- Food Security and Safety Focus Area, Faculty of Natural and Agriculture Sciences, North-West University, Mmabatho, South Africa
Metagenomics is a scientific breakthrough that can reveal the variations in the microbial diversities and functions between the healthy and diseased plants, towards a productive deployment in diverse biotechnological processes and agricultural activities. This study investigated the possible functional diversity in the rhizosphere microbiome of both healthy and Northern Corn Leaf Blight (NCLB) infected maize growing at farms in the Lichtenburg (LI) and Mafikeng (MA) areas of the North West Province, South Africa. We hypothesized variations in the abundance and diversities of microbial functions in the healthy (LI and MA) and diseased (LID and MAD) maize plants. Hence, we extracted DNA from the healthy and diseased maize rhizosphere in the two maize farms and sequenced using a shotgun approach. Using the SEED subsystem, we discovered that the healthy rhizosphere maize plant was dominated by 24 functional categories, while the NCLB infected rhizosphere maize plant was dominated by 4 functional categories. Alpha diversity analysis showed no significant (p > 0.05) difference between the healthy and diseased maize rhizosphere. However, the analysis of beta diversity showed a significant difference. The substantial abundance of functional groups detected especially in LI indicates that presence of plant diseases altered the functions of soil microbiomes. The significant abundance of the unknown role of rhizosphere microbiomes in disease management suggests the presence of some undiscovered functional genes associated with the microbiome of the healthy maize rhizosphere. Hence, further investigation is needed to explore the roles of these functional genes for their agricultural or biotechnological relevance.
Introduction
Maize (Zea mays L.) is one of the world’s most popular and fastest-growing economically important cereal crops. It is the most cultivated cereal across the globe, which forms a food staple for over 900 million people and one-third of malnourished children across the globe. Despite its importance, the quality and quantity of maize is being reduced by a variety of diseases including bacteria, viruses, mycoplasma-like organisms, nematodes, and other higher parasitic plants (Peiffer et al., 2013; ur Rehman et al., 2021). This results in up to 9.4% annual losses across the world, which directly affects human health and income generation (Bandyopadhyay et al., 2019; Seyi-Amole and Onilude, 2021). Some of the most common diseases of maize limiting maize output worldwide include gray leaf spots, various types of rust diseases, southern corn leaf blight (SCLB), and northern corn leaf blight (NCLB) (Naeem et al., 2021). Northern Corn Leaf Blight caused by Exserohilum turcicum is a serious foliar disease of maize distributed widely across the world and is more prevalent in humid weather with temperature between 20°C–28°C. It has been reported to causing a range of 27.6%–97.5% yield loss (Kumar et al., 2011; Craven et al., 2020).
Several disease management approaches have been employed in ameliorating the impact of NCLB, including improved management practices (conventional tillage, crop rotation), foliar fungicide use, biological control, and planting resistant cultivars (Galiano-Carneiro and Miedaner, 2017; Ranganatha et al., 2017). However, the proposed solution to the current threat to food security and safety reportedly lies in the sustainable utilization of beneficial microorganisms to achievelong-term agricultural production, with minimal or no adverse impact to the ecosystem, since plants selectively recruit microbes from the soil to build a diverse, yet stable and predictable microbial community in their root region (Adedeji et al., 2020), which provides them with specific benefits, especially plant disease resistance (Akanmu et al., 2021; Fadiji et al., 2021b). The process entails the suppression of pathogens in the rhizosphere by secretion of antimicrobial chemicals, competition within an ecological niche (i.e., nutrition), or through induced systemic resistance (ISR). Thus, the microbiome can indirectly boost the capacity of resistant pathogens to regulate the plant immune system (Qu et al., 2020).
The rhizosphere microbiome is the initial line of defense against soil-borne infections, and the ecological connections between plant and soil microbes are the primary determinant of whether or not the pathogen can infect the roots (Chiaramonte et al., 2021). More so, the associated microbial structure and functions are dependent on the soil physicochemical status which plays a significant role in the ecological processes and functions. It also aids the plant-microbes relationship such as the stimulation of plant growth, mineralization, and rapid decomposition of soil organic matter (Akinola et al., 2021). Rhizosphere organisms have been extensively investigated for their beneficial effects on plant development and health, including nitrogen-fixing bacteria, mycorrhizal fungi, plant growth-promoting rhizobacteria (PGPR), biocontrol microorganisms, mycoparasitic fungi, and protozoa (Mendes et al., 2013). Although the interaction between plants and microbes have been widely explored, limited information exists on the impact of the vast majority of plant-associated microbes on plant development, health, and disease. As a result, understanding the functional roles of plant microbiome is essential in enhancing the plant health and productivity.
The rhizosphere microbial populations have been extensively studied using culture-dependent methodologies (Ajiboye et al., 2022). The drawback of this approach is the inability to sufficiently elucidate the roles of soil microorganisms in a specific environment (Fadiji et al., 2021c). Whereas, shotgun metagenomics has gained more relevance for its application in employing both the culture-dependent and culture-independent methodologies in studying the community profiles and functional diversities of microbial populations present in an environment. Rather than focusing on a specific genomic location, shotgun metagenomic sequencing analyzes all DNA samples taken from a certain environment (Fadiji et al., 2021a).
We hypothesize using a shotgun metagenomics method, that an invasive pathogen, either directly or indirectly through the plant, triggers stress responses in the microbial community, which lead to the activating antagonistic traits and shift in the microbiome composition that restricts pathogen invasion. Upon pathogenic attack, plants can use microbial assemblages from the soil for protection against infections (Mendes, 2018). Since shotgun metagenomics enables researchers to decipher the functional variety of microbiomes in a given context (Fadiji et al., 2021c), knowledge of the relationship between microbial distribution and function could be harnessed to explore new strains of microbes useful for agricultural use while elucidating the role of rhizosphere microbiome in reducing disease impacts. Therefore, profiling the functional characteristics of microbial communities in the maize rhizosphere is critical to linking rhizosphere soils of healthy and NCLB diseased maize plants with varied functions that enhance plant and soil health, thus ensuring sustainable maize production. Hence, this study aims at unveiling the functional diversity of microbes in the maize rhizosphere of both healthy and NCLB infected maize plants using a shotgun metagenomics approach.
Materials and Methods
History of the Sampled Fields
The maize rhizosphere soil samples used in this research were collected from two different locations within the North West Province in South Africa. The North-West University farm in Mafikeng (25°47′19.1″S 25°37′05.1″E) and a commercial farm in Lichtenburg (25⁰59′40.2″ S, 26°31′44.2″E) were sampled in this study. The province has a summer season between October and March, the typical rainfall ranges from 300 to 700 mm per annum and temperatures from 22°C to 34°C. The average winter temperature is 16°C, with the hottest months being May and July. The maize cultivar WE 3127 was planted in both sites for the sample collection period. The Lichtenburg (LI) farm has been noted for maize monoculture practice for the past 10 years, while the University farm (MA) has been exposed to the rotational cultivation of maize and cowpea since 1989. The two farms have a history of synthetic pesticides and NPK fertilizer use, mechanized land tilling systems, and artificial irrigation techniques. The choice of the locations was influenced by the occurrence of Northern corn leaf blight (NCLB) disease in some parts of the farms. Identification of NCLB diseased plants was based on the occurrence of symptoms on the leaves, such as elliptical, grey-green lesions of 3–15 cm long, tan with distinct dark zones of fungal sporulation, which turns to a blight that becomes severe in its advanced stage (Vieira et al., 2014). Soil samples were collected from the rhizosphere of both healthy and NCLB-infected plants from each of the farms at about 8 weeks after planting.
Sample Collection
The sample collection from the two selected maize growing farms (MA and LI) was carried out in January 2021. To enable adequate representation of the field sampled, each field was demarcated into two areas, and each area was independently sampled for the healthy (15 samples) and NCLB infected (15 samples). Each sampled plant was uprooted and gently shaken to remove the loose soils while soil tightly bound to the root were carefully scrapped into separate sterile bags. The respective samples from heathy and diseased maize collected from each farm area were then pooled into two replicates and labelled accordingly. Hence, a total of four samples was collected from each farm sampled. These were transferred into iced-cooler boxes (4°C), and transported to the laboratory where the samples were stored at −20°C until DNA extraction for high throughput sequencing to be conducted.
Physicochemical Analysis of the Soil Samples
Prior to the use of each soil sample for physicochemical analysis, they were separately ground and sieved with a 2 mm sieve. Determination of the total nitrogen content was conducted according to the method of Sáez-Plaza et al. (2013). pH was determined using a pH meter in the soil, the distilled water ratio was 1:2.5. Bray No. 1 solution was used as the extractant when determining the phosphorous level of the soil (Bray and Kurtz, 1945), the phosphorus extracted was quantified colorimetrically, based on the development of the molybdenum blue colour with ammonium molybdate. The compound’s absorbance, as measured by a spectrophotometer at 882 nm, was directly proportional to the quantity of phosphorus extracted from the soil. The exchangeable N-NO3 and N-NH4 were determined using 1 M KCl solution according to the method described by Kachurina et al. (2000). Spectrophotometric measurements of the absorbance were made at 260 and 220 nm, respectively. The dry combustion technique was used to calculate the total amounts of N and C (Wright and Bailey, 2001). The exchangeable potassium and sodium were measured by 1 mM ammonium acetate analysis at neutral pH, whereas the sulphur was identified by HCl extraction. The loss of ignition approach was used to calculate organic matter (Hoogsteen et al., 2015). Determination of soil organic carbon was conducted in line with the procedure explained by Walkley and Black (1934).
DNA Extraction and Shotgun Sequencing
The samples of rhizosphere soils collected were subjected to the DNA extraction protocol of the Qiagen DNeasy PowerSoil Pro Kit (Qiagen, Hilden, Germany). From each sample, 0.25 g was measured for DNA extraction. The shotgun approach of the whole-metagenome sequencing method generated the datasets used in this investigation. This was carried out at the Molecular Research LP, Texas, United States. The DNA concentration of the samples were obtained using a life technologies assay kit-Qubit® dsDNA HS. The libraries were then prepared with 20–50 ng of DNA using the Illumina DNA Prep (M) tagmentation library preparation kit in line with the manufacturer’s instructions, and samples were fragmented and adapter sequences added. Next, a limited-cycle PCR was performed using the adapters, and the material was supplemented using unique indices. The library preparation was followed by the ultimate concentration of the libraries, after which the final concentration of the prepared libraries was determined using the Qubit® dsDNA HS Assay Kit (Life Technologies), and the average size of the library was determined using the Agilent 2100 Bioanalyzer (Agilent Technologies, United States). The libraries were pooled and diluted to 0.6 nM equimolar ratios and sequenced at the paired end for 300 cycles on the NovaSeq 6000 system (Illumina, United States).
Metagenome Annotation and Data Analysis
The metagenome sequences were uploaded to the metagenomics rapid annotation online service (MG-RAST) (Meyer et al., 2008), which performed the quality control steps involving dereplication, which removes artificial sequences, ambiguous base-filtering, and host species-specific sequences. After which, the annotation of the sequences was carried out with BLAST algorithm (Kent, 2002) against the M5NR database (Wilke et al., 2012) which permits non-redundant alignment of several databases. The RDP database was used to classify organisms according to their taxonomy, and the SEED subsystem database’s default settings were used to assign functional categories from level 1 to level 3 to the organisms. While constructing the functional table, the failed sequences during annotation were deleted, while the unclassified reads were retained for statistical analysis. The percentages were calculated and the functional table arranged for each functional level. The raw sequences associated with this study were deposited with NCBI under the BioProject accession number PRJNA821718. The variations in soil physicochemical properties between the rhizosphere soil of the healthy and NCLB diseased maize were calculated using a one-way ANOVA and Tukey’s pairwise comparison test.
The Shannon diversity and Pielou Evenness indices of the samples were determined, and the indices between the sites were compared with the Kruskal–Wallis test, and the test of significance was determined by a Monte Carlo permutation test using 9999 random permutations. The variations in the community composition observed within the same sample group were determined by PAST version 3.20. The beta diversity was determined by using primary coordinate analysis based on the Euclidean distance matrix using one-way analysis of similarities (ANOSIM) (Carrell and Frank, 2015). The Shiny heat map was used to plot the heat maps (Khomtchouk et al., 2017). The PCA and PCoA were plotted using Canoco software (ter Braak and Šmilauer, 2012).
Results
Physicochemical Analysis of NCLB Diseased and Healthy Maize Rhizosphere Soil
The chemical properties of soil samples in Lichtenburg generally recorded higher significance p < 0.05 than samples obtained from the maize farm in Mafikeng, except N-NH4 (mg/kg) (Table 1). The pH in both LI and LID are close to neutral while MA and MAD are slightly acidic. With the exception of N-NH4 in MA and MAD, all the samples expressed small quantities of total carbon, sulphur, organic carbon, organic matter, and ammonium, while higher quantities of phosphorous and potassium contents were recorded. Generally, more significant (p < 0.05) amounts were observed in LI and LID, compared to MA and MAD (Table 2).
Metagenomics Sequencing and Processing
The mean values of samples from the rhizosphere of healthy maize in Lichtenburg (LI) (1,615,034,280 bp) and Mafikeng (MA) (7,255,180,845) were found in the sequences uploaded for analysis on the MG-RAST server, while the sequence count for rhizosphere soils from NCLB diseased plants in Lichtenburg (LID) and Mafikeng (MAD) was 526,054,117 and 1,105,047,462. However, following QC, the quality of retained mean sequences revealed LI = 1,165,623,372 and MA = 673,172,171 for samples from healthy rhizosphere soils, and LID = 4,876,551,985 and MAD = 1,025,190,816 for samples from the diseased plant rhizosphere soils. The abundance and diversity of microbial communities in the rhizosphere are depicted by the rarefaction generated by MG-RAST database.
Taxonomy and Functional Analyses of Rhizosphere Microbiomes Associated With Healthy and Diseased Maize Plant
The predominant phyla across the rhizosphere samples were Proteobacteria, Actinobacteria, Streptophyta, Acidobacteria, and Bacteroidetes while Euryarchaeota and Ascomycota were the major Archaea and Fungal phyla. Higher abundance of the microbial community was evident in samples from the healthy than diseased rhizosphere, especially in Lichtenburg (Table 3).
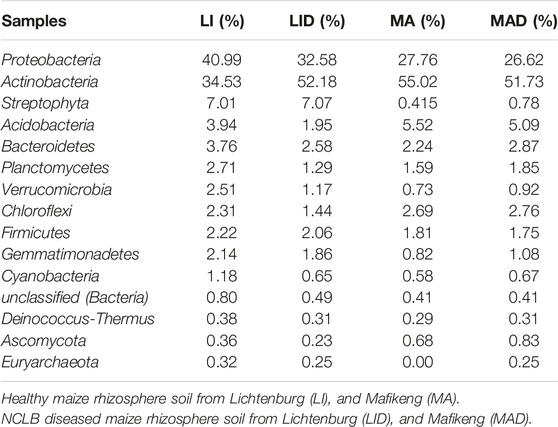
TABLE 3. Taxonomic analyses of rhizosphere microbiomes associated with healthy and diseased maize plants.
Across the sampled sites investigated, a total of 28 functions of the microbial profile with variations in their relative abundances were obtained at level 1 through the SEED subsystem hierarchical gene annotation. The functional categories dominated samples from the LI site. Functions recorded in these samples including, virulence, stress response, disease and defense, RNA metabolism, membrane transport, cell wall and capsule, sulfur metabolism, regulation and cell signaling. Others include cell division and cell cycle, phosphorus metabolism, nitrogen metabolism, iron acquisition and metabolism, potassium metabolism, miscellaneous and protein metabolism. These functions were more predominant rhizosphere samples from the LI site while nucleosides and nucleotides, DNA metabolism, photosynthesis, and clustering-based subsystems dominated the samples from the LID site.
The dominant functional categories as obtained from the MA site were fatty acids, isoprenoids and lipids, respiration, metabolism of aromatic compounds, motility and chemotaxis, prophages, phages, plasmids, transposable elements, dormancy and sporulation, cofactors, prosthetic groups, vitamins, pigments, carbohydrates, amino acids, and derivatives as observed in samples from the MA sites, while secondary metabolism, clustering-based subsystems, and miscellaneous dominated the MAD samples (Figure 1).
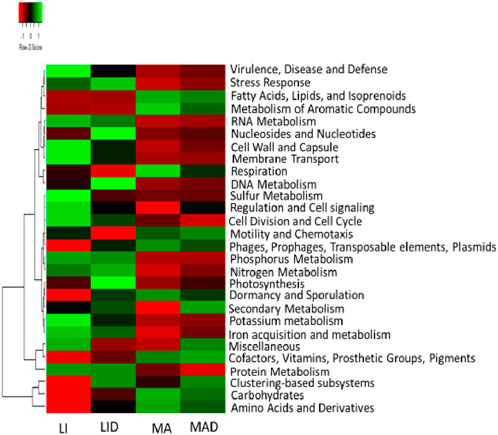
FIGURE 1. Heatmap of the subsystem level 1 showing the key function of the microbiome. The color saturation gradient of the scale bar represents the relative abundances with a z-score. Healthy maize rhizosphere soil from Lichtenburg (LI), and Mafikeng (MA). NCLB diseased maize rhizosphere soil from Lichtenburg (LID), and Mafikeng (MAD).
Principal component analysis (PCA) was used to examine the distribution of different functional categories between samples from the healthy rhizosphere (LI and MA) and the diseased rhizosphere (LID and MAD) (Figure 2). No significant variation was observed in the functional categories observed across the study sites (Table 4). The findings showed that the LI site was dominated by 16 functional categories, the MA site by 10, the LI site by 4, and the LID site by 3 functional categories (Figure 3). The Lichtenburg location had 13 and 4 main functional categories dominating LI and LID, respectively, whereas the Mafikeng sites had 8 and 4 major functional categories which dominated MA and MAD, respectively.
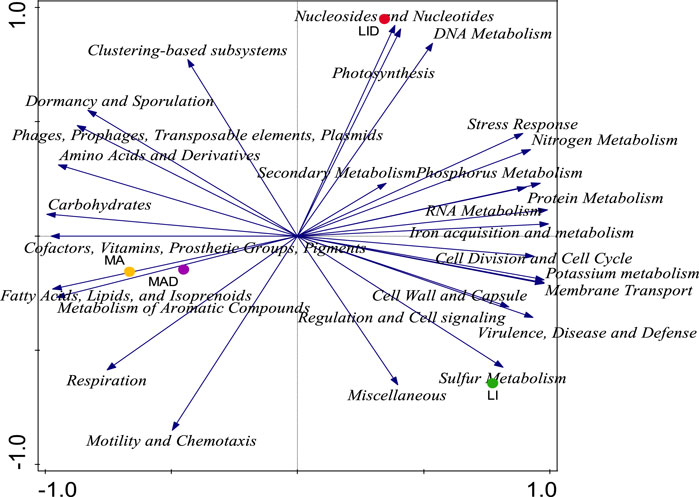
FIGURE 2. PCA analysis showing a graph of the distribution of major microbial function of the healthy (LI and MA) and diseased (LID and MAD) rhizosphere soil samples. PC-Axis 1 (88.6%) and PC-Axis 2 (10.1%), the variations were described based on Euclidean dissimilarities. Healthy maize rhizosphere soil from Lichtenburg (LI), and Mafikeng (MA). NCLB diseased maize rhizosphere soil from Lichtenburg (LID), and Mafikeng (MAD).

TABLE 4. Diversity evaluation of Evenness, Simpson, and Shannon indices of microbial community at level 1 of the SEED subsystem across the sampled sites.
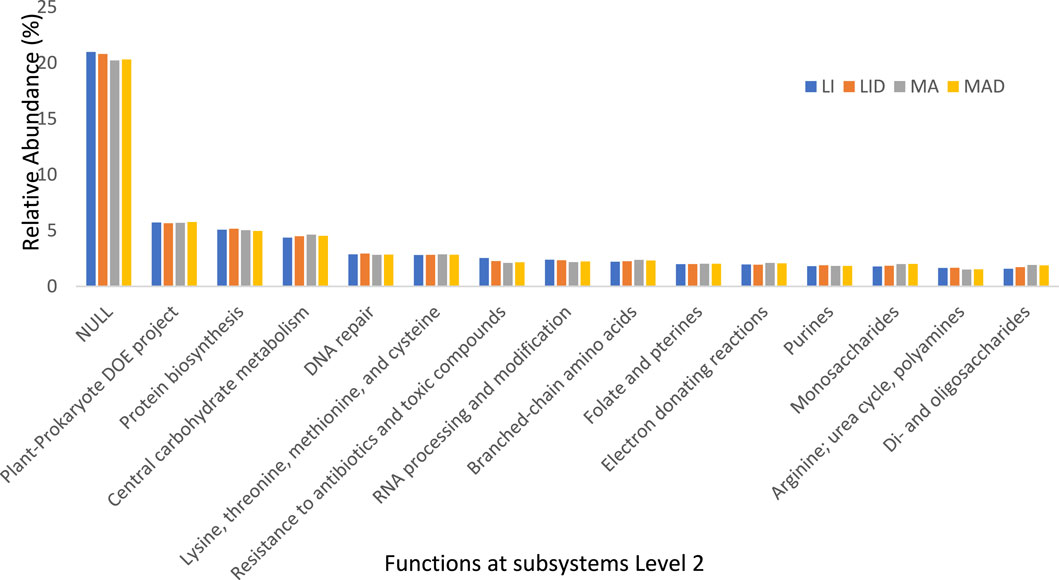
FIGURE 3. The bar chart representing the functional groups obtained at level 2 of the SEED subsystems. Healthy maize rhizosphere soil from Lichtenburg (LI), and Mafikeng (MA). NCLB diseased maize rhizosphere soil from Lichtenburg (LID), and Mafikeng (MAD).
Unknown functions were shown to be more prevalent at the subsystem Level 2 hierarchy for gene annotation across all locations. The unknown protein had the highest relative abundance of 20.99% (LI), 20.8% (LID), 20.2% (MA), and 20.3% (MAD). This was followed by the plant-prokaryote DOE project with 5.71% (LI), 5.66% (LID), 5.7% (MA), and 5.76% (MAD). The abundance of protein biosynthesis was 5.08% (LI), 5.16% (LID), 5.02% (MA), and 4.95% (MAD) across the sites (Figure 3).
The variety of the functional categories at SEED subsystem level 1 was examined using the evenness, Simpson, and Shannon indices. The findings showed no significant (p > 0.05) variation (Table 4). The amount of variation in functional diversity among the sites was analyzed using the Kruskal–Wallis method and found to be non-significant (p = 0.99). The PCoA graph revealed that the relative abundance of the 28 functional categories found at SEED Subsystems level 1 differed significantly across LI and LID sites and MA and MAD sites (Supplementary Figure S1A). To see if the locations were similar, one-way ANOSIM was utilized. The findings demonstrated a statistically significant (p < 0.05) difference in the 28 functional categories from all samples across the locations (R = 0.58 and p = 0.01).
Pathways of the Metabolic Functions of the Microbial Communities in the Soil Samples
The Subsystems at level 3 expressed the sequences involved in the pathways present in the sulfur metabolism, carbohydrates metabolism, nitrogen metabolism, disease and defense, virulence, secondary metabolism, and phosphorus metabolism. From the sulfur metabolism pathways, there was an abundance of sulfur oxidation, sulfatide metabolism and galactosylceramide, thioredoxin-disulfide reductase, utilization of glutathione, taurine utilization, DMSP breakdown, sulfate reduction associated complexes, alkanesulfonates utilization in the LI site while the MA site obtained an abundance of L-cystine uptake and metabolism, release of Dimethyl sulfide (DMS) from Dimethylsulfoniopropionate (DMSP) and inorganic sulfur assimilation. However, the same value was obtained as the relative abundance of alkanesulfonate assimilation in the two sites in F1 (LI and MAD). The abundance of sulfur oxidation, utilization of glutathione, DMSP breakdown and sulfate reduction associated complexes was higher in the LI rhizosphere than the LID, MA, and MAD rhizosphere samples (Supplementary Figure S1B and Supplementary Table S2). However, the relative abundances of alkanesulfonate assimilation and galactosylceramide and sulfatide metabolism in the LID rhizosphere were higher than those obtained from the LI, MA, and MAD rhizosphere samples. In the MA rhizosphere samples, the was a higher abundance of L-cystine uptake and metabolism, release of Dimethyl sulfide (DMS) from Dimethylsulfoniopropionate (DMSP) and Inorganic sulfur assimilation obtained than in LI, LID, and MAD. Also, the same relative abundance of 0.02% alkanesulfonates utilization and 0.03% L-cystine uptake and metabolism was observed for this metabolic pathway across all the rhizosphere samples from all sites.
For the pathway involved in utilization of glutathione, its relative abundances were the same (0.07%) in all rhizosphere samples except in LID, where its relative abundance was 0.06% (Supplementary Figure S1B and Supplementary Table S2). For the metabolic pathways linked with carbohydrate metabolism, there was abundance in sugar utilization in thermotogales, maltose and maltodextrin utilization, trehalose biosynthesis, entner-Doudoroff pathway, pentose phosphate pathway, Pyruvate metabolism II: acetyl-CoA L-rhamnose utilization, inositol catabolism, and photorespiration (oxidative C2 cycle) in the MA and MAD samples. The pathways including serine glyoxylate cycle, glycolysis and gluconeogenesis, and dehydrogenase complexes were more dominant in the MA rhizosphere sample while the calvin benson cycle was observed on the LID and MA samples. TCA cycle pathway was dominant in the LI rhizosphere sample, while Pyruvate metabolism I: PEP was only present in the LID rhizosphere sample. The same values were obtained for glycolysis and gluconeogenesis (0.46%) and Pyruvate metabolism II: acetyl-CoA L-rhamnose utilization (0.42%) for the diseased rhizosphere samples (LID and MAD). In TCA cycle the same values (0.57%) were obtained across all sites besides MAD which was (0.56%) (Supplementary Figure S2 and Supplementary Table S3). Sequences related to nitrogen metabolism were dominant in LI. Some metabolic pathways such as allantoin utilization, Nitrilase, Amidase clustered with urea and nitrile hydratase functions, and Nitric oxide synthase were more dominant in the MA site. The dominance of major metabolic pathways such as ammonia assimilation, denitrification, nitrosative stress, cyanate hydrolysis, nitrogen fixation, and dissimilatory nitrite reductase was also observed in the LI site, more on the healthy maize plant rhizosphere sample (Supplementary Figure S3 and Supplementary Table S4). The same value (0.01%) of Amidase clustered with urea and nitrile hydratase functions was obtained across all samples. In the results for virulence, disease, and defense, the metabolic pathways involved in beta-lactamase, arsenic resistance, copper homeostasis: copper tolerance, copper homeostasis, mercuric reductase, multidrug resistance efflux pumps, Multidrug efflux pump in Campylobacter jejuni (CmeABC operon), Methicillin resistance in Staphylococci, Streptococcus pyogenes Virulome, resistance to fluoroquinolones, resistance to chromium compounds and The mdtABCD multidrug resistance cluster were more dominant in the MA and MAD rhizosphere samples (Supplementary Figure S2A). Bacterial cyanide production and tolerance mechanisms, BlaR1 family regulatory sensor-transducer disambiguation, cobalt-zinc cadmium resistance, multilocus sequence typing (MLST), MexE-MexF-OprN multidrug efflux system, resistance to vancomycin, Streptococcus pyogenes virulome, and zinc resistance were dominant in the LI and LID site (Supplementary Figure S4 and Supplementary Table S5). Also, the multidrug resistance efflux pump relative abundances were higher in the diseased rhizosphere samples (LID and MAD). However, for the relative abundances observed in the metabolic pathways involved in Multidrug efflux pump in Campylobacter jejuni (CmeABC operon), Streptococcus agalactiae virulome and the mdtABCD multidrug resistance cluster were more dominant in the MA rhizosphere sample. We also observed that the pathway, Multidrug efflux pump in Campylobacter jejuni Campylobacter jejuni (CmeABC operon), and MexE-MexF-OprN multidrug efflux system, were the same (0.02%) in all rhizosphere samples (LI, LID, MA, and MAD). The dominance of some major metabolic pathways in phosphate metabolism was also established with the abundance of pathways such as phosphorus uptake, alkylphosphonate utilization, phosphonate metabolism, phosphoenolpyruvate phosphomutase, high affinity phosphate transporter, and control of PHO regulon and phosphate metabolism in the LI site (Supplementary Figure S5 and Supplementary Table S6). The abundance of Phosphate-binding_DING_proteins was observed in the MAD rhizosphere samples. The same value was obtained for phosphonate metabolism, phosphoenolpyruvate phosphomutase, and phosphate metabolism (0.01%) across all samples from the two sites. Furthermore, for the pathways involved in secondary metabolism, we observed that the metabolic pathways, including steroid sulfates, alkaloid biosynthesis from L-lysine, cinnamic acid degradation, and auxin degradation were relatively higher in LI and LID rhizosphere samples than those of the MA and MAD rhizosphere samples. The pathways of flavanone biosynthesis, Phenylpropionate degradation, Biflavanoid_biosynthesis, 2-isocapryloyl 3R hydroxymethyl-gamma butyrolactone and other bacterial morphogens, clavulanic acid biosynthesis, tannin biosynthesis, cannabinoid biosynthesis, non-ribosomal peptide synthetases (NRPS) in Frankia sp. Ccl3, and auxin biosynthesis were more present in the MA and MAD rhizosphere samples. Also, the pathways of cinnamic acid degradation, phenazine biosynthesis, and cannabinoid biosynthesis were present in the diseased rhizosphere samples (LID and MAD) (Supplementary Figure S6 and Supplementary Table S7). The same values were obtained for flavanone biosynthesis (0.02%), cinnamic acid degradation (0.02%), auxin degradation (0.03%), Non-ribosomal peptide synthetases (NRPS) in Frankia sp. Ccl3 (0.01%), and auxin biosynthesis (0.10%) across all rhizosphere samples from both sites in the study (Supplementary Figure S6 and Supplementary Table S7).
Discussion
Maize is a major food crop cultivated all over the world and is a strategic solution to supporting the food security of the growing human population that is estimated to reach 9 billion people by 2050 (Sucher et al., 2017; Tyczewska et al., 2018; Fadiji et al., 2021c). However, the quantity and quality of maize are often compromised by plant diseases which causes significant economic damage, with additional cost implications on disease management (Sucher et al., 2017). Northern corn leaf blight caused by Exserohilum turcicum (Pass.) is one of the most persistent and devastating foliar diseases of maize, causing more than 30% yield reduction (De Rossi et al., 2021; Naeem et al., 2021). Hence, achieving improved plant health and yield necessitates an increased understanding of the functions and nutrient pathways of the rhizosphere microbiome populations involved, particularly the beneficial ones engaged in agricultural sustainability, growth promotion, and ecosystem balance (Fadiji et al., 2021c).
In addition to a plant’s health status, soil types and plant root exudates have also been considered as contributory factors to variations in the microbial composition and abundance in maize rhizosphere. The physiological and functional properties of soil microbial populations mostly recorded in this study, Proteobacteria, Actinobacteria, Streptophyta, Acidobacteria, Ascomucota, and Euryarchaeota among others, have been reported for their roles in enhancing the soil nutrient status (Cordero et al., 2020; Adedayo et al., 2022). Moreso, bacterial diversity in many soils is significantly influenced by soil pH and abundance of most macronutrients is reportedly increased in alkaline soils (Molefe et al., 2021). This is consistent with the findings in this study, where the soil chemical properties recorded in the samples collected from Lichtenburg were mostly higher compared to those from Mafikeng, thus reaffirming the report of Vanlauwe et al. (2007) who discussed the variations in the soil chemical properties and soil fertility status across different fields. However, the ability of plants to create an environment rich in carbohydrates, aromatic compounds, and amino acids has been linked to their potential in altering the microbial diversity and selection in the rhizosphere.
Earlier investigations have explored several techniques involving both the culture dependent and culture independent including Pyrosequencing, 16SrRNA, and ITS in investigating the microbial communities (Lee et al., 2019). However, employing the shotgun metagenomic approach to study the healthy and NCLB diseased maize rhizosphere gives a further insight into the composition, diversity, and functions of the associated microorganisms. Although the non-significance of the alpha diversity across the samples revealed similar representation of the microbial activities and metabolic pathways of the SEED subsystem across the sampled sites, as confirmed by the Kruskal-Wallis’ test with a p-value 0.99 (Table 4). This was also verified by the cumulative variance of 98.75% (PCA 1 and 2) showing the extent of diversity in the study, as found in line with the report of Dinsdale et al. (2008).
Samples obtained from the diseased plant rhizosphere (LID and MAD) demonstrated higher relative abundance of some dominant functions including carbohydrates metabolism, cell division and cell cycle, iron acquisition and metabolism, motility, and chemotaxis as reflected in the subsystems at level 1. We also observed the metabolism of important mineral nutrients such as nitrogen, sulfur, phosphorus, and potassium (Figure 1). Meanwhile, mineral nutrition has a significant impact on the growth of plants and crop yields, which makes it an important contributor to sustainable agriculture (White and Brown, 2010; Fageria and Moreira, 2011). Furthermore, the microbial abundance in the healthy rhizosphere (LI and MA) is further evidence of the plant growth-promoting fungi (PGPF) and plant growth-promoting rhizobacteria (PGPR) activities of the associated microbes (Akanmu et al., 2021; Dlamini et al., 2022). Furthermore, the abundance of the unknown function observed from all sites at subsystem level 2, combined with clustering-based subsystems, indicate that there are several important functions connected with this rhizosphere microbiome that have yet to be discovered.
The findings show that the microbial communities across the samples support the growth and development of maize plants through the availability of important nutrients, especially in sample LI which showed increased prevalence of these metabolisms, this could be validated with the presence of organic and inorganic sulfur metabolism, potassium metabolism, and ABC transporters in the subsystems at level 2 (Figure 3). The level 3 metabolic pathways of sulfur metabolism also revealed that the samples were dominated with 12 pathways (Supplementary Figure S1B and Supplementary Table S2). Plants require sulphur biomolecules to develop effective defense mechanisms in response to a variety of stresses. Within the cell, Sulphur assimilates serve a critical role as structural components for essential cellular compounds. Furthermore, their function as a signaling agent for intracellular communication has been established (Ihsan et al., 2019). Our findings imply that microorganisms in the fields can create thioredoxin and use glutathione, a peptide that contains L-cysteine, as a cofactor to protect maize plants from oxidative stress (Shahid et al., 2014; Takagi and Ohtsu, 2016; Chukwuneme et al., 2021b).
From our results on chemical soil properties (Table 1), the sulfur contents of our samples were lower at Mafikeng site, especially in MAD soil, as a result, the presence of sulfur metabolic genes in our samples revealed that microorganisms maintain a healthy sulfur metabolism in their environments. Studies have shown that sulfur absorption in plants is critical to the natural sulfur cycle. The sulfur absorption route in plants fixes inorganic sulfur in the environment, such as sulfate ions in soil and sulfur dioxide gas in the air (Saito, 2004). The presence of nitrogen, sulfur, phosphorus, and potassium metabolism metabolic pathways in our samples thereby implies that soil bacteria play an important role in plant growth promotion and nutrient cycling (Etesami and Adl, 2020).
Our results also revealed that the 15 pathways linked with carbohydrate metabolism, dominated the MA and MAD sites (Supplementary Figure S2 and Supplementary Table S3). Microbial survival is reliant on the ability to use a variety of accessible carbohydrates as carbon and energy sources in any environment. Carbon is the most significant medium component since it provides energy to microorganisms and aids in their development as well as the generation of primary and secondary metabolites (Mekonnen et al., 2019). Our findings show that the microbial communities in our samples aid plants in collecting carbon through various metabolic pathways. The primary pathways for simple carbohydrate generation are gluconeogenesis and pentose phosphate shunt, and they have an important role as osmoregulatory chemicals in cells, particularly in stressful settings (Arnao et al., 2021). The glycolysis route allows glucose to be converted to pyruvate, which can be used as a respiratory substrate. If enzymes in the glycolysis route are suppressed, the amount of pyruvate used as a respiratory substrate decrease, and this produces an indirect effect on the electron transport chain through altering the TCA cycle. Furthermore, disruption to the TCA cycle has been linked to male sterility of the crop plant in the past (Geng et al., 2018).
In the results for virulence, disease, and defense, the 22 metabolic pathways were more dominant in the MA and MAD rhizosphere samples (Supplementary Figure S4 and Supplementary Table S5). The colonization of chick caeca by the metabolic pathway C. jejuni shows that the samples include antibiotic resistance genes (Fadiji et al., 2021c). Moreso, the pathways related to nitrogen metabolism were dominant in the LI site (Supplementary Figure S3 and Supplementary Table S4). The results showed lower levels of the nitrogen fixing pathway on the MA site. Cereals, just like other plants, interact with a variety of bacteria and fungi, including nitrogen-fixing bacteria known as diazotrophs. Since the levels of nitrogen-fixation achieved with nitrogen-fixing bacteria in cereals are insufficient to meet the plant’s needs increasing nitrogen-fixation in cereals has been the focus of several studies (Rosenblueth et al., 2018).
The dominance of some major metabolic pathways in phosphate metabolism was also established with the abundance recorded in LI (Supplementary Figure S5 and Supplementary Table S6). Phosphorus is also a crucial macronutrient for plants and an important biomolecule involved in energy metabolism. Phosphorus fertilizer is frequently used to improve plant soil since it is frequently scarce in the soil and is readily absorbed by plants (Akinola et al., 2021). The prevalence of phosphorus metabolism pathways in the samples suggests that soil bacteria have increased the mineralization, solubility, and availability of phosphorus for plant use (Chukwuneme et al., 2021a). Furthermore, the dominance of some major metabolic pathways in secondary metabolisms was also prevalent in the MAD samples (Supplementary Figure S6 and Supplementary Table S7). Non-ribosomal peptides (NRPs) and polyketides (PKs) are two of the most diverse families of secondary metabolites, encompassing a wide range of compounds with diverse functions such as iron scavenging siderophores, pigments that provide protection against a variety of stress factors, communication molecules, and antimicrobials. Microbes produce secondary metabolites which have antibacterial properties against the plant diseases. Plant defenses can also be induced by some microorganisms (Rat et al., 2021). Secondary metabolism, which involves the creation of many low-molecular-weight molecules known as metabolites by microorganisms as a representation of metabolic complexity, is an important feature of soil bacteria (Chukwuneme et al., 2021b). Despite the widespread recognition of the importance of the rhizosphere microbiome for plant growth, the vast majority of rhizosphere bacteria are still unknown.
Conclusion
Using a shotgun metagenomics approach, we conducted a comprehensive impact of northern corn leaf blight disease on the functional diversity of the maize rhizosphere microbiome. The study showed that the major functional groups were more prevalent in the rhizosphere microbial community of the disease than in healthy maize samples in the two locations studied. The rhizosphere microbiomes function in sustainable agricultural production by boosting plant growth and instilling disease resistance. Furthermore, we identified some metabolic pathway sequences associated with sulfur metabolism, carbohydrate metabolism, nitrogen metabolism, virulence, disease, defense, phosphorus metabolism, and secondary metabolism. It is possible that the soil microbes are well adapted to the agricultural management practices used in the soils based on the enrichment of the metabolic pathways linked to stress response, carbohydrate, nitrogen, and phosphorus metabolism in the soils. This enrichment also reflects the genetic potential of the various microbial communities to cycle nutrients, acquire carbon, and respond to environmental stresses in their habitats. The study also reported a large number of unknown functional groups, indicating that the maize rhizosphere microbial community contains several important functional genes.
Data Availability Statement
The datasets presented in this study can be found in online repositories. The names of the repository/repositories and accession number(s) can be found in the article/Supplementary Material. The standard sequence data obtained in this work have been deposited in the NCBI Sequence Read Archive (SRA) under accession number PRJNA821718.
Author Contributions
OB conceived the concepts and provided the resources and professional guide. All authors collected the samples, conducted the experiment, and were involved in the manuscript write-up. All authors consented to the submission of the manuscript for publication.
Funding
The study was funded by the National Research Foundation, South Africa (UID: 123634 and UID132595).
Conflict of Interest
The authors declare that the research was conducted in the absence of any commercial or financial relationships that could be construed as a potential conflict of interest.
Acknowledgments
SD and AA acknowledged the North-West University, South Africa for the Postgraduate and Postdoctoral fellowships. OB appreciates the National Research Foundation, South Africa, for the grants (UID: 123634 and UID132595) that support research in her laboratory.
Supplementary Material
The Supplementary Material for this article can be found online at: https://www.frontierspartnerships.org/articles/10.3389/sjss.2023.10964/full#supplementary-material
References
Adedayo, A. A., Fadiji, A. E., and Babalola, O. O. (2022). The Effects of Plant Health Status on the Community Structure and Metabolic Pathways of Rhizosphere Microbial Communities Associated with Solanum lycopersicum. Horticulturae 8, 404. doi:10.3390/horticulturae8050404
Adedeji, A. A., Häggblom, M. M., and Babalola, O. O. (2020). Sustainable Agriculture in Africa: Plant Growth-Promoting Rhizobacteria (PGPR) to the Rescue. Sci. Afr. 9, e00492. doi:10.1016/j.sciaf.2020.e00492
Ajiboye, T. T., Ayangbenro, A. S., and Babalola, O. O. (2022). Functional Diversity of Microbial Communities in the Soybean (Glycine Max L.) Rhizosphere from Free State, South Africa. Int. J. Mol. Sci. 23, 9422. doi:10.3390/ijms23169422
Akanmu, A. O., Babalola, O. O., Venturi, V., Ayilara, M. S., Adeleke, B. S., Amoo, A. E., et al. (2021). Plant Disease Management: Leveraging on the Plant-Microbe-Soil Interface in the Biorational Use of Organic Amendments. Front. Plant Sci. 12, 700507. doi:10.3389/fpls.2021.700507
Akinola, S. A., Ayangbenro, A. S., and Babalola, O. O. (2021). Metagenomic Insight into the Community Structure of Maize-Rhizosphere Bacteria as Predicted by Different Environmental Factors and Their Functioning within Plant Proximity. Microorganisms 9, 1419. doi:10.3390/microorganisms9071419
Arnao, M. B., Hernández-Ruiz, J., Cano, A., and Reiter, R. J. (2021). Melatonin and Carbohydrate Metabolism in Plant Cells. Plants 10, 1917. doi:10.3390/plants10091917
Bandyopadhyay, R., Cardwell, K., Ortega-Beltran, A., Schulthess, F., Meikle, W., Setamou, M., et al. (2019). “Identifying and Managing Plant Health Risks for Key African Crops: Maize,” in Critical Issues in Plant Health: 50 Years of Research in African Agriculture (Cambridge: Burleigh Dodds Science Publishing Limited), 173–212. doi:10.19103/AS.2018.0043.08
Bray, R. H., and Kurtz, L. T. (1945). Determination of Total, Organic, and Available Forms of Phosphorus in Soils. Soil Sci. 59, 39–46. doi:10.1097/00010694-194501000-00006
Carrell, A. A., and Frank, C. (2015). Bacterial Endophyte Communities in the Foliage of Coast Redwood and Giant Sequoia. Front. Microbiol. 6, 1008. doi:10.3389/fmicb.2015.01008
Chiaramonte, J. B., Mendes, L. W., and Mendes, R. (2021). “Rhizosphere Microbiome and Soil-Borne Diseases,” in Rhizosphere Biology: Interactions between Microbes and Plants (Berlin, Germany: Springer), 155–168.
Chukwuneme, C. F., Ayangbenro, A. S., and Babalola, O. O. (2021a). Impacts of Land-Use and Management Histories of Maize Fields on the Structure, Composition, and Metabolic Potentials of Microbial Communities. Curr. Plant Biol. 28, 100228. doi:10.1016/j.cpb.2021.100228
Chukwuneme, C. F., Ayangbenro, A. S., Babalola, O. O., and Kutu, F. R. (2021b). Functional Diversity of Microbial Communities in Two Contrasting Maize Rhizosphere Soils. Rhizosphere 17, 100282. doi:10.1016/j.rhisph.2020.100282
Cordero, J., de Freitas, J. R., and Germida, J. J. (2020). Bacterial Microbiome Associated with the Rhizosphere and Root Interior of Crops in Saskatchewan, Canada. Can. J. Microbiol. 66, 71–85. doi:10.1139/cjm-2019-0330
Craven, M., Morey, L., Abrahams, A., Njom, H. A., and van Rensburg, B. J. (2020). Effect of Northern Corn Leaf Blight Severity on Fusarium Ear Rot Incidence of Maize. South Afr. J. Sci. 116, 1–11. doi:10.17159/sajs.2020/8508
De Rossi, R., Guerra, F., Plazas, M., Vuletic, E., Brücher, E., Guerra, G., et al. (2021). Crop Damage, Economic Losses, and the Economic Damage Threshold for Northern Corn Leaf Blight. Crop Prot. 154, 105901. doi:10.1016/j.cropro.2021.105901
Dinsdale, E. A., Edwards, R. A., Hall, D., Angly, F., Breitbart, M., Brulc, J. M., et al. (2008). Functional Metagenomic Profiling of Nine Biomes. Nature 452, 629–632. doi:10.1038/nature06810
Dlamini, S. P., Akanmu, A. O., and Babalola, O. O. (2022). Rhizospheric Microorganisms: The Gateway to a Sustainable Plant Health. Front. Sustain. Food Syst. 6, 925802. doi:10.3389/fsufs.2022.925802
Etesami, H., and Adl, S. M. (2020). “Plant Growth-Promoting Rhizobacteria (PGPR) and Their Action Mechanisms in Availability of Nutrients to Plants,” in Phyto-Microbiome in Stress Regulation. Editors M. Kumar, V. Kumar, and R. Prasad (Singapore: Springer), 147–203. doi:10.1007/978-981-15-2576-6_9
Fadiji, A. E., Ayangbenro, A. S., and Babalola, O. O. (2021a). Shotgun Metagenomics Reveals the Functional Diversity of Root-Associated Endophytic Microbiomes in Maize Plant. Curr. Plant Biol. 25, 100195. doi:10.1016/j.cpb.2021.100195
Fadiji, A. E., Ayangbenro, A. S., and Babalola, O. O. (2021b). Unveiling the Putative Functional Genes Present in Root-Associated Endophytic Microbiome from Maize Plant Using the Shotgun Approach. J. Appl. Genet. 62, 339–351. doi:10.1007/s13353-021-00611-w
Fadiji, A. E., Kanu, J. O., and Babalola, O. O. (2021c). Impact of Cropping Systems on the Functional Diversity of Rhizosphere Microbial Communities Associated with Maize Plant: a Shotgun Approach. Archives Microbiol. 203, 3605–3613. doi:10.1007/s00203-021-02354-y
Fageria, N. K., and Moreira, A. (2011). The Role of Mineral Nutrition on Root Growth of Crop Plants. Adv. Agron. 110, 251–331. doi:10.1016/B978-0-12-385531-2.00004-9
Galiano-Carneiro, A. L., and Miedaner, T. (2017). Genetics of Resistance and Pathogenicity in the maize/Setosphaeria Turcica Pathosystem and Implications for Breeding. Front. Plant Sci. 8, 1490. doi:10.3389/fpls.2017.01490
Geng, X., Ye, J., Yang, X., Li, S., Zhang, L., and Song, X. (2018). Identification of Proteins Involved in Carbohydrate Metabolism and Energy Metabolism Pathways and Their Regulation of Cytoplasmic Male Sterility in Wheat. Int. J. Mol. Sci. 19, 324. doi:10.3390/ijms19020324
Hoogsteen, M. J., Lantinga, E. A., Bakker, E. J., Groot, J. C., and Tittonell, P. A. (2015). Estimating Soil Organic Carbon through Loss on Ignition: Effects of Ignition Conditions and Structural Water Loss. Eur. J. Soil Sci. 66, 320–328. doi:10.1111/ejss.12224
Ihsan, M. Z., Daur, I., Alghabari, F., Alzamanan, S., Rizwan, S., Ahmad, M., et al. (2019). Heat Stress and Plant Development: Role of Sulphur Metabolites and Management Strategies. Acta Agric. Scand. Sect. B - Soil Plant Sci. 69, 332–342. doi:10.1080/09064710.2019.1569715
Kachurina, O., Zhang, H., Raun, W., and Krenzer, E. (2000). Simultaneous Determination of Soil Aluminum, Ammonium- and Nitrate-Nitrogen Using 1 M Potassium Chloride Extraction. Commun. Soil Sci. Plant Analysis 31, 893–903. doi:10.1080/00103620009370485
Kent, W. J. (2002). BLAT—the BLAST-like Alignment Tool. Genome Res. 12, 656–664. doi:10.1101/gr.229202
Khomtchouk, B. B., Hennessy, J. R., and Wahlestedt, C. (2017). Shinyheatmap: Ultra Fast Low Memory Heatmap Web Interface for Big Data Genomics. PloS one 12, e0176334. doi:10.1371/journal.pone.0176334
Kumar, S., Pardurange Gowda, K., Pant, S., Shekhar, M., Kumar, B., Kaur, B., et al. (2011). Sources of Resistance to Exserohilum Turcicum (Pass.) and Puccinia Polysora (Underw.) Incitant of Turcicum Leaf Blight and Polysora Rust of Maize. Archives Phytopathology Plant Prot. 44, 528–536. doi:10.1080/03235400903145558
Lee, S., Kim, Y., Kim, J. M., Chu, B., Joa, J. H., Sang, M. K., et al. (2019). A Preliminary Examination of Bacterial, Archaeal, and Fungal Communities Inhabiting Different Rhizocompartments of Tomato Plants under Real-World Environments. Sci. Rep. 9, 9300–9315. doi:10.1038/s41598-019-45660-8
Mekonnen, E., Kebede, A., Tafesse, T., and Tafesse, M. (2019). Investigation of Carbon Substrate Utilization Patterns of Three Ureolytic Bacteria. Biocatal. Agric. Biotechnol. 22, 101429. doi:10.1016/j.bcab.2019.101429
Mendes, R., Garbeva, P., and Raaijmakers, J. M. (2013). The Rhizosphere Microbiome: Significance of Plant Beneficial, Plant Pathogenic, and Human Pathogenic Microorganisms. FEMS Microbiol. Rev. 37, 634–663. doi:10.1111/1574-6976.12028
Mendes, R. (2018). “Rhizosphere interactions for disease suppression and biocontrol Embrapa Meio Ambiente-Artigo em anais de congresso (ALICE),” in Australasian Soilborne Diseases Symposium (Adelaide, Australia: National Wine Centre of Australia), 10.
Meyer, F., Paarmann, D., D'Souza, M., Olson, R., Glass, E. M., Kubal, M., et al. (2008). The Metagenomics RAST Server–A Public Resource for the Automatic Phylogenetic and Functional Analysis of Metagenomes. BMC Bioinforma. 9, 386–388. doi:10.1186/1471-2105-9-386
Molefe, R. R., Amoo, A. E., and Babalola, O. O. (2021). Metagenomic Insights into the Bacterial Community Structure and Functional Potentials in the Rhizosphere Soil of Maize Plants. J. Plant Interact. 16, 258–269. doi:10.1080/17429145.2021.1936228
Naeem, S., Tahir, M., Khan, K., Hassan, A., and Ahmad, R. (2021). Morphological and Molecular Markers Based Screening of Maize Hybrids against Northern Corn Leaf Blight. Pak. J. Bot. 53, 301–308. doi:10.30848/pjb2021-1(5)
Peiffer, J. A., Spor, A., Koren, O., Jin, Z., Tringe, S. G., Dangl, J. L., et al. (2013). Diversity and Heritability of the Maize Rhizosphere Microbiome under Field Conditions. Proc. Natl. Acad. Sci. 110, 6548–6553. doi:10.1073/pnas.1302837110
Qu, Q., Zhang, Z., Peijnenburg, W., Liu, W., Lu, T., Hu, B., et al. (2020). Rhizosphere Microbiome Assembly and its Impact on Plant Growth. J. Agric. Food Chem. 68, 5024–5038. doi:10.1021/acs.jafc.0c00073
Ranganatha, H., Lohithaswa, H., and Anand, S. (2017). Understanding the Genetic Architecture of Resistance to Northern Corn Leaf Blight and Southern Corn Rust in Maize (Zea mays L.). Indian J. Genet. 77, 357–363. doi:10.5958/0975-6906.2017.00048.7
Rat, A., Naranjo, H. D., Krigas, N., Grigoriadou, K., Maloupa, E., Alonso, A. V., et al. (2021). Endophytic Bacteria from the Roots of the Medicinal Plant Alkanna Tinctoria Tausch (Boraginaceae): Exploration of Plant Growth Promoting Properties and Potential Role in the Production of Plant Secondary Metabolites. Front. Microbiol. 12, 633488. doi:10.3389/fmicb.2021.633488
Rosenblueth, M., Ormeño-Orrillo, E., López-López, A., Rogel, M. A., Reyes-Hernández, B. J., Martínez-Romero, J. C., et al. (2018). Nitrogen Fixation in Cereals. Front. Microbiol. 9, 1794. doi:10.3389/fmicb.2018.01794
Sáez-Plaza, P., Navas, M. J., Wybraniec, S., Michałowski, T., and Asuero, A. G. (2013). An Overview of the Kjeldahl Method of Nitrogen Determination. Part II. Sample Preparation, Working Scale, Instrumental Finish, and Quality Control. Crit. Rev. Anal. Chem. 43, 224–272. doi:10.1080/10408347.2012.751787
Saito, K. (2004). Sulfur Assimilatory Metabolism. The Long and Smelling Road. Plant Physiol. 136, 2443–2450. doi:10.1104/pp.104.046755
Seyi-Amole, D. O., and Onilude, A. A. (2021). “Microbiological Control: A New Age of Maize Production,” in Cereal Grains (London, UK: IntechOpen). doi:10.5772/intechopen.97464
Shahid, M., Pourrut, B., Dumat, C., Nadeem, M., Aslam, M., and Pinelli, E. (2014). Heavy-metal-induced Reactive Oxygen Species: Phytotoxicity and Physicochemical Changes in Plants. Rev. Environ. Contam. Toxicol. 232, 1–44. doi:10.1007/978-3-319-06746-9_1
Sucher, J., Boni, R., Yang, P., Rogowsky, P., Büchner, H., Kastner, C., et al. (2017). The Durable Wheat Disease Resistance Gene Lr34 Confers Common Rust and Northern Corn Leaf Blight Resistance in Maize. Plant Biotechnol. J. 15, 489–496. doi:10.1111/pbi.12647
Takagi, H., and Ohtsu, I. (2016). L-cysteine Metabolism and Fermentation in Microorganisms. Amino Acid. Ferment. 159, 129–151. doi:10.1007/10_2016_29
ter Braak, C. J., and Šmilauer, P. (2012). Canoco Reference Manual and User's Guide: Software for Ordination, version 5.0. Ithaca, NY, USA: Microcomputer Power.
Tyczewska, A., Woźniak, E., Gracz, J., Kuczyński, J., and Twardowski, T. (2018). Towards Food Security: Current State and Future Prospects of Agrobiotechnology. Trends Biotechnol. 36, 1219–1229. doi:10.1016/j.tibtech.2018.07.008
ur Rehman, F., Adnan, M., Kalsoom, M., Naz, N., Husnain, M. G., Ilahi, H., et al. (2021). Seed-borne Fungal Diseases of Maize (Zea mays L.): A Review. Agrinula J. Agroteknologi Dan. Perkeb. 4, 43–60. doi:10.36490/agri.v4i1.123
Vanlauwe, B., Tittonell, P., and Mukalama, J. (2007). “Within-farm Soil Fertility Gradients Affect Response of Maize to Fertiliser Application in Western Kenya,” in Advances in Integrated Soil Fertility Management in Sub-saharan Africa: Challenges and Opportunities (Berlin, Germany: Springer), 121–132.
Vieira, R. A., Mesquini, R. M., Silva, C. N., Hata, F. T., Tessmann, D. J., and Scapim, C. A. (2014). A New Diagrammatic Scale for the Assessment of Northern Corn Leaf Blight. Crop Prot. 56, 55–57. doi:10.1016/j.cropro.2011.04.018
Walkley, A., and Black, I. A. (1934). An Examination of the Degtjareff Method for Determining Soil Organic Matter, and a Proposed Modification of the Chromic Acid Titration Method. Soil Sci. 37, 29–38. doi:10.1097/00010694-193401000-00003
White, P. J., and Brown, P. (2010). Plant Nutrition for Sustainable Development and Global Health. Ann. Bot. 105, 1073–1080. doi:10.1093/aob/mcq085
Wilke, A., Harrison, T., Wilkening, J., Field, D., Glass, E. M., Kyrpides, N., et al. (2012). The M5nr: a Novel Non-redundant Database Containing Protein Sequences and Annotations from Multiple Sources and Associated Tools. BMC Bioinforma. 13, 141–145. doi:10.1186/1471-2105-13-141
Keywords: disease management, functional genes, plant health, root microbiomes, SEED subsystem
Citation: Dlamini SP, Akanmu AO and Babalola OO (2023) Variations in the Functional Diversity of Rhizosphere Microbiome of Healthy and Northern Corn Leaf Blight Infected Maize (Zea mays L.). Span. J. Soil Sci. 13:10964. doi: 10.3389/sjss.2023.10964
Received: 09 October 2022; Accepted: 04 January 2023;
Published: 20 January 2023.
Edited by:
Avelino Núñez-Delgado, University of Santiago de Compostela, SpainCopyright © 2023 Dlamini, Akanmu and Babalola. This is an open-access article distributed under the terms of the Creative Commons Attribution License (CC BY). The use, distribution or reproduction in other forums is permitted, provided the original author(s) and the copyright owner(s) are credited and that the original publication in this journal is cited, in accordance with accepted academic practice. No use, distribution or reproduction is permitted which does not comply with these terms.
*Correspondence: Olubukola Oluranti Babalola, b2x1YnVrb2xhLmJhYmFsb2xhQG53dS5hYy56YQ==