- 1Molecular Medicine Research Center, Hamadan University of Medical Sciences, Hamadan, Iran
- 2Department of Medical Biotechnology, School of Advanced Technologies in Medicine, Shahid Beheshti University of Medical Science, Tehran, Iran
- 3Core Research Facilities, Isfahan University of Medical Sciences, Isfahan, Iran
- 4Applied Physiology Research Center, Cardiovascular Research Institute, Isfahan University of Medical Sciences, Isfahan, Iran
- 5Infectious Diseases and Tropical Medicine Research Center, Isfahan University of Medical Sciences, Isfahan, Iran
- 6Department of Biotechnology, Faculty of Advanced Sciences and Technology, Tehran Islamic Azad University of Medical Sciences, Tehran, Iran
Frequent, and sometimes more dangerous, mutations in SARS-CoV-2 indicate that a stronger strategy is needed to produce an effective vaccine—a vaccine that contains a wider range of virus factors and remains effective if one or more mutations have occurred in a part of the genome. In this study, four important virus proteins were used to make a multi-epitope protein vaccine. For this purpose, antigenic determinant of 4 proteins were selected and a protein structure was designed using 4 domains containing epitopes. After examining its antigenic potential, its three-dimensional structure was designed and then docked with immune system receptors. Finally, using the dynamic molecular (MD) simulation, complexes and interactions were investigated and their interaction energies were measured. The results of the study showed that the designed structure has good relative stability and interacts well with its receptors and can be used as a vaccine candidate for further studies.
Introduction
COVID-19 continues around the world despite the many efforts that have been made against it. These efforts include developing more diagnostic methods, potential treatments, and the development of various vaccines across different laboratory and clinical stages (Rabie, 2022a; Eltayb et al., 2023). In such epidemics and pandemics, vaccines can be the most effective way to eliminate or reduce the destructive effects of the disease. They are produced at an unprecedented rate, and many of them use new strategies (Giurgea and Memoli, 2020; Haque and Pant, 2020; Kotta et al., 2020; Nokhodian et al., 2020; Mistry et al., 2021). Vaccination strategies become more important when many mutations occur in the virus genome, sometimes leading to a more dangerous and pathogenic virus. Therefore, there is a need to design a type of vaccine that is much more impactful, and in which the mutations of the virus are less detrimental its effectiveness.
There are different types of vaccines, one of which—used against infectious agents—is the production of protein-based or peptide-based vaccines that contain important epitopes of antigens of the infectious agent. These vaccines may have greater advantages over vaccines consisting of larger protein sequences or inactivated viruses. They are smaller and may provoke a more intense immune response than other vaccines because of their stronger immunogenic domains (Malonis et al., 2020; Requena et al., 2020).
The COVID-19 causative virus, severe acute respiratory syndrome coronavirus 2 (SARS-CoV-2), contains several nonstructural proteins (Nsps) and several structural proteins. The virus genome is mainly in the form of two non-structural proteins, ORF1a and ORF1ab, followed by structural proteins. In other words, it has been shown that it encodes 16 non-structural, 4 structural, and 6 accessory proteins. The unstructured proteins include the main protease (Mpro) and papain-like protease (PLpro). Structural proteins include homo-trimeric Spike glycoprotein (S), Nucleocapsid protein (N), and Membrane (M) and Envelope (E) proteins. These structural proteins can play an important role in the development of subunit and protein-based vaccines. The large S protein that emerges from the surface of the membrane acts to bind the virus to the receptor on the membrane of the host cells. This protein is almost completely outside the surface of the virus and can be an important component for the development of subunit-based vaccines. Two other proteins, E and M, have various roles, including regulation of the virion’s assembly and maturation and the budding of new virion particles. These are proteins within the virus envelope, with several transmembrane domains, the epitopes of which can be used in vaccine design (Arya et al., 2020; Bai et al., 2022).
During the pandemic period, various mutations have been reported for different genes of the virus, especially spike proteins, some of which have created a new trend in the disease and have sometimes increased the virulence and lethality of the virus. Some of the most famous variants in spike protein include 28813G>T, 23012G>A, and 23063A>T. Various mutations resulted in several dangerous variants of the virus such as Alpha, Beta, Gamma, Delta, and Omicron, which left devastating effects (Mohammadi et al., 2021). However, some preventive and therapeutic approaches caused their effects to be somewhat curbed. For example, many studies have been carried out on the inhibition or reduction of the virulence of the Omicron variant (Rabie and Abdalla, 2023; Rabie and Eltayb, 2023). Despite all efforts, there is still a need for the production of vaccines that have a high potential to prevent the pathogenicity of all or most of the variants.
The aim of the current in silico study is to determine the important epitopes of three structural proteins, E, M, and S, and a nonstructural protein, Mpro, of the virus using online tools and databases, and to construct a multi-epitope and evaluate the immunogenic potential of this putative protein with bioinformatic software as a vaccine candidate against COVID-19.
Methods
Retrieval of sequences and structures of the proteins
The amino acid sequences of four proteins, S (NCBI Reference Sequence: YP_009724390.1), E (NCBI Reference Sequence: YP_009724392.1), M (GenBank: QIC53216.1), and Mpro (NCBI Reference Sequence: YP_009742612.1), were retrieved from Protein, National Center for Biotechnology Information (NCBI) database1. Also, the structures of two proteins, S (PDB ID: 6VYB) and Mpro (PDB ID: 6Y2F), were obtained from Protein Data Bank (PDB) database2 (Berman et al., 2000). The structures of two other proteins, E and M, were modelled as reported in our previous article (Alibakhshi et al., 2020).
Preparation and analysis of proteins
The physicochemical properties of the proteins were evaluated using the ProtParam online server (Wilkins et al., 1999). The transmembrane protein topologies of three proteins E, M, and S were analyzed using UniProt database(UniProt Consortium, 2019) (Entry identifiers: P0DTC2, P0DTC5, and P0DTC4 for S, M, and E proteins, respectively). Also, using TMHMM Server v. 2.0, transmembrane helices in proteins were predicted with a hidden Markov model (Krogh et al., 2001). The structures of proteins were prepared using UCSF Chimera Version 1.8.1 by removing the ligand and water molecules (Pettersen et al., 2004).
CTL, HTL, and linear epitope prediction (prediction of T-cell (HLA class I and II) epitopes and B-cell epitopes (linear))
In the first step, the online prediction server Immune Epitope Database (IEDB)3 was used to predict the T-cell epitopes of each determinant protein (Nielsen et al., 2003). MHCpred (Guan et al., 2003)4 and ProPred servers (Singh and Raghava, 2001)5 were also used to predict the MHC restricted T-cell epitopes.
Moreover, the peptide binders to MHCI and MHCII molecules from protein sequences were predicted using the RANKPEP server (Reche et al., 2004)6.
The ABCpred (Saha and Raghava, 2006)7, BCpreds (El-Manzalawy et al., 2008)8, and BepiPred servers (Jespersen et al., 2017)9 were used to predict the linear B-cell epitopes. The Ellipro server (Ponomarenko et al., 2008)10 was also used to identify continuous epitopes in the viral proteins.
In addition, for further evaluation and comparison of the scores of selected epitopes, the SVMTriP server (Yao et al., 2012)11 was used to predict the antigenic epitopes based on sequence input. This server employs a Support Vector Machine (SVM) approach which combines the tri-peptide similarity and propensity scores (SVMTriP) in order to achieve higher accuracy and specificity by the leave-one-out test.
Multi-epitope vaccine construction and analysis
After selecting the epitopes, three domains from the S protein, two domains from the M protein, two domains from the Mpro protein, and one domain from the E protein were selected and, using these domains and conventional linkers, the amino acid structure was constructed. The selection of protein domains was based on the score of epitopes and their location on the outer region of the envelope proteins.
The selected domains were linked by two conventional linkers, AAY and GPGPG. These linkers help to enhance epitope presentation as well as protein flexibility and folding, and stimulate immune responses (Nezafat et al., 2014). Finally, an 1820 amino acid multi-epitope protein was constructed. The sequence of putative multi-epitope construct was submitted to ProtParam online server (Wilkins et al., 1999) for evaluation of physicochemical parameters.
Antigenicity and allergenicity evaluation
The antigenicity of the suggested construct was predicted by the VaxiJen v2.0 server (Doytchinova and Flower, 2007). This server predicts the antigenicity potential using an alignment-independent method and physicochemical properties of proteins without the aid of sequence alignment. We also examined the potential allergenicity of the construct by the AllerTOP v2.0 server (Dimitrov et al., 2013), which predicts the allergenicity based on the main physicochemical properties of proteins.
Protein modelling, tertiary structure refinement, and validation
The multi-epitope sequence was submitted to two protein structure prediction servers, the Robetta server and I-TASSER server. While Robetta employs a relatively fast and accurate deep learning-based method—TrRosetta (Yang et al., 2020)—to predict protein 3D structure, I-TASSER uses a hierarchical approach to protein folding prediction and structure-based function annotation. This identifies structural templates by a multiple threading approach with atomic models which are constructed by template-based fragment simulations (Yang and Zhang, 2015). Each of these servers generated five models. All the models were then submitted to the 3Drefine server (Bhattacharya et al., 2016) for model refinement. 3Drefine uses iterative optimization of a hydrogen bonding network combined with atomic-level energy minimization for consistent and computationally efficient protein structure refinement. The protein models were then analyzed by ProSA (Wiederstein and Sippl, 2007), PROCHECK (Laskowski et al., 1993), and ERRAT (Colovos and Yeates, 1993) programs for model qualification and validation. The ProSA displays a Z-score indicating overall model quality for measuring the deviation of the structure’s total energy. A value outside the range characteristic for native proteins points to a potentially erroneous structure. On the other hand, PROCHECK program examines the stereochemical quality of a protein structure by analyzing both overall and residue-by-residue geometry. The ERRAT analyzes non-bonded atomic interaction and displays a plot with an overall quality factor in which, in the higher values, the model is better.
Molecular dynamic (MD) simulation of the multi-epitope construct
The best 3D model of the construct as determined by our qualification/validation study was then subjected to molecular dynamic (MD) simulation for more optimization and refinement of the 3D model of the multi-epitope protein. MD simulation was carried out by GROMACS software (Abraham et al., 2015) v. 5.1.1 using GROMOS96 54a7 force field (Schmid et al., 2011). The MD simulation was set up by placing the construct in a cube box. The box was then filled with water (spc216), and the system was neutralized with Na+ ions. The solvated and electroneutral system was energy minimized. Before dynamics, the system was equilibrated in two phases under temperature ensemble (NVT) and pressure ensemble (NPT) for 100 ps, and finally, MD simulation was performed for 30 ns. The trajectory was then analyzed by Root Mean Square Fluctuation (RMSF), Mean Square Deviation (RMSD) and Radius of gyration (Rg) to characterize the dynamics of the multi-epitope construct.
Analysis of spatial epitopes of the multi-epitope construct
The 3D structures of the multi-epitope protein and four proteins used for the construction of the multi-epitope were submitted to SEPPA3.0 server (Zhou et al., 2019) to analyze the structural epitopes. This server uses a prediction algorithm based on surface residues and glycosylation properties of antigens to predict the spatial and conformational epitopes.
Preparing the structures of immune receptors, molecular docking, and refinement
The crystallographic structures that most commonly occur in HLA Class I and Class II subtypes in the human population, i.e., 02:01 (PDB ID: 4U6Y) and DRB1 01:01 (PDB ID: 1AQD) as well as the 3D structure of human Toll-like Receptor 3 (TLR3)—which plays an essential role in the innate immune system (PDB ID: 1ZIW)—were obtained from the PDB database2. The structures were prepared for protein-protein docking by UCSF Chimera software. Also, the last frame of the trajectory of the simulation was used as the input structures for protein-protein molecular docking with immune receptors. PatchDock server (Schneidman-Duhovny et al., 2005) with a molecular docking algorithm based on shape complementarity principles was employed for structure prediction of protein-protein. The server generates several docked complexes based on favorable interactions. The ten solutions with the best positions and orientations were selected for interaction refinement by FireDock server (Mashiach et al., 2008), an efficient method for the refinement and re-scoring of rigid-body protein-protein docking solutions. Finally, the best complexes were selected for MD simulation based on the server’s global energy.
MD simulation of docked complexes
For a detailed examination of selected protein complexes, we performed a 10-ns MD simulation for each complex according to the described method. Besides the mentioned analysis for multi-epitope MD simulation, in this step, minimum distance (nm), number of contacts (<0.6), the sum of electrostatic and van der Waals energies (kJmol-1), and number of the hydrogen bond between multi-epitope and receptor, were calculated.
MM/PBSA binding free energy
The absolute binding affinities of receptor/multi-epitope complexes were calculated using molecular mechanics (MM) energies combined with Poisson–Boltzmann (PB) and Surface Area (SA) solvation methods (MM/PBSA) (Genheden and Ryde, 2015) and g_mmpbsa package (Kumari et al., 2014). In the MM/PBSA method that was developed by Kollman et al. (2000), ΔG bind is estimated from the free energies of the two reactants and the final complex:
Results
Analysis of the protein sequence and structure
Table 1 shows the overall characteristics of the viral protein targets. The predicted characteristics of the proteins confirm their appropriateness for antigen discovery. Here, in selecting the final epitopes, an attempt was made to use more exposed areas. Some of the other primary features predicted for proteins that influence epitope selection are shown in Figure 1 and Table 2.
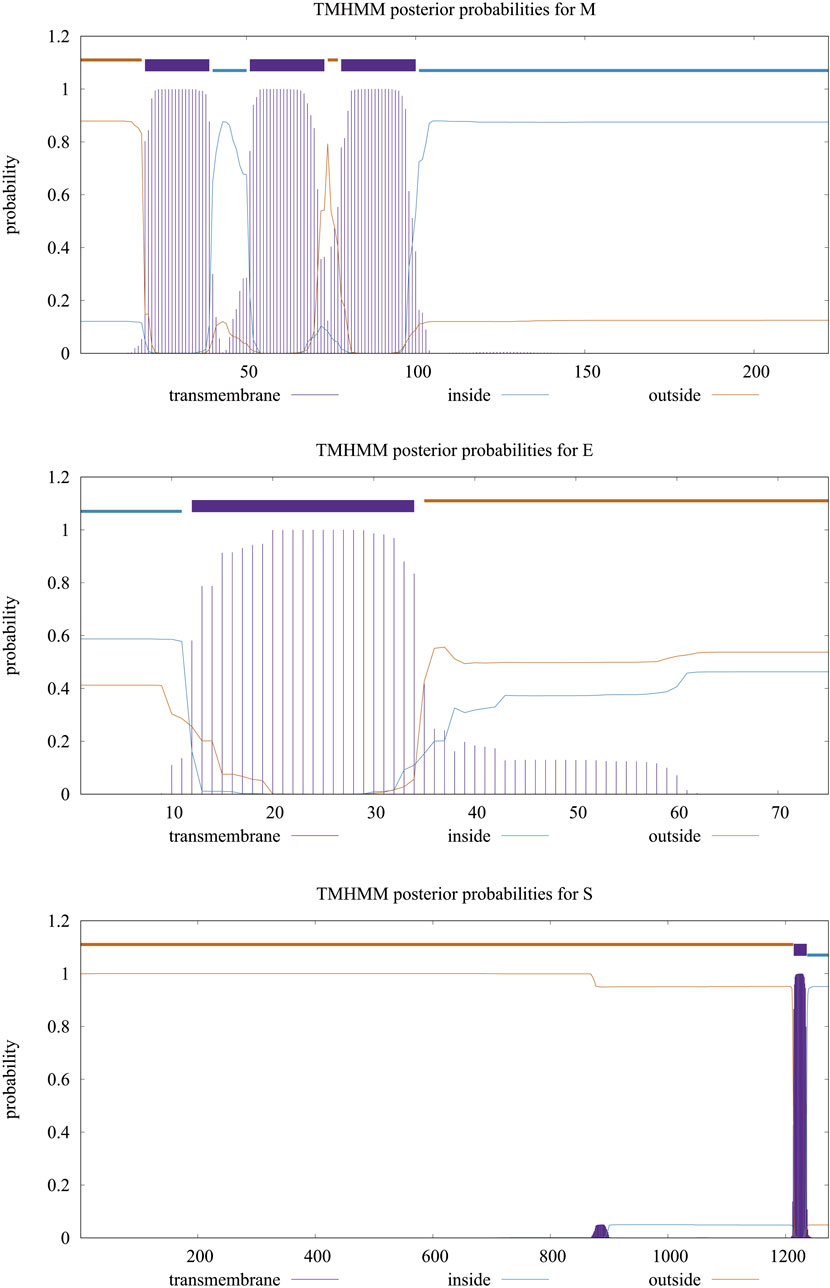
FIGURE 1. Transmembrane topology of proteins. The purple line shows the sequences outside the envelope.
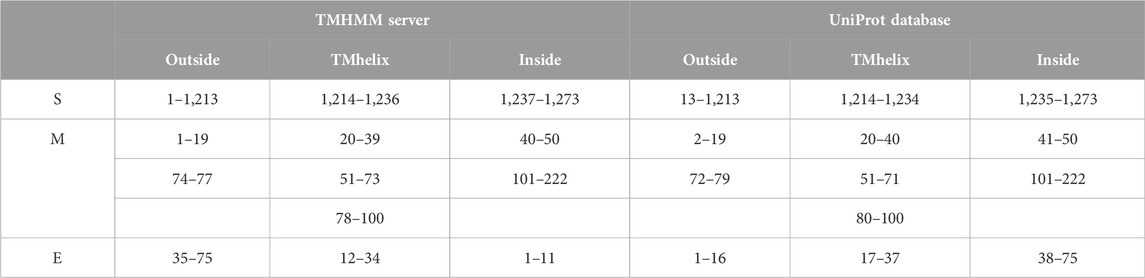
TABLE 2. Location and the topology of the virus’s proteins, predicted by TMHMM server and studied by UniProt database. As it is shown in this table and Figure 1, the significant parts of all three proteins are located in the outer space of the viral membrane, which causes more epitopes to be exposed to immune system factors.
Epitope prediction and designing of the multi-epitope protein
The epitopes with the best scores in stimulating immune system components for all four proteins are listed in Table 3. Then, with further investigation, domains of each of the proteins, which were most likely to contain the epitopes, were preferred (Table 4), and these domains were used to construct and design the final multi-epitope using linkers.
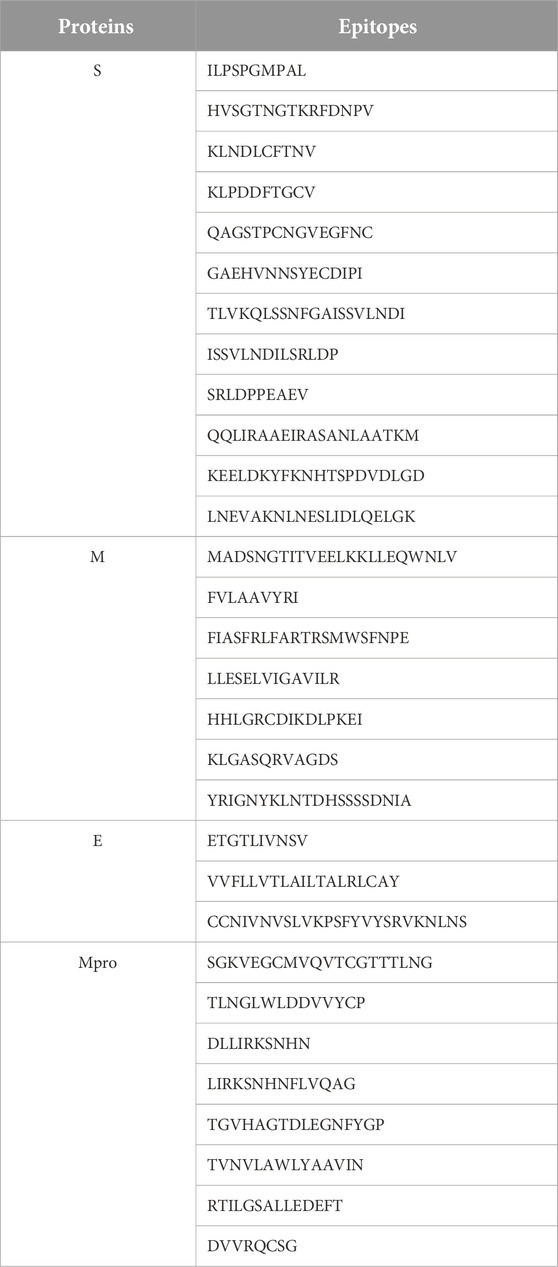
TABLE 3. Predicted epitopes of the proteins. For protein S, more epitopes are considered due to its large size.
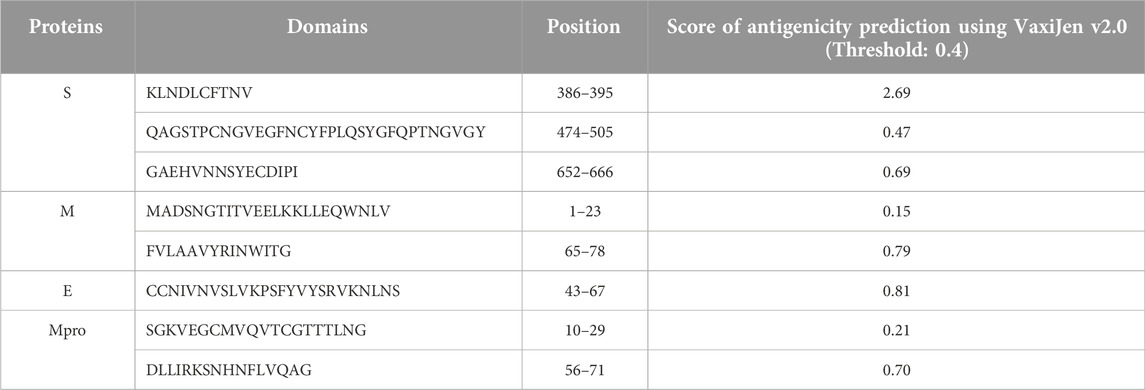
TABLE 4. Epitope-rich domains of the proteins deployed for the construction of a putative multi-epitope protein. The final structure was designed and built using these domains and in the order mentioned in the table.
Antigenicity and allergenicity evaluation
VaxiJen v2.0 server predicted an overall antigenicity score of 0.5579. The score falls above the threshold intended for viruses (0.4), conforming as a probable antigen. In addition to being antigenic, the designed protein should not induce—or induce only minimal—allergenicity in the host body, by provoking an IgE antibody response. The AllerTOP v. 2.0 server was therefore used to predict the allergenicity of the designed multi-epitope protein, and the result identified the construct as a probable non-allergen.
Multi-epitope construct modelling and validation
Using two well-known protein structure prediction servers (I-TASSER and Robetta), the multi-epitope structure was modeled, and the obtained structures were then refined and validated using a range of model qualification tools. Almost all the structures predicted by the Robatta server (TrRosetta method) showed to be higher quality as compared with those predicted by the I-TASSER server (Figure 2A). More specifically, the best model obtained from I-TASSER showed 58.2% of residues in the most favored regions, 38.3% in the allowed regions and 3.4% in the disallowed region of the Ramachandran plot, whereas the corresponding values for the Robetta-obtained model were 71.2%, 27.4%, and 1.4% respectively. In addition, while ERRAT showed an overall quality factor of 66.66 and ProSA gave a Z-Score of −3.24 for the best I-TASSER-obtained model, the best Robettal-obtained model was characterized by the corresponding values of 82.75 and −5.39 (Figures 2B–D). Therefore, the best 3D structure predicted by Robetta was considered for the next steps of the study.
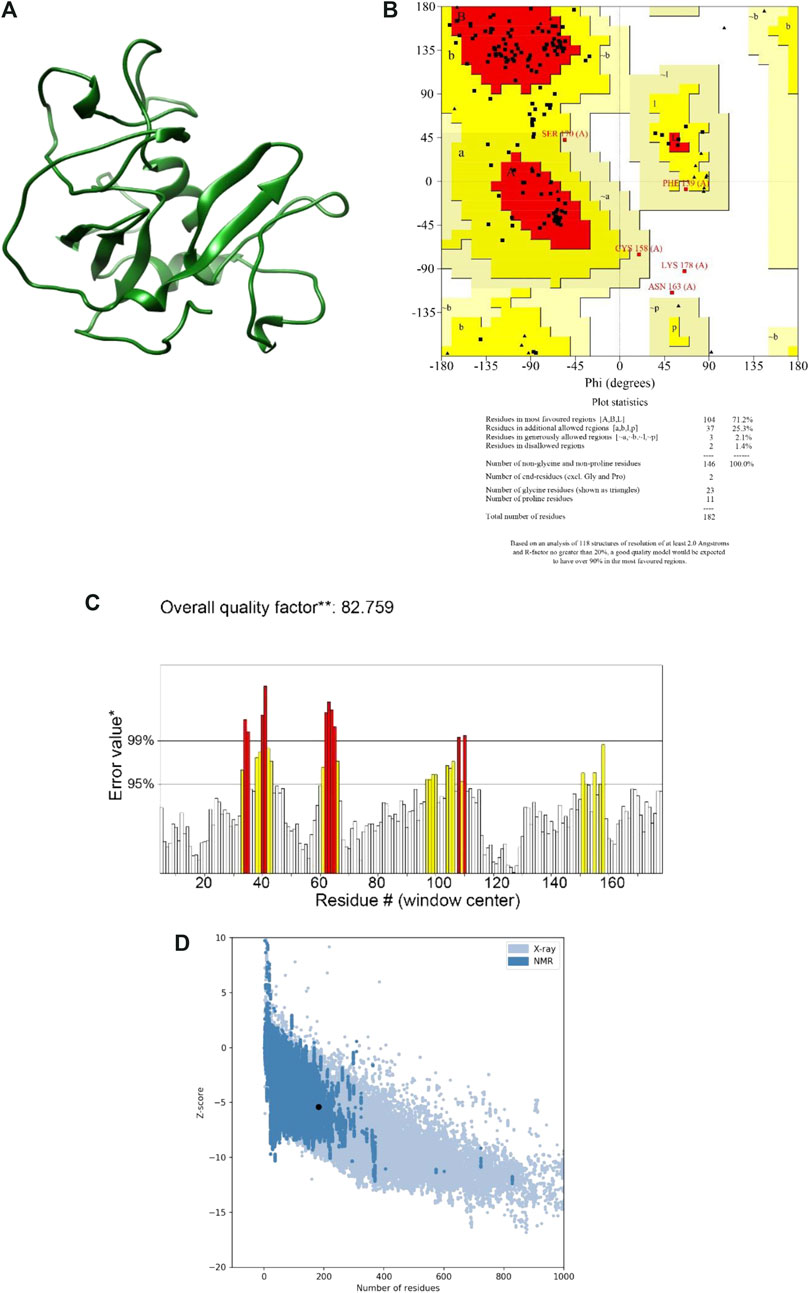
FIGURE 2. The final model and the results of the validation. (A) Final model structure. (B) Ramachandran plot. It is shown in the graph that a total of 98.6% of the residues are in favored and allowed areas. (C) ERRAT plot. This plot shows that error values for residues had an overall quality factor of 82.7% for residues of the multi-epitope protein. (D) ProSA-web Z-score. This plot indicates overall model quality. The protein construct indicated a Z-score of −3.24 that is within the Z-scores range of experimentally determined protein chains of a similar size.
MD simulation of the multi-epitope construct
The MD simulation was used to verify the 3D structure of the multi-epitope protein from an atomic-resolution model construction. The structure was relaxed through the energy minimization process, demonstrating a steady convergence of potential energy (Figure 3A). The system was also well-equilibrated at a temperature of 300 K and under pressure until it reached a proper density. Following the MD simulation for 30 ns, the dynamics of the construct were characterized by calculating RMSD, RMSF, and Rg across the trajectory (Figure 3B). While the evolution of RMSD and Rg show the time-dependent stability of the protein structure, RMSF accounts for the structural adaptability of Cα atoms of every residue (Ahmad et al., 2020). RMSD for the final 10 ns was 0.447 ± 0.011, and Rg for this time showed a value equal to 1.561 ± 0.007. The result analysis showed an RMSF of 0.181 ± 0.082. These results showed that the protein was well-stabilized. The structure of the multi-epitope after MD simulation is shown in Figure 3C.
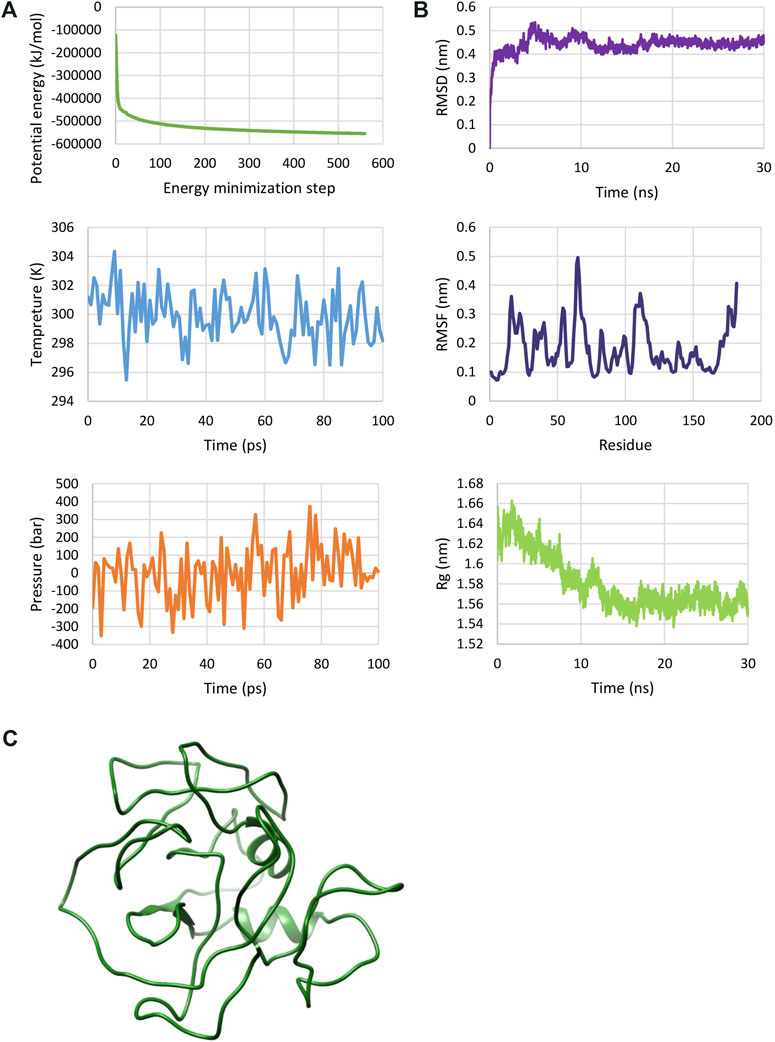
FIGURE 3. MD simulation of the multi-epitope vaccine. (A) The plotted results after energy minimization and equilibration steps. These plots show a protein construct energy minimized and equilibrated. (B) RMSD, RMSF and Rg plots determined by molecular dynamics (MD) simulation. These plots show that the protein has a relatively stable structure. (C) 3D structure of multi-epitope after MD simulation.
Analysis of conformational epitopes
The structural epitopes play a critical role in stimulating the immune system. In this study, after designing a multi-epitope vaccine and before docking with immune system receptors, all the structural epitopes predicted by the SEPPA server were assessed and compared with all four primer proteins, and it was found that most of the spatial epitopes in the final model have similar structures (Figure 4).
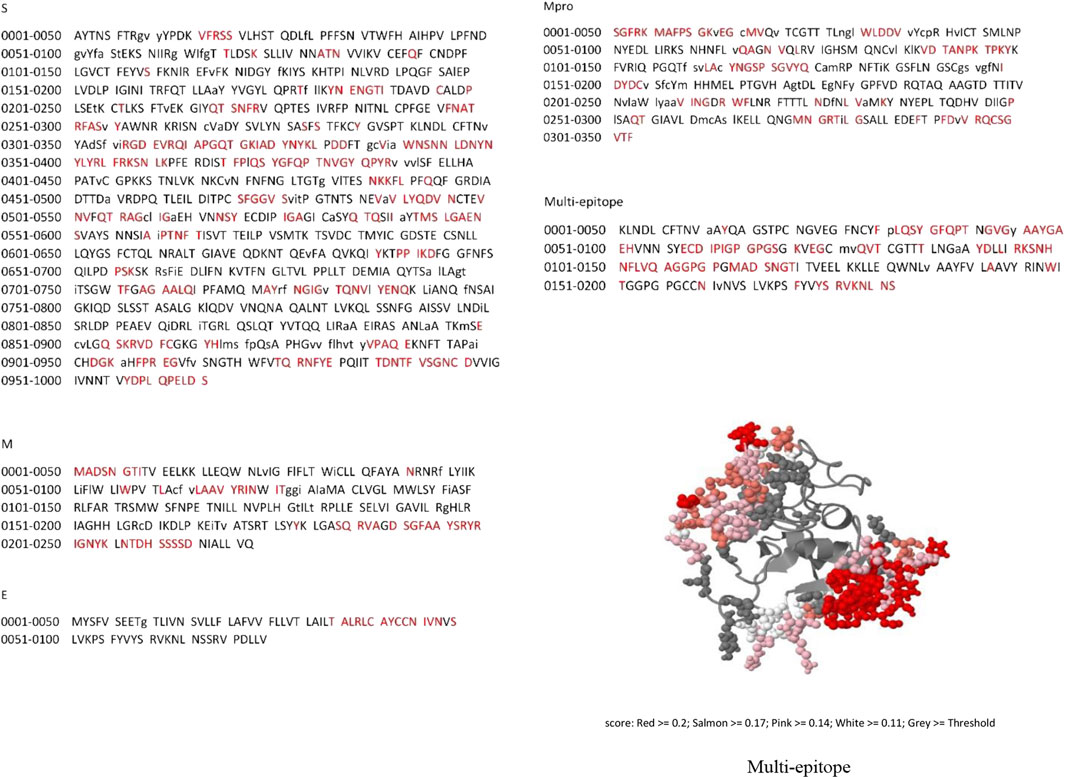
FIGURE 4. The spatial epitope prediction of the four proteins and final multi-epitope by SEPPA 3.0 server. Red residues are spatial epitopes.
Protein-protein docking and refinement
The protein-protein docking between the multi-epitope and three receptors in the immune system was conducted in different modes with the help of two servers, PatchDock and FireDock, and the top 10 solutions were assessed based on the global binding energy (Table 5). This ranking is according to several binding energies, including desolvation energy (atomic contact energy, ACE), van der Waals interactions, partial electrostatics, hydrogen and disulfide bonds, aliphatic interactions, and others. In this regard, solution 9, solution 5, and solution 2 for DRB1 0101/Multi-epitope, HLA-A0201/Multi-epitope, and TLR3/Multi-epitope complexes, respectively, were selected for further evaluation and calculations due to their superior binding energy.
MD simulation of docked complexes
With regards to the stable structure of the multi-epitope and receptors, we performed a 10-ns MD simulation of their complex. RMSD analysis of the trajectory shows that the complex reaches a stable state after four nano-seconds (Figure 5A). Rg, RMSD, and RMSF analysis of the multi-epitope construct in each complex, in addition to the number of the hydrogen bonds between the multi-epitope and each receptor, showed that the multi-epitope construct has a stable state during the interaction with each receptor (Figure 5; Table 6). The average values of the minimum distance (nm), the number of contacts, and the sum of electrostatic and van der Waals energies (kJmol−1) between the vaccine construct and each receptor, show a consistent interaction between them (Table 6). Figure 6 shows a schematic representation of complexes after MD simulation in which the interaction of the multi-epitope vaccine candidate with three immune system receptors, DRB1 0101, HLA-A0201, and TLR3, has been shown.
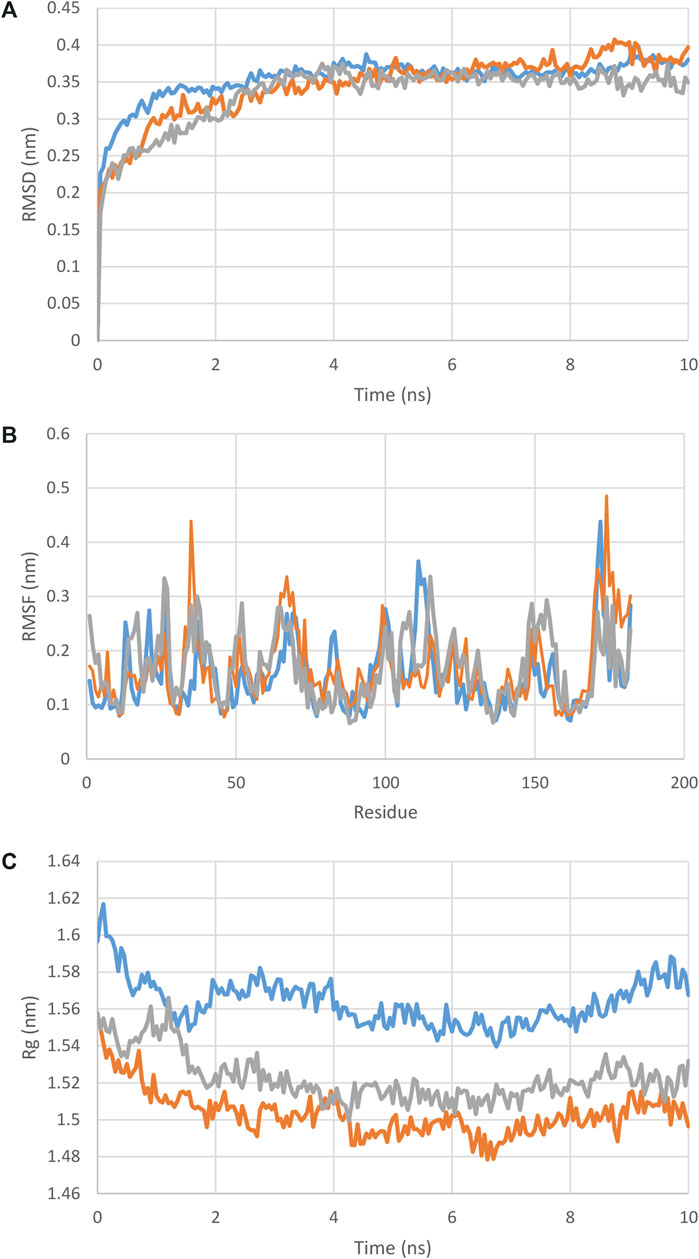
FIGURE 5. (A) : RMSD, (B) : RMSF, (C) : Rg plot of the multi-epitope in complex with each receptor. As it is clear from these graphs and the RMSD and Rg values from Table 5, the docked complexes reached relative stability very quickly and did not show any specific deviation in the structures.
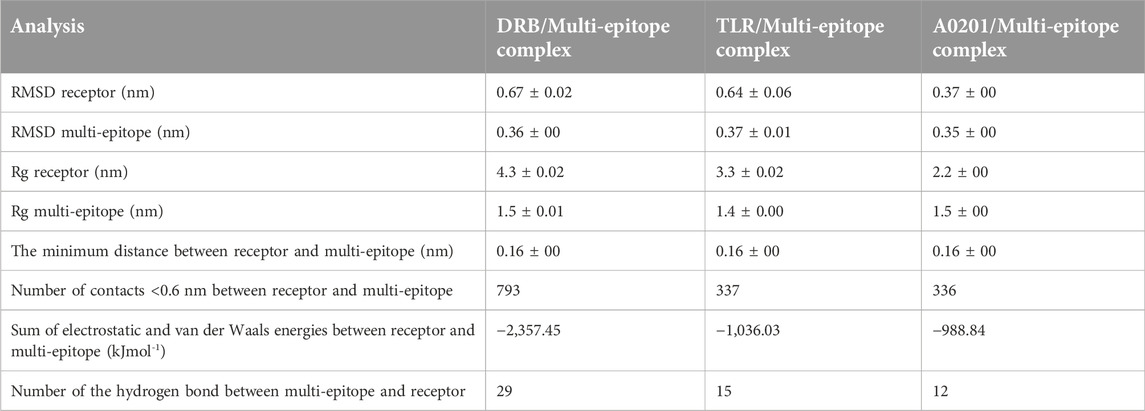
TABLE 6. The averages of the MD analysis parameters for complexes of multi-epitope and DRB, TLR and A0201.
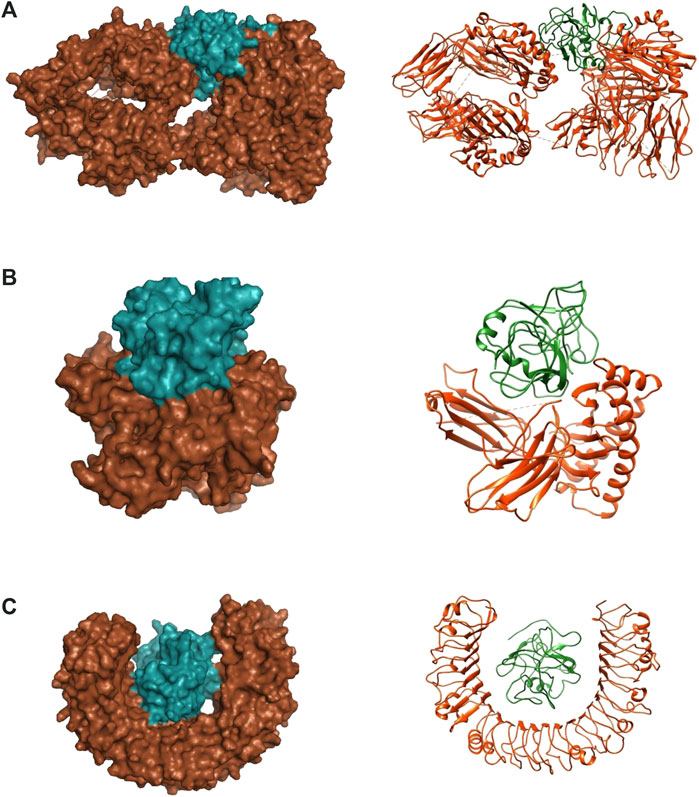
FIGURE 6. Docked complexes between multi-epitope vaccine candidates and three immune system receptors. (A) DRB1 0101/Multi-epitope. (B) HLA-A0201/Multi-epitope. (C) TLR3/Multi-epitope. Brown color: receptor, Green color: Multi-epitope.
MM/PBSA binding free energy
Table 7 shows the calculated binding energies between immune receptors and the multi-epitope construct, including van der Waals, Electrostatic, Polar solvation, SASA, and total binding energies. In total, the calculations indicated high interaction energies. The binding energy in the A0201/Multi-epitope and TLR/Multi-epitope showed strong interactions, but in the DRB/Multi-epitope, although sufficient, it is not as strong as the other two complexes.
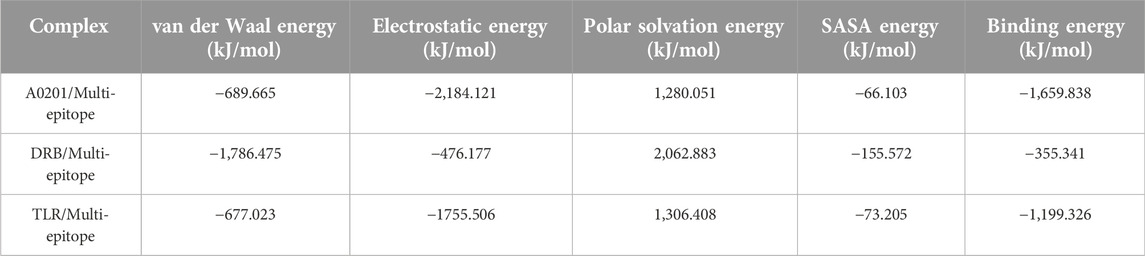
TABLE 7. The binding energies and total energy are calculated by the MM/PBSA method. The calculation of free binding energies between two molecules of the complexes show a high affinity and interaction. The high affinity is especially remarkable for the two complexes A0201/Multi-epitope and TLR/Multi-epitope.
Discussion
The development of vaccines is one of the most critical achievements in medicine, through which many infectious diseases could be eradicated. Recently, multi-epitope vaccines based on epitope selection have been increasingly used and improved. New types of vaccines with high immunogenicity and low risks, including epitope-based vaccines, are being used. Continued spread of COVID-19, an infectious disease caused by SARS-CoV-2, has killed more than millions of people worldwide and has harmed social, financial, and political institutions around the world. With no effective drug treatment available, vaccines are essential to prevent the spread of the disease.
In this in silico study of vaccine design, we tried to use various antigens. The low values of the instability index marked all four proteins as stable proteins in the test tube. The aliphatic index is an indicator of the relative volume occupied by some nonpolar amino acids and the stability of the proteins against temperature variation, by which the higher the value, the more stable the protein. Grand Average Hydropathicity (GRAVY) shows the level of protein hydrophilicity, where the lower value indicates greater hydrophilicity. Figure 1 and Table 2 present the information on the location and the topology of the proteins (S, E, and M) in the virus envelope. Generally, the protein areas located outside the virion and envelope are considered to have the strongest potential to stimulate the immune system. The predicted characteristics of the proteins confirm their appropriateness for antigen discovery. Here, in selecting the final epitopes, an attempt was made to use more exposed areas. Epitope prediction results obtained from several epitope databases and servers were analyzed, and epitopes with the best score in stimulating immune system components were selected that could be used as domains with more epitopes to construct and design a putative multi-epitope in the order mentioned in the Table 4 using the linkers.
After confirming the antigenicity of the structure and its non-allergenic nature, the suggested construct was modeled and validated. Also, the results of MD simulation showed that the protein was well-stabilized. The structure was docked with three immune receptors to show its relative strength in stimulating the immune system. Three solutions for DRB1 0101/Multi-epitope, HLA-A0201/Multi-epitope, and TLR3/Multi-epitope complexes, respectively, were selected due to better binding energy for further evaluation and calculations. The results of MD simulation of the docked complexes showed that a consistent interaction and the binding energy analysis revealed that in the A0201/Multi-epitope and TLR/Multi-epitope, strong interactions were formed, but in the DRB/Multi-epitope, although sufficient, it is not as strong as the other two complexes.
In recent years, various studies have been conducted to deal with this pandemic. These studies have included methods for prevention and the design of various new vaccines, as well as attempts to design and discover potential drugs. In this way, in silico methods have made a significant contribution, because methods with fast delivery of results are needed to control such pandemics. In the last 3 years, many studies have been conducted to find effective molecules to inhibit the activity of virus protein factors, or the stages of virus production and assembly, or the stages of disease progression. These effective molecules include FDA approved drugs or plant molecules, among others. Some of these molecules have shown promising results after being tested in laboratory and animal models (Rabie, 2021a; Rabie, 2021b; Rabie, 2021c; Rabie, 2022b; Rabie and Abdalla, 2022; Alibakhshi et al., 2023; Sharma et al., 2023; Tong et al., 2023). Also, toward the goal of prevention and vaccine design, very diverse and sometimes valuable works have been carried out, some of which lead to the production of vaccines that have entered the market. These vaccines include live attenuated vaccines, molecular components of the virus, DNA vaccines, and mRNA vaccines.
During the pandemic, various vaccines have been designed, developed, and tested (Hadj Hassine, 2022). Multi-epitope vaccines can be used more often because they are safe, stable, easy to produce, and can stimulate both humoral and cellular immune system responses (Forouharmehr et al., 2022). The development of multi-epitope vaccines has made up a significant portion of in silico and laboratory studies on SARS-CoV-2. In these studies, different genes and virus variants have been used, and interesting results have been obtained in almost all of them (Jyotisha et al., 2022; Khairkhah et al., 2022; Parmar et al., 2022). The results of our work have been in line with studies in this field and show similar outcomes. The difference in this work is the use of domains that each have several epitopes. All in all, more investigations and more fundamental changes, as well as the use of laboratory tests, can show that these types of vaccines should be given more attention.
In conclusion, in this study, after designing a putative multi-epitope and determining its 3D structure using dynamic molecular simulation, it was found that the model has a stabile biomolecular structure. In addition, with the help of molecular docking, molecular dynamics methods and interaction energies were shown that suggested the structure can stimulate the immune system. Therefore, according to the confirmed immunogenicity of this structure, it can be confirmed that the in silico designed structure has good relative stability and interacts well with its receptors, making it a potentially viable a vaccine candidate and worth further evaluation using laboratory methods.
Data availability statement
The original contributions presented in the study are included in the article/supplementary material, further inquiries can be directed to the corresponding author.
Author contributions
AA: carried out the experiments. AAB: carried out the experiments. EM: carried out the experiments. SA: wrote the manuscript. MM: helped supervise the project. All authors contributed to the article and approved the submitted version.
Conflict of interest
The authors declare that the research was conducted in the absence of any commercial or financial relationships that could be construed as a potential conflict of interest.
Footnotes
1https://www.ncbi.nlm.nih.gov/protein
4http://www.ddg-pharmfac.net/mhcpred/MHCPred/
5http://crdd.osdd.net/raghava/propred/
6http://imed.med.ucm.es/Tools/rankpep.html
7http://crdd.osdd.net/raghava/abcpred/
8http://ailab.ist.psu.edu/bcpred/
9http://www.cbs.dtu.dk/services/BepiPred/
10http://tools.iedb.org/ellipro/
11http://sysbio.unl.edu/SVMTriP/prediction.php
References
Abraham, M. J., Murtola, T., Schulz, R., Páll, S., Smith, J. C., Hess, B., et al. (2015). Gromacs: high performance molecular simulations through multi-level parallelism from laptops to supercomputers. SoftwareX 1-2, 19–25. doi:10.1016/j.softx.2015.06.001
Ahmad, S. S., Sinha, M., Ahmad, K., Khalid, M., and Choi, I. (2020). Study of caspase 8 inhibition for the management of alzheimer’s disease: a molecular docking and dynamics simulation. A Mol. Docking Dyn. Simul. 25 (9), 2071. doi:10.3390/molecules25092071
Alibakhshi, A., Gharibi, S., Ahangarzadeh, S., and Yarian, F. (2023). Bioinformatic screening of compounds from Iranian lamiaceae family members against SARS-CoV-2 spike protein. Lett. Drug Des. Discov. 20 (6), 684–698. doi:10.2174/1570180819666220509090514
Alibakhshi, A., Ranjbar, M. M., Javanmard, S. H., Yarian, F., and Ahangarzadeh, S. (2020). Virtual screening for the identification of potential candidate molecules against envelope (E) and membrane (M) proteins of SARS-CoV-2. J. Comput. Biophysics Chem. 20, 209–224. doi:10.1142/s2737416521500083
Arya, R., Kumari, S., Pandey, B., Mistry, H., Bihani, S. C., Das, A., et al. (2020). Structural insights into SARS-CoV-2 proteins. J. Mol. Biol. 433 (2), 166725. doi:10.1016/j.jmb.2020.11.024
Bai, C., Zhong, Q., and Gao, G. F. (2022). Overview of SARS-CoV-2 genome-encoded proteins. Sci. China Life Sci. 65 (2), 280–294. doi:10.1007/s11427-021-1964-4
Berman, H. M., Westbrook, J., Feng, Z., Gilliland, G., Bhat, T. N., Weissig, H., et al. (2000). The protein Data Bank. Nucleic acids Res. 28 (1), 235–242. doi:10.1093/nar/28.1.235
Bhattacharya, D., Nowotny, J., Cao, R., and Cheng, J. (2016). 3Drefine: An interactive web server for efficient protein structure refinement. Nucleic acids Res. 44 (W1), W406–W409. doi:10.1093/nar/gkw336
Colovos, C., and Yeates, T. O. (1993). Verification of protein structures: Patterns of nonbonded atomic interactions. Protein Sci. 2 (9), 1511–1519. doi:10.1002/pro.5560020916
Dimitrov, I., Flower, D. R., and Doytchinova, I. (2013). AllerTOP--a server for in silico prediction of allergens. BMC Bioinforma. 14 (6), S4. doi:10.1186/1471-2105-14-s6-s4
Doytchinova, I. A., and Flower, D. R. (2007). VaxiJen: A server for prediction of protective antigens, tumour antigens and subunit vaccines. BMC Bioinforma. 8, 4. doi:10.1186/1471-2105-8-4
El-Manzalawy, Y., Dobbs, D., and Honavar, V. (2008). Predicting linear B-cell epitopes using string kernels. J. Mol. Recognit. 21 (4), 243–255. doi:10.1002/jmr.893
Eltayb, W. A., Abdalla, M., and Rabie, A. M. (2023). Novel investigational anti-SARS-CoV-2 agent ensitrelvir “S-217622”: a very promising potential universal broad-spectrum antiviral at the therapeutic frontline of coronavirus species. ACS Omega 8 (6), 5234–5246. doi:10.1021/acsomega.2c03881
Forouharmehr, A., Banan, A., Mousavi, S. M., and Jaydari, A. (2022). Development of a novel multi-epitope vaccine candidate against Streptococcus iniae infection in fish: an immunoinformatics study. Archives Razi Inst. 77 (1), 45–56. doi:10.22092/ARI.2021.353377.1601
Genheden, S., and Ryde, U. (2015). The MM/PBSA and MM/GBSA methods to estimate ligand-binding affinities. Expert Opin. drug Discov. 10 (5), 449–461. doi:10.1517/17460441.2015.1032936
Giurgea, L. T., and Memoli, M. J. (2020). Navigating the quagmire: comparison and interpretation of COVID-19 vaccine phase 1/2 clinical trials. Vaccines 8 (4), 746. doi:10.3390/vaccines8040746
Guan, P., Doytchinova, I. A., Zygouri, C., and Flower, D. R. (2003). MHCPred: a server for quantitative prediction of peptide-MHC binding. Nucleic acids Res. 31 (13), 3621–3624. doi:10.1093/nar/gkg510
Hadj Hassine, I. (2022). COVID-19 vaccines and variants of concern: a review. Rev. Med. virology 32 (4), e2313. doi:10.1002/rmv.2313
Haque, A., and Pant, A. B. (2020). Efforts at COVID-19 vaccine development: challenges and successes. Vaccines 8 (4), 739. doi:10.3390/vaccines8040739
Jespersen, M. C., Peters, B., Nielsen, M., and Marcatili, P. (2017). BepiPred-2.0: Improving sequence-based B-cell epitope prediction using conformational epitopes. Nucleic acids Res. 45 (W1), W24–W29. doi:10.1093/nar/gkx346
Jyotisha, , Singh, S., and Qureshi, I. A. (2022). Multi-epitope vaccine against SARS-CoV-2 applying immunoinformatics and molecular dynamics simulation approaches. J. Biomol. Struct. Dyn. 40 (7), 2917–2933. doi:10.1080/07391102.2020.1844060
Khairkhah, N., Bolhassani, A., Agi, E., Namvar, A., and Nikyar, A. (2022). Immunological investigation of a multiepitope peptide vaccine candidate based on main proteins of SARS-CoV-2 pathogen. PloS one 17 (6), e0268251. doi:10.1371/journal.pone.0268251
Kollman, P. A., Massova, I., Reyes, C., Kuhn, B., Huo, S., Chong, L., et al. (2000). Calculating structures and free energies of complex molecules: Combining molecular mechanics and continuum models. Accounts Chem. Res. 33 (12), 889–897. doi:10.1021/ar000033j
Kotta, S., Aldawsari, H. M., Badr-Eldin, S. M., Alhakamy, N. A., Md, S., Nair, A. B., et al. (2020). Combating the pandemic COVID-19: clinical trials, therapies and perspectives. Front. Mol. Biosci. 7, 606393. doi:10.3389/fmolb.2020.606393
Krogh, A., Larsson, B., von Heijne, G., and Sonnhammer, E. L. (2001). Predicting transmembrane protein topology with a hidden markov model: Application to complete genomes. J. Mol. Biol. 305 (3), 567–580. doi:10.1006/jmbi.2000.4315
Kumari, R., Kumar, R., and Lynn, A. (2014). g_mmpbsa--a GROMACS tool for high-throughput MM-PBSA calculations. J. Chem. Inf. Model. 54 (7), 1951–1962. doi:10.1021/ci500020m
Laskowski, R. A., MacArthur, M. W., Moss, D. S., and Thornton, J. M. (1993). Procheck: A program to check the stereochemical quality of protein structures. J. Appl. Crystallogr. 26 (2), 283–291. doi:10.1107/s0021889892009944
Malonis, R. J., Lai, J. R., and Vergnolle, O. (2020). Peptide-based vaccines: current progress and future challenges. Curr. Prog. Future Challenges 120 (6), 3210–3229. doi:10.1021/acs.chemrev.9b00472
Mashiach, E., Schneidman-Duhovny, D., Andrusier, N., Nussinov, R., and Wolfson, H. J. (2008). FireDock: A web server for fast interaction refinement in molecular docking. Nucleic acids Res. 36 (Web Server issue), W229–W232. doi:10.1093/nar/gkn186
Mistry, P., Barmania, F., Mellet, J., Peta, K., Strydom, A., Viljoen, I. M., et al. (2021). SARS-CoV-2 variants, vaccines, and host immunity. Front. Immunol. 12, 809244. doi:10.3389/fimmu.2021.809244
Mohammadi, E., Shafiee, F., Shahzamani, K., Ranjbar, M. M., Alibakhshi, A., Ahangarzadeh, S., et al. (2021). Novel and emerging mutations of SARS-CoV-2: Biomedical implications. Biomed. Pharmacother. 139, 111599. doi:10.1016/j.biopha.2021.111599
Nezafat, N., Ghasemi, Y., Javadi, G., Khoshnoud, M. J., and Omidinia, E. (2014). A novel multi-epitope peptide vaccine against cancer: An in silico approach. J. Theor. Biol. 349, 121–134. doi:10.1016/j.jtbi.2014.01.018
Nielsen, M., Lundegaard, C., Worning, P., Lauemøller, S. L., Lamberth, K., Buus, S., et al. (2003). Reliable prediction of T-cell epitopes using neural networks with novel sequence representations. Protein Sci. 12 (5), 1007–1017. doi:10.1110/ps.0239403
Nokhodian, Z., Ranjbar, M. M., Nasri, P., Kassaian, N., Shoaei, P., Vakili, B., et al. (2020). Current status of COVID-19 pandemic; characteristics, diagnosis, prevention, and treatment. J. Res. Med. Sci. 25, 101. doi:10.4103/jrms.jrms_476_20
Parmar, M., Thumar, R., Sheth, J., and Patel, D. (2022). Designing multi-epitope based peptide vaccine targeting spike protein SARS-CoV-2 B1.1.529 (Omicron) variant using computational approaches. Struct. Chem. 33 (6), 2243–2260. doi:10.1007/s11224-022-02027-6
Pettersen, E. F., Goddard, T. D., Huang, C. C., Couch, G. S., Greenblatt, D. M., Meng, E. C., et al. (2004). UCSF Chimera--a visualization system for exploratory research and analysis. J. Comput. Chem. 25 (13), 1605–1612. doi:10.1002/jcc.20084
Ponomarenko, J., Bui, H. H., Li, W., Fusseder, N., Bourne, P. E., Sette, A., et al. (2008). ElliPro: A new structure-based tool for the prediction of antibody epitopes. BMC Bioinforma. 9, 514. doi:10.1186/1471-2105-9-514
Rabie, A. M. (2021a). Two antioxidant 2,5-disubstituted-1,3,4-oxadiazoles (CoViTris2020 and ChloViD2020): Successful repurposing against COVID-19 as the first potent multitarget anti-SARS-CoV-2 drugs. New J. Chem. 45 (2), 761–771. doi:10.1039/d0nj03708g
Rabie, A. M. (2021b). Potent toxic effects of Taroxaz-104 on the replication of SARS-CoV-2 particles. Chemico-Biological Interact. 343, 109480. doi:10.1016/j.cbi.2021.109480
Rabie, A. M. (2021c). Teriflunomide: a possible effective drug for the comprehensive treatment of COVID-19. Curr. Res. Pharmacol. Drug Discov. 2, 100055. doi:10.1016/j.crphar.2021.100055
Rabie, A. M. (2022a). Potent inhibitory activities of the adenosine analogue cordycepin on SARS-CoV-2 replication. ACS Omega 7 (3), 2960–2969. doi:10.1021/acsomega.1c05998
Rabie, A. M. (2022b). Efficacious preclinical repurposing of the nucleoside analogue didanosine against COVID-19 polymerase and exonuclease. ACS Omega 7 (25), 21385–21396. doi:10.1021/acsomega.1c07095
Rabie, A. M., and Abdalla, M. (2022). Forodesine and riboprine exhibit strong anti-SARS-CoV-2 repurposing potential: in silico and in vitro studies. ACS Bio Med Chem Au 2 (6), 565–585. doi:10.1021/acsbiomedchemau.2c00039
Rabie, A. M., and Abdalla, M. (2023). Evaluation of a series of nucleoside analogs as effective anticoronaviral-2 drugs against the Omicron-B.1.1.529/BA.2 subvariant: a repurposing research study. Med. Chem. Res. 32 (2), 326–341. doi:10.1007/s00044-022-02970-3
Rabie, A. M., and Eltayb, W. A. (2023). Potent dual polymerase/exonuclease inhibitory activities of antioxidant aminothiadiazoles against the COVID-19 Omicron virus: a promising in silico/in vitro repositioning research study. Mol. Biotechnol. 24, 1–20. doi:10.1007/s12033-022-00551-8
Reche, P. A., Glutting, J. P., Zhang, H., and Reinherz, E. L. (2004). Enhancement to the RANKPEP resource for the prediction of peptide binding to MHC molecules using profiles. Immunogenetics 56 (6), 405–419. doi:10.1007/s00251-004-0709-7
Requena, D., Médico, A., Chacón, R. D., Ramírez, M., and Marín-Sánchez, O. (2020). Identification of novel candidate epitopes on SARS-CoV-2 proteins for south America: a review of HLA frequencies by country. Front. Immunol. 11, 2008. doi:10.3389/fimmu.2020.02008
Saha, S., and Raghava, G. P. (2006). Prediction of continuous B-cell epitopes in an antigen using recurrent neural network. Proteins 65 (1), 40–48. doi:10.1002/prot.21078
Schmid, N., Eichenberger, A. P., Choutko, A., Riniker, S., Winger, M., Mark, A. E., et al. (2011). Definition and testing of the GROMOS force-field versions 54A7 and 54B7. Eur. biophysics J. EBJ 40 (7), 843–856. doi:10.1007/s00249-011-0700-9
Schneidman-Duhovny, D., Inbar, Y., Nussinov, R., and Wolfson, H. J. (2005). PatchDock and SymmDock: Servers for rigid and symmetric docking. Nucleic acids Res. 33 (Web Server issue), W363–W367. doi:10.1093/nar/gki481
Sharma, P., Joshi, T., Joshi, T., Mathpal, S., Maiti, P., Nand, M., et al. (2023). In silico screening of natural compounds to inhibit interaction of human ACE2 receptor and spike protein of SARS-CoV-2 for the prevention of COVID-19. J. Biomol. Struct. Dyn. 41 (2), 646–658. doi:10.1080/07391102.2021.2010599
Singh, H., and Raghava, G. P. (2001). ProPred: Prediction of HLA-DR binding sites. Bioinforma. Oxf. Engl. 17 (12), 1236–1237. doi:10.1093/bioinformatics/17.12.1236
Tong, Q., Liu, G., Sang, X., Zhu, X., Fu, X., Dou, C., et al. (2023). Targeting RNA G-quadruplex with repurposed drugs blocks SARS-CoV-2 entry. PLoS Pathog. 19 (1), e1011131. doi:10.1371/journal.ppat.1011131
UniProt Consortium (2019). UniProt: A worldwide hub of protein knowledge. Nucleic acids Res. 47 (D1), D506–D515. doi:10.1093/nar/gky1049
Wiederstein, M., and Sippl, M. J. (2007). ProSA-web: Interactive web service for the recognition of errors in three-dimensional structures of proteins. Nucleic Acids Res. 35 (Web Server issue), W407–W410. doi:10.1093/nar/gkm290
Wilkins, M. R., Gasteiger, E., Bairoch, A., Sanchez, J. C., Williams, K. L., Appel, R. D., et al. (1999). Protein identification and analysis tools in the ExPASy server. Methods Mol. Biol. 112, 531–552. doi:10.1385/1-59259-584-7:531
Yang, J., Anishchenko, I., Park, H., Peng, Z., Ovchinnikov, S., and Baker, D. (2020). Improved protein structure prediction using predicted interresidue orientations. Proc. Natl. Acad. Sci. U. S. A. 117 (3), 1496–1503. doi:10.1073/pnas.1914677117
Yang, J., and Zhang, Y. (2015). I-TASSER server: New development for protein structure and function predictions. Nucleic acids Res. 43 (W1), W174–W181. doi:10.1093/nar/gkv342
Yao, B., Zhang, L., Liang, S., and Zhang, C. (2012). SVMTriP: A method to predict antigenic epitopes using support vector machine to integrate tri-peptide similarity and propensity. PloS One 7 (9), e45152. doi:10.1371/journal.pone.0045152
Keywords: SARS-CoV-2, COVID-19, multi-epitope, vaccine, dynamic molecular simulation
Citation: Alibakhshi A, Alagheband Bahrami A, Mohammadi E, Ahangarzadeh S and Mobasheri M (2024) In-silico design of a new multi-epitope vaccine candidate against SARS-CoV-2. Acta Virol. 67:12481. doi: 10.3389/av.2023.12481
Received: 23 March 2023; Accepted: 22 November 2023;
Published: 04 January 2024.
Edited by:
Katarina Polcicova, Slovak Academy of Sciences, SlovakiaCopyright © 2024 Alibakhshi, Alagheband Bahrami, Mohammadi, Ahangarzadeh and Mobasheri. This is an open-access article distributed under the terms of the Creative Commons Attribution License (CC BY). The use, distribution or reproduction in other forums is permitted, provided the original author(s) and the copyright owner(s) are credited and that the original publication in this journal is cited, in accordance with accepted academic practice. No use, distribution or reproduction is permitted which does not comply with these terms.
*Correspondence: Abbas Alibakhshi, alibakhshi2630@yahoo.com; Shahrzad Ahangarzadeh, shahrzadahangar@yahoo.com