfman, Y., Erick, T. K., Reddy, S. S., Galwankar, S., Nanayakkara, P. W. B., Di Somma, S., et al. (2020). Potential immunotherapeutic targets for hypoxia due to COVI-flu. Shock 54 (4), 438–450. doi:10.1097/SHK.0000000000001627
Ng, C. T., Mendoza, J. L., Garcia, K. C., and Oldstone, M. B. A. (2016). Alpha and beta type 1 interferon signaling: passage for diverse biologic outcomes. Cell 164 (3), 349–352. doi:10.1016/J.CELL.2015.12.027
Perez-Riverol, Y., Bai, J., Bandla, C., García-Seisdedos, D., Hewapathirana, S., Kamatchinathan, S., et al. (2022). The PRIDE database resources in 2022: a hub for mass spectrometry-based proteomics evidences. Nucleic Acids Res. 50 (D1), D543–D552. doi:10.1093/nar/gkab1038
Rutkowska, E., Kwiecień, I., Żabicka, M., Maliborski, A., Raniszewska, A., Kłos, K., et al. (2021). Cytokines and leukocytes subpopulations profile in SARS-CoV-2 patients depending on the CT score severity. Viruses 13 (5), 880. doi:10.3390/V13050880
Salazar, F., Bignell, E., Brown, G. D., Cook, P. C., and Warris, A. (2022). Pathogenesis of respiratory viral and fungal coinfections. Clin. Microbiol. Rev. 35 (1), e0009421–21. doi:10.1128/CMR.00094-21
Santa Cruz, A., Mendes-Frias, A., Oliveira, A. I., Dias, L., Matos, A. R., Carvalho, A., et al. (2021). Interleukin-6 is a biomarker for the development of fatal severe acute respiratory syndrome coronavirus 2 pneumonia. Front. Immunol. 12, 613422. doi:10.3389/FIMMU.2021.613422
The, M., MacCoss, M. J., Noble, W. S., and Käll, L. (2016). Fast and accurate protein false discovery rates on large-scale proteomics data sets with percolator 3.0. J. Am. Soc. Mass Spectrom. 27 (11), 1719–1727. doi:10.1007/S13361-016-1460-7
Thimme, R., Wieland, S., Steiger, C., Ghrayeb, J., Reimann, K. A., Purcell, R. H., et al. (2003). CD8+ T cells mediate viral clearance and disease pathogenesis during acute hepatitis B virus infection. J. Virol. 77 (1), 68–76. doi:10.1128/JVI.77.1.68-76.2003
von Andrian, U. H., and Mackay, C. R. (2000). T-cell function and migration. Two sides of the same coin. N. Engl. J. Med. 343 (14), 1020–1034. doi:10.1056/NEJM200010053431407
Wang, J., Luo, Q., Chen, R., Chen, T., and li, J. (2020). Susceptibility analysis of COVID-19 in smokers based on ACE2. Prepr. Org. 2020, 030078. doi:10.20944/preprints202003.0078.v1
Wang, X., and Liotta, L. (2011). Clinical bioinformatics: a new emerging science. J. Clin. Bioinforma. 1 (1), 1–8. doi:10.1186/2043-9113-1-1
Keywords: SARS-CoV-2, leukocytes, CD19+ lymphocytes, proteomic profiling, COVID-19
Citation: Marcin M, Zahornacký O, Kacírová M, Šuliková M, Bajer-Ďuráčová M, Řehulka P, Bober P, Fedačko J, Jarčuška P and Sabo J (2023) Initial high throughput proteomic analysis reveals alterations in CD19+ B lymphocyte profile in acute COVID-19 patients. Acta Virol. 67:11702. doi: 10.3389/av.2023.11702
Received: 19 June 2023; Accepted: 10 August 2023;
Published: 22 August 2023.
Edited by:
Peter Sabaka, Comenius University, SlovakiaReviewed by:
Juraj Kopacek, Institute of Virology (SAS), SlovakiaPeter Barath, Slovak Academy of Sciences (SAS), Slovakia
Copyright © 2023 Marcin, Zahornacký, Kacírová, Šuliková, Bajer-Ďuráčová, Řehulka, Bober, Fedačko, Jarčuška and Sabo. This is an open-access article distributed under the terms of the Creative Commons Attribution License (CC BY). The use, distribution or reproduction in other forums is permitted, provided the original author(s) and the copyright owner(s) are credited and that the original publication in this journal is cited, in accordance with accepted academic practice. No use, distribution or reproduction is permitted which does not comply with these terms.
*Correspondence: Ján Sabo, jan.sabo@upjs.sk