- 1Department of Microbiology, Immunology and Transplantation, KU Leuven, Leuven, Belgium
- 2Department of Nephrology and Medical Intensive Care, Charité Universitätsmedizin Berlin, Berlin, Germany
- 3Department of Nephrology, Radboud University Medical Center, Nijmegen, Netherlands
- 4Department of Nephrology and Dialysis, Medical University of Vienna, Vienna, Austria
- 5Department of Surgical Sciences, Sapienza University of Rome, Rome, Italy
- 6Paris Translational Research Center for Organ Transplantation, Hôpital Saint Louis, Paris, France
- 7University of Barcelona, Barcelona, Spain
- 8Department of Nephrology, Technical University of Munich, Munich, Germany
- 9Centre for Evidence in Transplantation, Nuffield Department of Surgical Sciences, University of Oxford, Oxford, United Kingdom
- 10Department of Internal Medicine, Erasmus MC Transplant Institute, University Medical Center Rotterdam, Rotterdam, Netherlands
- 11Department of General, Transplant and Thoracic Surgery, Medical University of Innsbruck, Innsbruck, Austria
- 12Paris Translational Research Center for Organ Transplantation, Hôpital Necker, Paris, France
In kidney transplant recipients, late graft failure is often multifactorial. In addition, primary endpoints in kidney transplantation studies seek to demonstrate the short-term efficacy and safety of clinical interventions. Although such endpoints might demonstrate short-term improvement in specific aspects of graft function or incidence of rejection, such findings do not automatically translate into meaningful long-term graft survival benefits. Combining many factors into a well-validated model is therefore more likely to predict long-term outcome and better reflect the complexity of late graft failure than using single endpoints. If conditional marketing authorization could be considered for therapies that aim to improve long-term outcomes following kidney transplantation, then the surrogate endpoint for graft failure in clinical trial settings needs clearer definition. This Consensus Report considers the potential benefits and drawbacks of several candidate surrogate endpoints (including estimated glomerular filtration rate, proteinuria, histological lesions, and donor-specific anti-human leukocyte antigen antibodies) and composite scoring systems. The content was created from information prepared by a working group within the European Society for Organ Transplantation (ESOT). The group submitted a Broad Scientific Advice request to the European Medicines Agency (EMA), June 2020: the request focused on clinical trial design and endpoints in kidney transplantation. Following discussion and refinement, the EMA made final recommendations to ESOT in December 2020 regarding the potential to use surrogate endpoints in clinical studies that aim to improving late graft failure.
Introduction
Key primary endpoints in kidney transplantation are recipient death, graft failure, biopsy-confirmed acute rejection, and graft (dys)function. These endpoints have clear roles in research that aims to improve short-term clinical outcomes after transplantation, and they are also the efficacy endpoints used most often in clinical trials (1). However, as improvement in short-term graft survival (by inhibition of early rejection) does not translate into an equally long-term improvement in graft survival, and as graft failure is rare in the early years following transplantation, better predictors of long-term graft outcome are needed for use in randomized controlled trials (RCT).
If conditional marketing authorization could be considered for therapies that aim to improve long-term outcomes [see Naesens et al., this issue (2)], then the surrogate endpoint for graft failure (i.e., loss of graft function; excluding death with a functioning graft) for use in RCT needs clearer definition. A good surrogate endpoint should fulfill four criteria: 1) The disease process is sufficiently understood; 2) The surrogate endpoint has biologic plausibility; 3) The strength of the consistency supports the relationship between the surrogate marker and outcome; 4) Treatment effects on the surrogate endpoint predict treatment effects on the clinical outcome of interest (Table 1). In addition, the acceptability of a surrogate endpoint for conditional marketing authorization of new therapies also depends on a benefit–risk evaluation and/or public health aspects, such as a serious life-threatening disease with no other therapeutic option, difficulties with studying the (rare or delayed) clinical endpoint, and the availability of a large safety database (2).
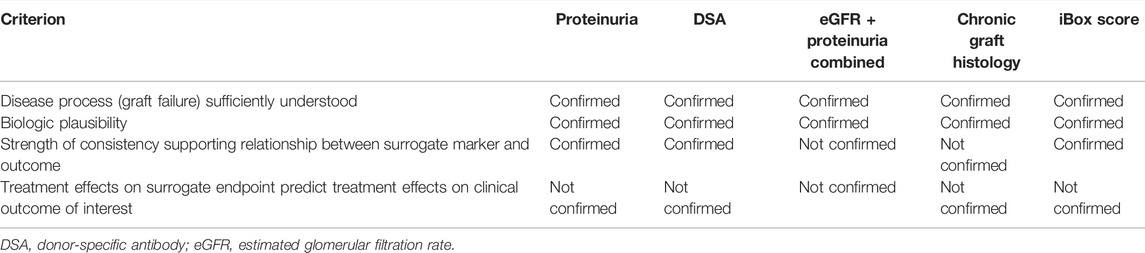
TABLE 1. Criteria for a valid surrogate endpoint, applied to potential surrogate endpoints in kidney transplantation.
Definition and Causes of Graft Failure
Graft failure/loss of graft function is defined as return to dialysis or pre-emptive re-transplantation. Death of the recipient with a functioning graft is typically a primary safety endpoint, but we do not recommend including this in a surrogate endpoint for kidney transplantation outcome because of the wide variety of underlying causes of death observed (e.g., malignancy, infection, cardiovascular disease), lack of relation to graft functional status, and very different risk factors compared with those for graft failure (3, 4). These causes of death are often influenced by immunosuppression (5).
Furthermore, death with a functioning graft is a competing risk to loss of graft function, as is also the case in chronic kidney disease (CKD). In CKD, censoring for death increasingly overestimated the risk of kidney failure over time from 7% at 5 years to 19% at 10 years, especially in people at heightened risk of death (6). Although it could be anticipated that this is also relevant in kidney transplantation, the impact of this competing risk on the accuracy of death-censored graft failure risk is poorly established.
Definitions of all-cause and overall graft failure are discussed elsewhere in this Special Issue (2); of note, in this document, “graft failure” denotes loss of graft function, not overall graft failure (which includes patient death as a reason for graft failure). Given that late graft failure (excluding death with a functioning graft) is often multifactorial (4), it is difficult to predict such failure accurately with a single marker; a composite marker may more fully reflect the heterogeneity. The most important causes of graft failure are acute or chronic T-cell mediated rejection (TCMR), antibody-mediated rejection (AMR), nonspecific chronic injury due to nephron loss (drug toxicity, metabolic and urological factors), calcineurin inhibitor toxicity, infection, and other medical events (cardiorenal problems, vascular disease, malignancy, postrenal causes) (7), as well as occurrence or recurrence of original kidney disease. Consequently, the following markers are associated with heightened risk of late graft functional decline and failure: measured glomerular filtration rate (GFR); estimated (e)GFR, slope of eGFR trajectory, and eGFR change; CKD stage; proteinuria; de novo (dn) donor-specific antibodies (DSA); AMR histology; interstitial fibrosis and tubular atrophy (IFTA); and transplant glomerulopathy (TG) (8, 9).
Single Markers as Surrogate Endpoint
Single surrogate markers of graft function may not fully reflect the complexity of graft failure and death in kidney transplantation because some background (donor or recipient) risk factors—such as age and pre-existing immunological risk, including pre-transplant DSA—also affect outcome and graft-function markers. Late graft failure is more complex than renal failure resultant from native kidney disease because of competing risks involved at different time points. For example, the ZEUS trial (phase III randomized trial of cyclosporine continuation vs. switch to everolimus at 4.5 months post-transplant) showed a slightly better GFR (the primary endpoint), but higher rates of DSA and AMR (with absence of effect on graft failure and increased risk of graft failure) in patients who developed dnDSA (7, 10). Furthermore, creating too stringent a definition of factors such as change in eGFR would require studies with long duration and large patient populations, which are difficult to achieve (11).
Conversely, considering only minor changes in a surrogate endpoint, such as eGFR or transplant glomerulopathy, increases the risk of error. For example, in histological terms, new or worsening transplant glomerulopathy could be considered as a surrogate endpoint in clinical trials, but the intrinsic heterogeneity of this pathology and varied data on its association with death-censored graft survival (12) make it difficult to translate findings into predictions for late events. In addition, this parameter has neither been used, nor accepted, by health authorities.
Combining multiple factors into a well-validated model is therefore more likely to predict long-term outcome (and better reflect the complexity of late graft failure) than using single endpoints or combining few factors. Relatively short-term improvements in such a complex score ideally would translate into long-term improvements in graft survival. It is also important that a valid surrogate marker for a well-understood disease process should have biological plausibility and a consistent relationship with outcome. Finally, treatment effects that change the surrogate marker should also have impact on clinical outcome.
Here we review the putative surrogate endpoints, including composite endpoints for predicting long-term graft outcome (excluding death with a functioning graft), focusing on eGFR, proteinuria, histological lesions, DSA, and complex scoring systems (Table 1).
GFR and eGFR
For in-depth discussion on the association between kidney function and graft failure, methodology for measuring kidney function and its validity as a primary endpoint for clinical trials, see Hilbrands et al. (13).
Because graft failure is intrinsically defined by functional parameters such as dialysis reinitiation or repeat transplantation, graft functional assessment is directly related to the true endpoint, graft failure. Any intervention that stabilizes long-term graft function will inherently decrease the incidence of graft failure, therefore graft function is a direct measure of graft failure.
Predicted graft survival based on 12-months eGFR correlates with observed graft survival (14); consequently, eGFR alone is potentially interesting as a surrogate marker for long-term graft failure. This parameter was applied in the only relatively recent organ transplantation study to show improved long-term outcome using a new treatment (belatacept) (15, 16). However, declining eGFR is a late and insensitive marker for late graft failure in heterogeneous populations (17). The initial injury processes contributing to late graft failure are subclinical, and not reflected by early decline in renal function. Consequently, the long-term predictive value of measures of early post-transplantation renal function is limited (17); such measures (including serum creatinine values and use of eGFR) are discussed elsewhere in this supplement (13).
Additional graft injuries may develop slowly over time: declining renal function is the ultimate consequence of nephron loss but does not capture causes of nephron injury. Also, compensatory hyperfiltration may obscure initial damage. Moreover, the static absolute level of eGFR is also related to donor (e.g., age, brain death, hypotension) and transplant (e.g., ischemia/reperfusion) factors that might reduce the number of functioning nephrons at transplantation; using a single eGFR measurement as a surrogate endpoint would not take these into account.
Clearly, GFR has limitations as a surrogate for late graft failure, since in the first year after transplantation it fails to capture ongoing disease processes that lead to late graft failure. Sensitive tools that better reflect the heterogeneity in causes of late graft failure are required.
Proteinuria
In CKD research there is increasing interest in using degree of proteinuria as a surrogate endpoint: the proteinuria level directly relates to the underlying glomerular disease process, and strongly correlates with progression to end-stage renal disease (18, 19). Proteinuria is routinely measured after kidney transplantation (20, 21); severe proteinuria in the nephrotic range often reflects structural damage to the nephron and is therefore associated with graft outcome (8, 22, 23). Histological signs of structural abnormality are TG, microcirculatory inflammation, and dn or recurrent glomerular disease (8), all of which are important causes of late graft failure.
Post-transplantation proteinuria thus tends to indicate poor prognosis, independent of graft function as assessed by eGFR (8, 24, 25), but may also reflect disease processes beyond renal function. Similar to general-population studies, an analysis that prospectively adjudicated cardiovascular events showed that albuminuria was strongly associated not only with graft failure, but also with cardiovascular events and mortality (25). Proteinuria alone has not widely been included as a surrogate endpoint in interventional studies of kidney transplantation and correcting post-transplantation proteinuria has not been proven to reduce the rates of long-term graft failure in studies of antihypertensive medication use in transplant populations (26–30). Conversely, studies with mTOR inhibitors revealed increases in proteinuria that did not translate into increased rates of long-term graft failure (7, 31).
Donor-Specific HLA Antibodies
Since the early days of clinical kidney transplantation, it has been recognized that antibodies directed against non-self human leukocyte antigen (HLA) could be extremely relevant for graft outcomes. A seminal study described the key features and potential impact of alloantibodies in transplantation, demonstrating that immediate catastrophic graft failure is more likely to happen in multiparous female patients or in people receiving second transplants, and is seen in up to 80% of cases where there was a “positive crossmatch” (i.e., reactivity of recipient serum against donor cells) (32). The researchers advocated that demonstration of preformed cytotoxic antibodies against the graft (“sensitization”) contraindicates allocation of a proposed graft to the transplant candidate. This recommendation was rapidly adopted and, aside from patients successfully desensitized by empirical approaches, remains rigidly enforced, using complement-dependent cytotoxicity crossmatching (CDC-XM) (32, 33).
With time, however, it became clear that CDC-XM lacks sensitivity for detecting circulating DSA: not all clinically significant pre-transplant DSA are identified. This led to the development of sensitive solid-phase tests, such as Luminex® single-antigen bead (SAB) assays (R&D Systems Inc., Minneapolis, MN, United States), which detect low-level DSA when the CDC test is negative. The definition of HLA antibody specificity by SAB assays added complexity to transplant risk stratification, by revealing extensive heterogeneity in the pathogenic potential of HLA-DSA. It is now well established that patients with pretransplant DSA detected by SAB, even with a negative CDC crossmatch, are at substantial risk of AMR and graft failure (34–37). Flow cytometry cross-matching adds additional insight into the actual immunologic risk for such patients (38).
The role of circulating anti–HLA-DSA is increasingly recognized as a major contributing factor to AMR and long-term graft failure (39–41). However, the occurrence of newly formed dnDSA after transplantation further increases the risk of graft failure (42–47), and complement-fixing DSA are particularly associated with graft rejection and failure (48). Some immunosuppressants (e.g., belatacept) appear to inhibit the development of dnHLA-DSA (16), while others (e.g., mTOR inhibitors) can be associated with a higher frequency of dnHLA-DSA (49). Importantly, under-immunosuppression and patient nonadherence are important risk factors for dnHLA-DSA development (50).
The STAR working group, a collaboration between the American Society for Histocompatibility and Immunogenetics and the American Society of Transplantation (51), made recommendations on the definitions and utilization of HLA diagnostic testing. In Europe, the European Federation for Immunogenetics publishes standards for histocompatibility and immunogenetics testing (52). Limitations of Luminex SAB assays that have been described include their semiquantitative nature, the prozone effect, test variability, and the need for arbitrary cut-off values to determine positivity. There are also technical challenges; for example, thresholds for DSA positivity are poorly defined and inconsistent, with European immunogenetics groups proposing mean fluorescence intensity (MFI) cut-off values of >3,000 or >5,000 MFI (53) and US groups proposing 1,400 MFI, which requires validation (51). A consistent definition of such a cut-off value, to indicate presence or absence of HLA antibodies, is crucial if DSA is to be considered as a single endpoint in RCTs. In addition, SAB MFI should not be used as a quantitative assay since it has a relatively high coefficient of variation (51). Thus, current technology cannot determine antibody titers or the clinical and biological relevance of positive test results (51, 54). In addition, although pretransplant DSA and dnHLA antibody development are strongly associated with AMR and graft failure (43, 55–60), no studies show that interventions affecting DSA levels or specificities after transplantation predict long-term improvement in graft survival rates (Table 2) (54, 61–63).
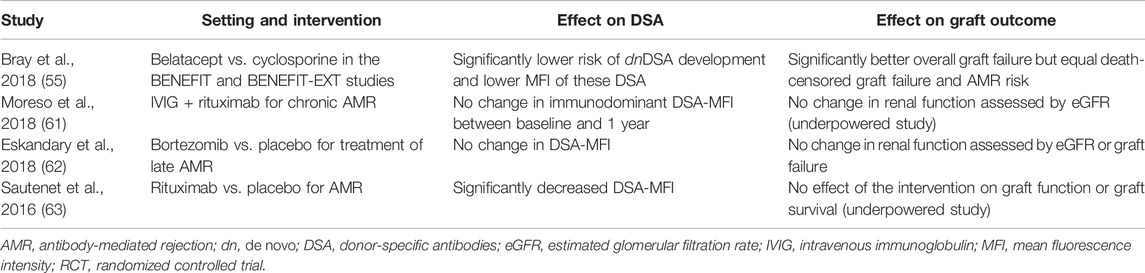
TABLE 2. Association between changes in DSA and graft outcome in kidney transplantation RCTs. No studies show that interventions that affect DSA predict long-term graft outcomes (55, 61–63).
Post hoc analyses of the BENEFIT and BENEFIT-EXT studies (phase III randomized trials of belatacept vs. cyclosporine) showed significant reductions in the risk of dnDSA occurrence (55) and best overall graft survival rates. However, numbers were too small to demonstrate that these effects were mediated through improved death-censored graft survival or decreased risk of AMR. In contrast, data from mTOR inhibitor conversion studies showed higher rates of DSA and AMR in groups treated with mTOR inhibitors, but during the observation period no overall effect on graft survival was noted (64, 65), although follow-up was short, and DSA status was often missing (65). Finally, although the RITUX ERAH RCT (randomized trial of rituximab vs. placebo in addition to plasma exchange, intravenous immunoglobulin and corticosteroids for the treatment of AMR) showed an effect of rituximab on DSA-MFI that did not translate into improved graft function or survival rate, this study was underpowered, so firm conclusions could not be made (66).
As identified in a systematic review (67), therapeutic strategies eliminating dnDSA, tested in RCTs that are sufficiently powered to assess long-term graft outcomes, are needed. Case series suggest that “impossible” transplants become possible with pre-transplant desensitization of HLA antibodies (67), but this does not validate HLA-DSA levels or specificities as surrogates for long-term outcome.
In summary, only the development of dnHLA-DSA with a clear MFI signal could be a meaningful surrogate endpoint that is strongly associated with adverse outcomes such as AMR and graft failure. While dnDSA development is clearly associated with immunosuppression, patient nonadherence (especially under-immunosuppression) may also play a role. The development of dnHLA-DSA has not been formally tested or validated as a surrogate endpoint for studies that aim to reduce graft failure because of AMR. In addition, as graft failure is heterogeneous and often no HLA-DSA are involved, dnDSA occurrence is insufficient as a surrogate for late graft failure by causes other than AMR.
Combined Functional Markers
The risk of adverse outcomes at a given eGFR certainly increases with higher levels of albuminuria. In addition, integrating proteinuria and eGFR assessment is a good predictor of graft outcome (24, 25); studies also demonstrate an independent association between graft outcome and eGFR or proteinuria (8, 68).
Although potentially interesting as surrogate marker, the performance of a model that integrates proteinuria and eGFR has not been further validated in transplantation (25). However, whether the combination of eGFR and proteinuria could be considered as a primary (rather than surrogate) endpoint in kidney transplantation, as it is in CKD, warrants further discussion. Indeed, in CKD, the KDIGO guideline on prognostication based on integration of eGFR and albuminuria is an accepted surrogate for outcome in clinical trials, but the European Medicines Agency (EMA)’s CHMP guideline for primary prevention (69) proposed two primary efficacy endpoints: prevention or slowing of decline in the level of renal function (defined as either time to occurrence of CKD 3 or incidence rate of CKD ≥3); and clinically meaningful and stable difference in GFR failure rate with or without prevention of proteinuria/albuminuria. A similar primary endpoint could be considered in kidney transplantation, and the US Food and Drug Administration already follows this approach (70). However, no RCT has been undertaken to demonstrate that changes in such a composite functional endpoint predict changes in long-term graft survival rates.
Composite Scores
Late graft failure (excluding death with a functioning graft) is a highly multifactorial state (4) that relates not only to early graft function, but also to subclinical injury processes including progressive IFTA or TG, drug toxicity, infections, medical events, recurrent disease, microvascular injury, and circulating DSA. Graft function is also highly dependent on pre-transplant donor/recipient risk factors (e.g., age, sex, delayed graft function), which further complicate the value of interpreting a single measurement of function as a surrogate for long-term outcome: studies show independent associations between these factors and graft failure in multivariate models (Table 3) (8, 9).
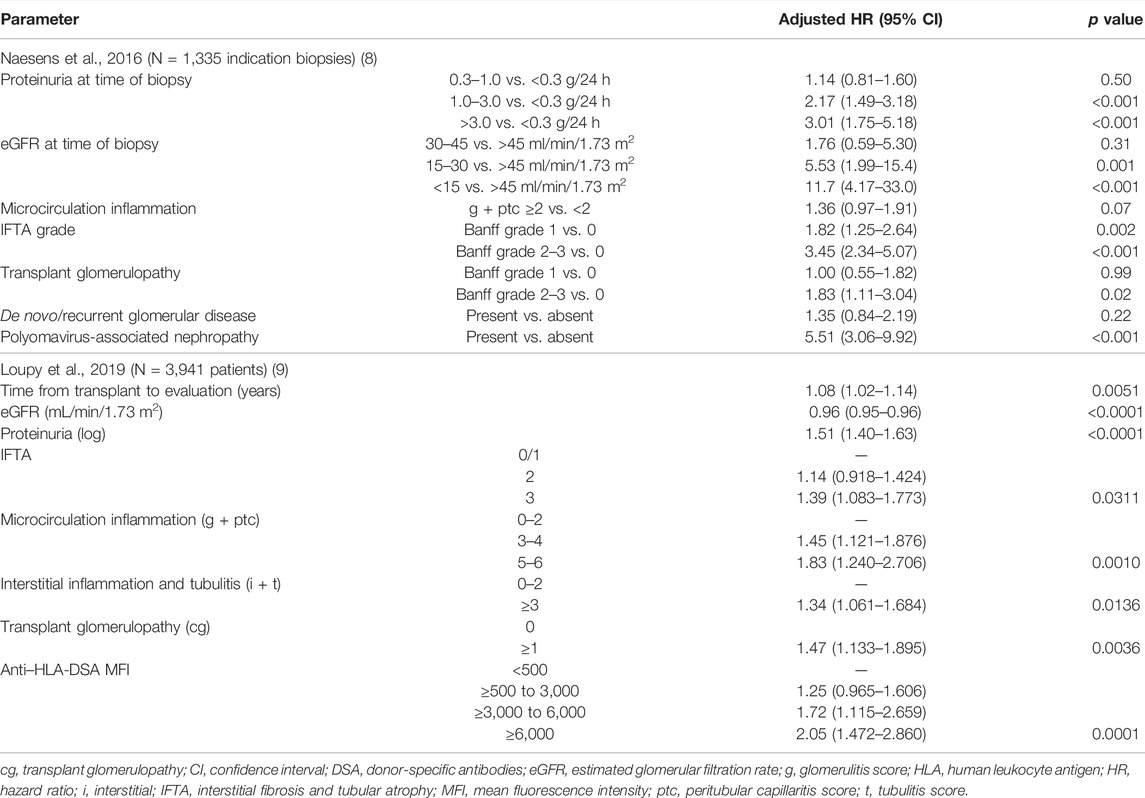
TABLE 3. HR (multivariate models) for graft failure according to graft histology, renal function, and proteinuria at time of biopsy, adjusted for donor age and time after transplantation (8,9).
A systematic review evaluated models developed to predict graft failure in kidney transplantation recipients (71). Fourteen studies used predictors that were measured after transplantation; few studies integrated graft functional data such as proteinuria (n = 5) or serum creatinine/eGFR (n = 12), and none evaluated histology as part of the composite prediction model. Nineteen studies reported on the validity of the model in external datasets, several of which warrant in-depth assessment of their potential usefulness as surrogate endpoints for long-term graft failure excluding death with a functioning graft (14, 72–77); key features of these publications are listed in Table 4. Another study suggested a composite method for predicting graft failure; but because it included recipient death, it is less appropriate than other approaches as a potential surrogate endpoint for death-censored graft failure (78, 79).
In the study by Kasiske et al. (72), eGFR at 1 year was the only functional value included in the final model for prediction of 5-years graft failure, along with baseline recipient criteria and hospitalization within the first year following transplantation. However, this analysis was performed on a large registry (USRDS) that lacked crucial information on several clinical parameters. Furthermore, although the model showed good calibration, no independent validation was performed, and the impact of therapeutic interventions that aimed to reduce long-term graft failure was not tested. Moore et al. (74) restricted post-transplant factors in the model to eGFR and eGFR evolution, but nevertheless reached adequate discrimination and calibration for death-censored graft failure. External validation was restricted to a single center, and again the impact of therapeutic interventions was not evaluated. Importantly, the risk scores derived and tested in this study offered no prognostic superiority over basic metrics, such as eGFR or recipient age in isolation (74).
Foucher et al. proposed a clinical scoring system, built on the French DIVAT registry (3). The score was constructed at 1 year post transplantation, for prediction of graft failure at 8 years, and reached a C-statistic of 0.78. External validation was performed, but in a small dataset (n = 317). Other limitations included limited exportability, restriction to French transplant centers, and no inclusion of data on DSA and rejection subtypes or histological lesions. In addition, this score was built on observations at only one time point. The potential of this prognostic score to be used as surrogacy for long-term graft failure was not tested in any RCT aiming to improve long-term outcome.
The first study to implement a previously developed risk score, in the context of a RCT aiming to improve long-term graft outcome, analyzed data from the USRDS registry (1995–2004) (14). Prediction models for all-cause graft survival were applied to participants in the BENEFIT and BENEFIT-EXT studies (phase III randomized trials of belatacept vs. cyclosporine), to determine whether the model could be used as a surrogate endpoint for late graft failure. Predicted and observed all-cause graft failures were well calibrated in standard- and expanded-criteria donor kidneys, as evaluated in the development cohort. Although data on model accuracy were lacking, aspects including eGFR and donor/recipient characteristics revealed a striking concordance between predicted and observed graft survival rates, when evaluated for 1-year outcome (14). However, when predicted survival estimates for 7 years post transplantation were compared with actual outcomes (16, 80), the predicted versus observed overall graft survival for the less-intensive group was 73.9 vs. 87.2%, and for the cyclosporine group was 69.0 vs. 78.3%. This illustrates that the calibration of the model for predicting longer-term survival was perhaps less than anticipated, which might be explained by the model being built on data obtained in an older era. As the surrogacy of the model established at 1 year for long-term graft failure was not directly confirmed, it is questionable whether it provides sufficient accuracy and calibration for use as a complex surrogate endpoint in future RCTs (14).
Shabir et al. developed a prediction model for 5-years graft failure using data from a single UK center, at 12 months post transplantation (75). The resultant risk scores were evaluated for prognostic utility (discrimination, calibration, and risk reclassification) in three independent cohorts in Europe and Canada. Recipient age, sex, and race; acute rejection rate; eGFR; serum albumin level; and urine albumin/creatinine ratio were included in scores for death-censored and overall graft failure. The rejection subtype was not further specified. In the validation cohorts, these scores showed good-to-excellent discrimination for death-censored transplant failure and moderate-to-good discrimination for overall transplant failure. Both scores demonstrated good calibration. Compared with eGFR in isolation, application of the scores resulted in statistically significant and clinically relevant risk reclassification for death-censored transplant failure [net reclassification improvement (NRI) 36.1–83.0%; all p < 0.001] and overall transplant failure (NRI 38.7–53.5%; all p < 0.001). Compared with the USRDS-based calculator, significant and relevant risk reclassification for overall transplant failure was seen (NRI 30.0%; p < 0.001) (75).
These scores have been externally validated (76): the risk model integrated 1-year histological and antibody data for prediction of graft failure at 5 years post transplantation in a single-center study (n = 1,465). The Birmingham Risk Score performed well, with good discrimination for recipients with or without graft failure 5 years after transplantation for both overall and death-censored graft failure (C-statistic 0.78 and 0.84, respectively), although this score has not been evaluated in an RCT designed to assess improvement of long-term graft outcome. Adding glomerulitis and interstitial fibrosis data to the Birmingham Risk Score improved the C-statistic for death-censored graft failure from 0.84 to 0.90, with further improved calibration and significant reclassification.
Decision-curve analyses aimed to determine how risk prediction could be improved when histological data were added to the clinical risk model proposed by Shabir et al. (75). However, this expanded model has not been independently validated and the impact of therapeutic interventions has not been evaluated. Prémaud et al. proposed a composite adjustable score for prediction of graft failure (AdGFS) using a conditional survival-tree analysis, undertaken using variables from patients transplanted between 1984 and 2011 in a French center (77). The analysis was based on serum creatinine and proteinuria at 12 months, dnDSA, serum creatinine cluster (creatinine value trajectories within the first year), acute rejection, donor age, and pre-transplant non-donor-specific HLA antibodies. Predictive performance of the AdGFS was good and the accuracy of the score at predicting graft failure remained high in the validation dataset, and in the external dataset (consisting of 896 patients from two other French centers, transplanted between 2002 and 2010). However, the study had limitations: the cohort did not represent current practice, there was no evaluation of the AdGFS response to therapies that aim to improve long-term graft outcome, validity in living donor kidney transplants and in recipients with pretransplant DSA was not tested, and data on DSA were lacking. In addition, international validation has not been performed.
iBox
Loupy et al. developed the largest and only specifically designed multivariate model that predicts long-term death-censored graft failure: the iBox model was created after a study was undertaken in which parameters were collected from day of transplantation, to provide a holistic appraisal of potential risk factors (9). Their data showed that, among 7,557 kidney transplant recipients, 1,067 grafts failed (14.12%) in a median post-transplant follow-up of 7.12 years [interquartile range (IQR) 3.51–8.77] (9). In the derivation cohort, eight functional, histological, and immunological prognostic factors were found to be independently associated with death-censored graft failure. These were then combined into a risk prediction score that included the following parameters, in order of importance: eGFR; proteinuria:creatinine ratio; structural markers [Banff IFTA grade, microcirculation inflammation (Banff g + ptc), TG (Banff cg score), interstitial inflammation, and tubulitis (Banff i + t)]; MFI of the immunodominant HLA-DSA, and time from transplant to risk evaluation. The risk prediction score exhibited accurate calibration and discrimination (0.81 derivation and 0.80–0.81 in validation cohorts) (9). The performance of this multivariate model was validated in cohorts from three European and three North American centers (9). Importantly, testing the iBox model involved unselected patient cohorts, covering all potential clinical scenarios.
The iBox model was accurate when assessed independently of time since transplant, was validated in different clinical scenarios, and outperformed a risk score based solely on eGFR, proteinuria and HLA-DSA, not including histological lesions (Table 5). The risk prediction score was also slightly superior to the conventional graft monitoring model based on eGFR and proteinuria assessments in terms of prediction capability; this was further demonstrated by a continuous NRI of 0.228 for the multivariate model compared with the functional model (95% confidence interval 0.174–0.290; p < 0.0001). In less-informed datasets, the new algorithm still performed with high accuracy (Table 5) (9).
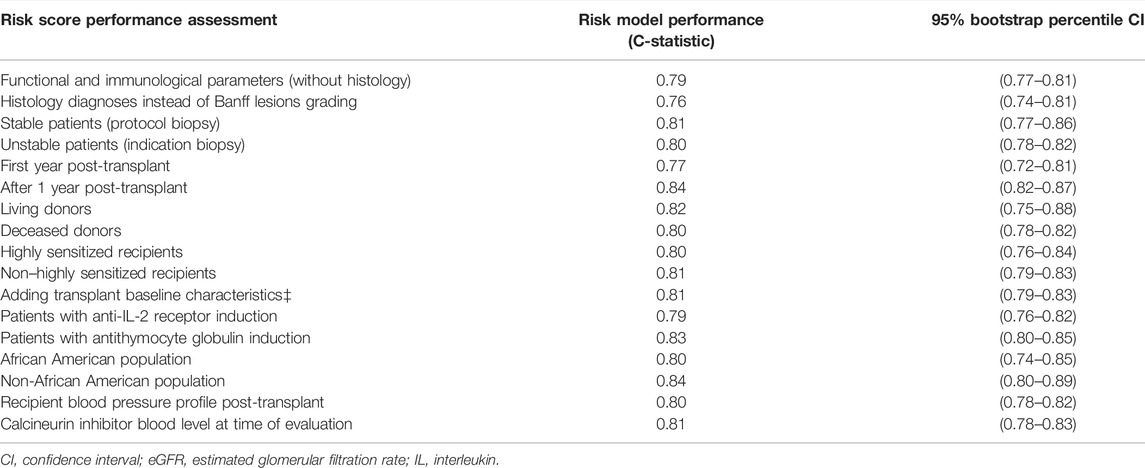
TABLE 5. Risk prediction score performance for iBox when assessed in different clinical scenarios and subpopulations (9).
The accuracy of the iBox risk score to predict long-term graft failure (9) was confirmed in post hoc analyses of data from three RCTs (Table 6) (62–64). Interventions performed in these studies affected the risk score, indicating that iBox adjusts to treatment effects. As the three RCTs did not significantly improve long-term graft outcome in the intervention group, the surrogacy of improvement of the score for predicting improvement of long-term graft survival could not be established directly. However, in the calcineurin inhibitor-free study arm of the CERTITEM study (randomized trial of switch to everolimus vs. cyclosporine continuation) there was a significantly increased risk of developing dnDSA in the everolimus group, higher rates of clinical or subclinical rejection, and worse eGFR, all of which were associated with a numerically higher risk of graft failure (5.2 vs. 1.0%). This difference in graft failure failed to reach statistical significance because of low event rates and thus lack of power (64). Post-hoc analysis of the TRANSFORM study (randomized trial of everolimus with reduced exposure calcineurin inhibitor vs. standard-exposure calcineurin inhibitor with mycophenolic acid) (81) indicated that an adapted iBox model (not all parameters were available) confirmed the noninferiority of everolimus with reduced cyclosporine vs. mycophenolic acid with standard cyclosporine for immunosuppression (82). The model projected kidney allograft survival up to 11 years postrandomization. The potential suitability of the iBox risk score as being a surrogate endpoint is further indicated by its general validity, good calibration in RCTs, adjustability over time (and in response to treatment), and its integration of risk factors that are well confirmed in the pathophysiology of (or trajectory toward) graft failure. The evolution after kidney transplantation should be considered as a multidimensional pathophysiology, which could not be identified by looking at one parameter at a time. Importantly, extensive validation through modeling different post-transplant treatment interventions appears to confirm the association between each component of the score and long-term graft failure. For example, the iBox takes account of how a drug might affect kidney function by interfering with renal haemodynamics and eGFR but reducing DSA occurrence. In the context of a clinical trial or immediate therapeutic intervention, each parameter in iBox is individually ranked in terms of the performance, discrimination, and calibration of the risk score.
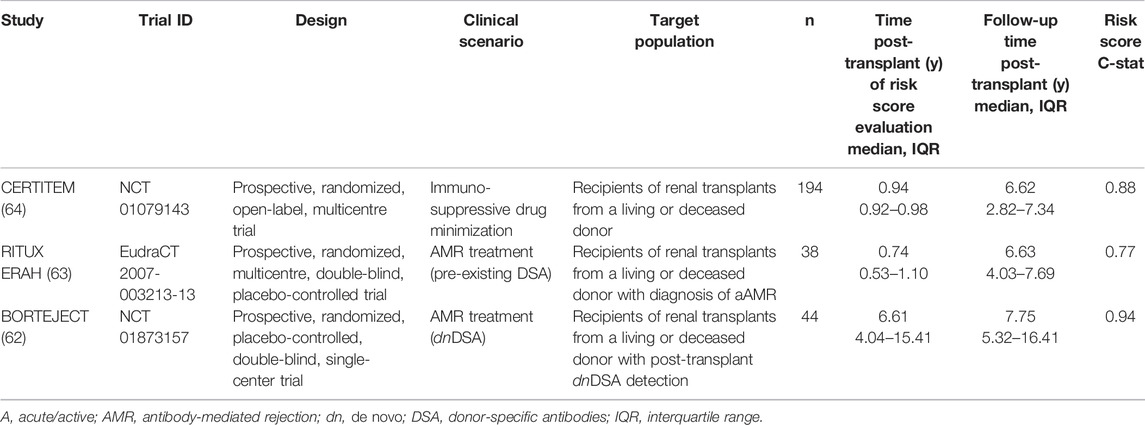
TABLE 6. Clinical trials depicting population characteristics, clinical scenarios and interventions, and prognostic performance of the iBox risk score (62–64).
Statistical methodology used in iBox was directly derived from hazard ratio in the Cox analysis; other analyses (e.g., forms of machine learning) were tested but none of the models outperformed Cox, which is widely used in clinical research. The US Food and Drug Administration (FDA) has acknowledged the iBox as a “reasonably likely surrogate endpoint” biomarker to predict 5-years risk of graft failure in kidney transplantation (83). The developers are conducting further modeling to provide additional dimensions, including options for surrogacy, evaluation of its use as an early endpoint in clinical trials, and evaluation of its prognostic ability in subgroup analyses. The developers also plan to make the iBox an open-source platform and are preparing for the 507 drug-development tool qualification process, GDPR compliance, and other aspects of cybersecurity.
Several limitations of the iBox risk score should be noted. Firstly, the method is only useful for prediction of death-censored graft failure: adding death with a functioning graft as a safety endpoint remains necessary. The decision to use the iBox score for predicting death-censored graft failure rather than overall graft failure (including death with a functioning graft) was made because recipient death and loss of graft function have very different causes (3, 4, 71, 84). All-cause graft failure is usually multifactorial and needs a specific design with transplant characteristics, donor characteristics, and factors related to recipient’s comorbidities at time of transplant and thereafter. In sensitivity analyses of the iBox study using competing risk regression models, allograft survival analyses performed in the final iBox model were not affected by competition with patient death.
Next, although the accuracy of the iBox model was maintained irrespective of whether histology was included as individual Banff lesion grades or histology diagnoses, scoring of individual histological lesions included in the composite score is hampered by reproducibility issues and interobserver variability. This limitation is relevant for any scoring system that includes histological parameters, is not specific for the iBox risk score, and needs to be addressed and mitigated in individual clinical trial designs and logistics. In addition, although the iBox score remained accurate across different centers using different methods of tissue typing and HLA antibody profiling, including the MFI of DSA means that this method is impacted by concerns relating to the absolute value of DSA-MFI, which is a semiquantitative rather than quantitative test. This must also be carefully addressed in clinical trial design.
With current evidence, we believe that the approach of multivariate models could be proposed as a surrogate marker for (death-censored) graft failure, since it considers the heterogeneity of causes of graft failure (excluding patient death with a functioning graft). Although it has not yet been shown in randomized trials that improvements in surrogate score actually predict improvements in long-term graft survival, the iBox model is the best-performing and best-validated algorithm to date (Table 6).
Conclusions
• It is difficult to promote single markers as surrogate endpoints for late graft failure:
○ GFR has limitations, since the early course of graft function fails to capture ongoing subclinical disease processes. More sensitive tools are required that reflect heterogeneity in causes of late graft failure.
○ Early proteinuria is associated with late graft failure but has not been proposed or tested as a surrogate endpoint in kidney transplantation.
○ Combining GFR and proteinuria has a better association with graft failure than either factor separately, but its potential validity as a surrogate endpoint has not been tested.
○ Development of dnHLA-DSA is associated with graft failure but has not been formally tested or validated as a surrogate endpoint in studies that aim to reduce graft failure caused by AMR. As graft failure also occurs in the absence of AMR, dnDSA occurrence is insufficient as a surrogate for late graft failure by causes other than AMR.
• AMR and TCMR are primary endpoints for kidney transplantation clinical trials, which diminishes the need to pursue their validation as surrogate endpoints for late graft failure.
• Death of the recipient with a functioning graft is typically a primary safety endpoint:
○ Death of the recipient with a functioning graft is a competing risk for graft failure, but the impact of this competing risk on the accuracy of predictive models is poorly described.
○ We recommend not to include recipient death in a surrogate endpoint for late graft failure because of the wide variety of underlying causes of a death observed, different to the causes of graft failure.
• Several composite scores have been proposed and could be useful surrogate endpoints for interventional studies evaluating late graft failure.
○ The iBox model is already a well-validated composite score that illustrates the robustness of this integrative approach, although further evaluations are in progress.
Scientific Advice From the Commitee for Medicinal Products (CHMP) of the European Medicines Agency (EMA) for Human Use Regarding These Conclusions
• The CHMP acknowledged that the ESOT proposes to combine several factors into a single well-validated model as a surrogate endpoint to predict long-term outcome. A surrogate would be expected to fulfill the following three criteria:
○ Show biological relationship to the clinical outcome.
○ Demonstrate, in epidemiological studies, prognostic value of the surrogate for clinical outcome.
○ Provide evidence from clinical trial settings that treatment effect on the surrogate corresponds to effect on clinical outcome.
• The CHMP noted that ESOT introduces the iBox model (9) to predict long-term kidney graft failure at 3, 5, and 7 years, based on the following factors:
○ Time from transplant to risk evaluation after transplantation.
○ eGFR.
○ Proteinuria.
○ Banff IFTA grade, g + ptc, cg, and i + t scores.
○ MFI of donor-specific HLA antibodies.
• Based on ESOT´s position and the publication by Loupy et al. (9), the score appears to be designed as a risk calculation score and validated as such in separate cohorts. As such, the iBox score could provide an important contribution to the stratification of participants of clinical trials of transplantation.
• It is not clear if the third criterion above has been fulfilled, i.e., that treatment effect measured via iBox translates into corresponding effect on clinical outcome, i.e., graft failure. Furthermore, the following issues need to be addressed:
○ The statistical model and iBox algorithm were not presented and the relative contribution of each factor of the model was not evident; several factors of the iBox are also interrelated, e.g., histological diagnosis and the various histological lesions.
○ “Time from transplant” is an important prognostic marker but is never affected by therapy, therefore it cannot predict the effect of therapy on clinical outcome.
○ Outcome of iBox included death-censored graft failure, which is not a robust and favored clinical endpoint to show surrogacy, as there are several limitations in using the score without additional sensitivity analyses.
• ESOT showed the correlation of each variable in the final iBox model to death-censored functional outcome, a density plot of iBox evaluations post transplantation and the hazard ratio of each factor of the model.
○ Sensitivity analysis of the iBox indicate that graft survival analysis was not affected by competition with patient death.
○ ESOT noted that all-cause graft failure was multifactorial, with very different risk factors than death-censored graft failure, where grafts from patients who died with a functioning graft, were defined as functional grafts in the model.
○ However, ESOT acknowledged the concern regarding the importance of all-cause mortality in clinical trials of kidney transplantation for regulatory purposes and proposed to include this as part of safety or composite endpoints.
○ ESOT outlined the plans to further explore these issues with the FDA, including the preparation for a Drug Development Tool (DDT) qualification process.
• For the time being iBox is not qualified as a surrogate endpoint for regulatory purposes and thus cannot be proposed a priori to be used in clinical practice to guide decision making.
○ Based on the high-level data provided, CHMP notes that there are still certain limitations in applying the iBox score for regulatory purposes: the applicability of this score seems limited to certain determinants of kidney graft and the death-censored functional aspect.
○ A formal EMA Qualification of Novel Methodologies procedure for the finalized iBox as a surrogate marker would be very relevant way forward and is recommended.
Author Contributions
All authors listed have made a substantial, direct, and intellectual contribution to the work and approved it for publication. This article is one of a series of papers developed from the Broad Scientific Advice request, submitted to the European Medicines Agency (EMA) Committee for Medicinal Products for Human Use (CHMP) by the European Society for Organ Transplantation (ESOT) in 2020: interactions between the EMA and ESOT regarding this request began in 2016. For the present article, through virtual and face-to-face discussions, the working group on surrogate endpoints in kidney transplantation developed the ESOT position on the core question ‘Does CHMP agree that long-term outcome after kidney transplantation is an area of unmet medical need, for which conditional marketing authorization procedures should be considered, to facilitate timely access to new therapies? If so, does CHMP agree with the proposed surrogate endpoints for clinical trials for therapies requiring conditional marketing authorization?' The Centre for Evidence in Transplantation provided support with specific data extraction requests: these literature searches formed the basis of evidence used in the Broad Scientific Advice request and the present article. Input into the working group’s output was provided from all ESOT members involved in the Broad Scientific Advice request process. The present article was adapted by MN from the final Broad Scientific Advice request submission (June 2020), presentation documents and minutes of the meeting between ESOT and the CHMP Scientific Advice Working Party (SAWP) (September 2020), and the final response from the SAWP (December 2020). All drafts of the article were circulated to all co-authors for review and approval before submission for publication.
Funding
This initiative was supported by the European Society for Organ Transplantation.
Conflict of Interest
KB has received honoraria and/or research funding from Alexion, Astellas, Bristol Myers Squibb, Chiesi, Fresenius, Hansa, Hexal, Merck, Novartis, Otsuka, Pfizer, Roche, Sandoz, Siemens, and Veloxis. LH reports speaker fees from Astellas, consultancy and research support from Chiesi, consultancy for Novartis, and research support from Sandoz. RO has received grants/research support from Amgen, Astellas, and Chiesi; and speakers’ bureaux/honoraria from Amgen, Astellas, Chiesi, Hansa, Neovii, Novartis, and Teva. DG reports consultancy agreements and scientific boards for AstraZeneca, BMS, Hansa, and Sanofi. UH has received grants/research support from Baxter, Chiesi, and Neovii; speakers’ bureaux/honoraria from Chiesi and Hansa; and consulting fees from Astellas, Hansa, Neovii, Novartis, and Teva. IJ’s institution has received speaker’s fees from XVIVO Perfusion. MR has received lecture fees from Astellas and Chiesi; and research grant support (paid to institution) from Astellas and Chiesi for investigator-initiated studies. SS has received grants/research support from Bridge to Life, Chiesi, Neovii, Novartis, Organ Recovery Systems, and Sandoz; speakers’ bureaux/honoraria from Astellas, BMS, Chiesi, Novartis, OrganOx, and Sanofi; and consulting fees from Astellas, Atara, Merck, NefroHealth, Novartis, Sandoz, and Teva.
The remaining authors declare that the work was conducted in the absence of any commercial or financial relationships that could be construed as a potential conflict of interest.
Acknowledgments
The authors thank the experts involved with the European Medicines Agency (EMA) Committee for Medicinal Products for Human Use (CHMP) who participated in the Broad Scientific Advice request. The published information is based on EMA feedback received during the Broad Scientific Advice request. EMA/CHMP have not been involved in the drafting or review of the manuscript to be published. This publication does not constitute a formal EMA/CHMP endorsement of the manuscript. Medical writing support was provided by Linda Edmondson, independent medical writer, funded by ESOT.
References
1. Sautenet, B, TongChapman, AJR, Chapman, JR, Warrens, AN, Rosenbloom, D, Wong, G, et al. Range and Consistency of Outcomes Reported in Randomized Trials Conducted in Kidney Transplant Recipients: a Systematic Review. Transplantation (2018) 102:2065–71. doi:10.1097/tp.0000000000002278
2. Naesens, M, Loupy, A, Hilbrands, L, Oberbauer, R, Bellini, MI, Glotz, D, et al. Rationale for Surrogate Endpoints and Conditional Marketing Authorization of New Therapies for Kidney Transplantation. Transpl Int (2022). 35:10137. doi:10.3389/ti.2022.10137
3. Van Loon, E, Senev, A, Lerut, E, Coemans, M, Callemeyn, J, Van Keer, JM, et al. Assessing the Complex Causes of Kidney Allograft Loss. Transplantation (2020) 104:2557–66. doi:10.1097/tp.0000000000003192
4. Mayrdorfer, M, Liefeldt, L, Wu, K, Rudolph, B, Zhang, Q, Friedersdorff, F, et al. Exploring the Complexity of Death-Censored Kidney Allograft Failure. J Am Soc Nephrol (2021) 32:1513–26. doi:10.1681/asn.2020081215
5. Opelz, G, and Döhler, B. Association between Steroid Dosage and Death with a Functioning Graft after Kidney Transplantation. Am J Transplant (2013) 13:2096–105. doi:10.1111/ajt.12313
6. Ravani, P, Fiocco, M, Liu, P, Quinn, RR, Hemmelgarn, B, James, M, et al. Influence of Mortality on Estimating the Risk of Kidney Failure in People with Stage 4 CKD. J Am Soc Nephrol (2019) 30:2219–27. doi:10.1681/asn.2019060640
7. Budde, K, Lehner, F, Sommerer, C, Reinke, P, Arns, W, Eisenberger, U, et al. Five-year Outcomes in Kidney Transplant Patients Converted from Cyclosporine to Everolimus: the Randomized ZEUS Study. Am J Transplant (2014) 15:119–28. doi:10.1111/ajt.12952
8. Naesens, M, Lerut, E, Emonds, M-P, Herelixka, A, Evenepoel, P, Claes, K, et al. Proteinuria as a Noninvasive Marker for Renal Allograft Histology and Failure: an Observational Cohort Study. J Am Soc Nephrol (2016) 27:281–92. doi:10.1681/asn.2015010062
9. Loupy, A, Aubert, O, Orandi, BJ, Naesens, M, Bouatou, Y, Raynaud, M, et al. Prediction System for Risk of Allograft Loss in Patients Receiving Kidney Transplants: International Derivation and Validation Study. BMJ (2019) 366:l4923. doi:10.1136/bmj.l4923
10. OʼConnell, PJ, Kuypers, DR, Mannon, RB, Abecassis, M, Chadban, SJ, Gill, JS, et al. Clinical Trials for Immunosuppression in Transplantation: the Case for Reform and Change in Direction. Transplantation (2017) 101:1527–34. doi:10.1097/TP.0000000000001648
11. Stegall, MD, Morris, RE, Alloway, RR, and Mannon, RB. Developing New Immunosuppression for the Next Generation of Transplant Recipients: the Path Forward. Am J Transplant (2016) 16:1094–101. doi:10.1111/ajt.13582
12. Aubert, O, Higgins, S, Bouatou, Y, Yoo, D, Raynaud, M, Viglietti, D, et al. Archetype Analysis Identifies Distinct Profiles in Renal Transplant Recipients with Transplant Glomerulopathy Associated with Allograft Survival. J Am Soc Nephrol (2019) 30:625–39. doi:10.1681/asn.2018070777
13. Hilbrands, L, Budde, K, Bellini, MI, Diekmann, F, Furian, L, Grinyo, J, et al. Allograft Function as Endpoint for Clinical Trials in Kidney Transplantation. Transpl Int (2022) 35:10139. doi:10.3389/ti.2022.10139
14. Schnitzler, MA, Lentine, KL, Axelrod, D, Gheorghian, A, You, M, Kalsekar, A, et al. Use of 12-Month Renal Function and Baseline Clinical Factors to Predict Long-Term Graft Survival. Transplantation (2012) 93:172–81. doi:10.1097/tp.0b013e31823ec02a
15. Larsen, CP, Grinyó, J, Medina-Pestana, J, Vanrenterghem, Y, Vincenti, F, Breshahan, B, et al. Belatacept-based Regimens versus a Cyclosporine A-Based Regimen in Kidney Transplant Recipients: 2-year Results from the BENEFIT and BENEFIT-EXT Studies. Transplantation (2010) 90:1528–35. doi:10.1097/tp.0b013e3181ff87cd
16. Vincenti, F, Rostaing, L, Grinyo, J, Rice, K, Steinberg, S, Gaite, L, et al. Belatacept and Long-Term Outcomes in Kidney Transplantation. N Engl J Med (2016) 374:333–43. doi:10.1056/nejmoa1506027
17. Kaplan, B, Schold and, J, and Meier-Kriesche, H-U. Poor Predictive Value of Serum Creatinine for Renal Allograft Loss. Am J Transplant (2003) 3:1560–5. doi:10.1046/j.1600-6135.2003.00275.x
18. Cravedi, P, Ruggenenti, P, and Remuzzi, G. Proteinuria Should Be Used as a Surrogate in CKD. Nat Rev Nephrol (2012) 8:301–6. doi:10.1038/nrneph.2012.42
19. Avasare, RS, and Radhakrishnan, J. Proteinuria as a Surrogate Marker for Renal Outcome: Are We There yet? Kidney Int (2015) 88:1228–30. doi:10.1038/ki.2015.301
20. Nankivell, BJ, and Kuypers, DR. Diagnosis and Prevention of Chronic Kidney Allograft Loss. Lancet (2011) 378:1428–37. doi:10.1016/s0140-6736(11)60699-5
21. Legendre, C, and Anglicheau, D. Proteinuria in Kidney Transplantation: an Ongoing story. Nat Rev Nephrol (2013) 9:251–2. doi:10.1038/nrneph.2013.61
22. Amer, H, Fidler, ME, Myslak, M, Morales, P, Kremers, WK, Larson, TS, et al. Proteinuria after Kidney Transplantation, Relationship to Allograft Histology and Survival. Am J Transplant (2007) 7:2748–56. doi:10.1111/j.1600-6143.2007.02006.x
23. Halimi, J-M, Buchler, M, Al-Najjar, A, Laouad, I, Chatelet, V, Marlière, J-F, et al. Urinary Albumin Excretion and the Risk of Graft Loss and Death in Proteinuric and Non-proteinuric Renal Transplant Recipients. Am J Transplant (2007) 7:618–25. doi:10.1111/j.1600-6143.2007.01665.x
24. Lam, NN, Tonelli, M, Lentine, KL, Hemmelgarn, B, Ye, F, Wen, K, et al. Albuminuria and Posttransplant Chronic Kidney Disease Stage Predict Transplant Outcomes. Kidney Int (2017) 92:470–8. doi:10.1016/j.kint.2017.01.028
25. Weiner, DE, Park, M, Tighiouart, H, Joseph, AA, Carpenter, MA, Goyal, N, et al. Albuminuria and Allograft Failure, Cardiovascular Disease Events, and All-Cause Death in Stable Kidney Transplant Recipients: a Cohort Analysis of the FAVORIT Trial. Am J Kidney Dis (2019) 73:51–61. doi:10.1053/j.ajkd.2018.05.015
26. Opelz, G, Zeier, M, Laux, G, Morath, C, and Döhler, B. No Improvement of Patient or Graft Survival in Transplant Recipients Treated with Angiotensin-Converting Enzyme Inhibitors or Angiotensin II Type 1 Receptor Blockers: a Collaborative Transplant Study Report. J Am Soc Nephrol (2006) 17:3257–62. doi:10.1681/asn.2006050543
27. Philipp, T, Martinez, F, Geiger, H, Moulin, B, Mourad, G, Schmieder, R, et al. Candesartan Improves Blood Pressure Control and Reduces Proteinuria in Renal Transplant Recipients: Results from SECRET. Nephrol Dial Transplant (2010) 25:967–76. doi:10.1093/ndt/gfp581
28. Mandelbrot, DA, Alberú, J, Barama, A, Marder, BA, Silva, HT, Flechner, SM, et al. Effect of Ramipril on Urinary Protein Excretion in Maintenance Renal Transplant Patients Converted to Sirolimus. Am J Transplant (2015) 15:3174–84. doi:10.1111/ajt.13384
29. Knoll, GA, Fergusson, D, Chassé, M, Hebert, P, Wells, G, Tibbles, LA, et al. Ramipril versus Placebo in Kidney Transplant Patients with Proteinuria: a Multicentre, Double-Blind, Randomised Controlled Trial. Lancet Diabetes Endocrinol (2016) 4:318–26. doi:10.1016/s2213-8587(15)00368-x
30. Hiremath, S, Fergusson, DA, Fergusson, N, Bennett, A, and Knoll, GA. Renin-angiotensin System Blockade and Long-Term Clinical Outcomes in Kidney Transplant Recipients: a Meta-Analysis of Randomized Controlled Trials. Am J Kidney Dis (2017) 69:78–86. doi:10.1053/j.ajkd.2016.08.018
31. Budde, K, Becker, T, Arns, W, Sommerer, C, Reinke, P, Eisenberger, U, et al. Everolimus-based, Calcineurin-inhibitor-free Regimen in Recipients of De-novo Kidney Transplants: an Open-Label, Randomised, Controlled Trial. Lancet (2011) 377:837–47. doi:10.1016/s0140-6736(10)62318-5
32. Patel, R, and Terasaki, PI. Significance of the Positive Crossmatch Test in Kidney Transplantation. N Engl J Med (1969) 280:735–9. doi:10.1056/nejm196904032801401
33. Gebel, HM, Bray, RA, and Nickerson, P. Pre-transplant Assessment of Donor-Reactive, HLA-specific Antibodies in Renal Transplantation: Contraindication vs. Risk. Am J Transplant (2003) 3:1488–500. doi:10.1046/j.1600-6135.2003.00273.x
34. Ziemann, M, Altermann, W, Angert, K, Arns, W, Bachmann, A, Bakchoul, T, et al. Preformed Donor-specific HLA Antibodies in Living and Deceased Donor Transplantation. Clin J Am Soc Nephrol (2019) 14:1056–66. doi:10.2215/cjn.13401118
35. Michielsen, LA, Wisse, BW, Kamburova, EG, Verhaar, MC, Joosten, I, Allebes, WA, et al. A Paired Kidney Analysis on the Impact of Pre-transplant Anti-HLA Antibodies on Graft Survival. Nephrol Dial Transplant (2019) 34:1056–63. doi:10.1093/ndt/gfy316
36. Mohan, S, Palanisamy, A, Tsapepas, D, Tanriover, B, Crew, RJ, Dube, G, et al. Donor-specific Antibodies Adversely Affect Kidney Allograft Outcomes. J Am Soc Nephrol (2012) 23:2061–71. doi:10.1681/asn.2012070664
37. Zecher, D, Bach, C, Staudner, C, Böger, CA, Bergler, T, Banas, B, et al. Characteristics of Donor-specific Anti-HLA Antibodies and Outcome in Renal Transplant Patients Treated with a Standardized Induction Regimen. Nephrol Dial Transplant (2017) 32:730–7. doi:10.1093/ndt/gfw445
38. Konvalinka, A, and Tinckam, K. Utility of HLA Antibody Testing in Kidney Transplantation. J Am Soc Nephrol (2015) 26:1489–502. doi:10.1681/asn.2014080837
39. Nankivell, BJ, and Alexander, SI. Rejection of the Kidney Allograft. N Engl J Med (2010) 363:1451–62. doi:10.1056/nejmra0902927
40. Lefaucheur, C, Loupy, A, Hill, GS, Andrade, J, Nochy, D, Antoine, C, et al. Preexisting Donor-specific HLA Antibodies Predict Outcome in Kidney Transplantation. J Am Soc Nephrol (2010) 21:1398–406. doi:10.1681/asn.2009101065
41. Senev, A, Coemans, M, Lerut, E, Van Sandt, V, Daniëls, L, Kuypers, D, et al. Histological Picture of Antibody‐mediated Rejection without Donor‐specific anti‐HLA Antibodies: Clinical Presentation and Implications for Outcome. Am J Transplant (2019) 19:763–80. doi:10.1111/ajt.15074
42. Wiebe, C, Gibson, IW, Blydt-Hansen, TD, Karpinski, M, Ho, J, Storsley, LJ, et al. Evolution and Clinical Pathologic Correlations of De Novo Donor-specific HLA Antibody post Kidney Transplant. Am J Transplant (2012) 12:1157–67. doi:10.1111/j.1600-6143.2012.04013.x
43. Aubert, O, Loupy, A, Hidalgo, L, Duong van Huyen, J-P, Higgins, S, Viglietti, D, et al. Antibody-Mediated Rejection Due to Preexisting versusDe NovoDonor-specific Antibodies in Kidney Allograft Recipients. J Am Soc Nephrol (2017) 28:1912–23. doi:10.1681/asn.2016070797
44. Cooper, JE, Gralla, J, Cagle, L, Goldberg, R, Chan, L, and Wiseman, AC. Inferior Kidney Allograft Outcomes in Patients with De Novo Donor-specific Antibodies Are Due to Acute Rejection Episodes. Transplantation (2011) 91:1103–9. doi:10.1097/tp.0b013e3182139da1
45. Ginevri, F, Nocera, A, Comoli, P, Innocente, A, Cioni, M, Parodi, A, et al. PosttransplantDe NovoDonor-specific HLA Antibodies Identify Pediatric Kidney Recipients at Risk for Late Antibody-Mediated Rejection. Am J Transplant (2012) 12:3355–62. doi:10.1111/j.1600-6143.2012.04251.x
46. Zhang, R. Donor-specific Antibodies in Kidney Transplant Recipients. Clin J Am Soc Nephrol (2018) 13:182–92. doi:10.2215/cjn.00700117
47. Mannon, RB, Askar, M, Jackson, AM, Newell, K, and Mengel, M. Meeting Report of the STAR-Sensitization in Transplantation Assessment of Risk: Naïve Abdominal Transplant Organ Subgroup Focus on Kidney Transplantation. Am J Transplant (2018) 18:2120–34. doi:10.1111/ajt.14977
48. Bouquegneau, A, Loheac, C, Aubert, O, Bouatou, Y, Viglietti, D, Empana, JP, et al. Complement-activating Donor-specific Anti-HLA Antibodies and Solid Organ Transplant Survival: A Systematic Review and Meta-Analysis. PLoS (2018) 15:e1002572. doi:10.1371/journal.pmed.1002572
49. Grimbert, P, and Thaunat, O. mTOR Inhibitors and Risk of Chronic Antibody-Mediated Rejection after Kidney Transplantation: where Are We Now? Transpl Int (2017) 30:647–57. doi:10.1111/tri.12975
50. Gokoel, SRM, Gombert-Handoko, KB, Zwart, TC, van der Boog, PJM, Moes, DJAR, and de Fijter, JW. Medication Non-adherence after Kidney Transplantation: A Critical Appraisal and Systematic Review. Transplant Rev (2020) 34:100511. doi:10.1016/j.trre.2019.100511
51. Tambur, AR, and Wiebe, C. HLA Diagnostics. Transplantation (2018) 102:S23–S30. doi:10.1097/tp.0000000000001817
52.European Federation of Immunogenetics. EFI Standards 2019/2020 (2019). Available at: https://efi-web.org/committees/standards-committee (Accessed January 14, 2022).
53. Süsal, C, Seidl, C, Schönemann, C, Heinemann, FM, Kauke, T, Gombos, P, et al. Determination of Unacceptable HLA Antigen Mismatches in Kidney Transplant Recipients: Recommendations of the German Society for Immunogenetics. Tissue Antigens (2015) 86:317–23. doi:10.1111/tan.12682
54. Karahan, GE, Claas, FHJ, and Heidt, S. Technical Challenges and Clinical Relevance of Single Antigen Bead C1q/C3d Testing and IgG Subclass Analysis of Human Leukocyte Antigen Antibodies. Transpl Int (2018) 31:1189–97. doi:10.1111/tri.13327
55. Bray, RA, Gebel, HM, Townsend, R, Roberts, ME, Polinsky, M, Yang, L, et al. De Novo donor-specific Antibodies in Belatacept-Treated vs Cyclosporine-Treated Kidney-Transplant Recipients: Post Hoc Analyses of the Randomized Phase III BENEFIT and BENEFIT-EXT Studies. Am J Transplant (2018) 18:1783–9. doi:10.1111/ajt.14721
56. Wiebe, C, Gibson, IW, Blydt-Hansen, TD, Pochinco, D, Birk, PE, Ho, J, et al. Rates and Determinants of Progression to Graft Failure in Kidney Allograft Recipients WithDe NovoDonor-specific Antibody. Am J Transplant (2015) 15:2921–30. doi:10.1111/ajt.13347
57. Wiebe, C, Rush, DN, Nevins, TE, Birk, PE, Blydt-Hansen, T, Gibson, IW, et al. Class II Eplet Mismatch Modulates Tacrolimus Trough Levels Required to Prevent Donor-specific Antibody Development. J Am Soc Nephrol (2017) 28:335–62. doi:10.1681/asn.2017030287
58. Lachmann, N, Terasaki, PI, Budde, K, Liefeldt, L, Kahl, A, Reinke, P, et al. Anti-human Leukocyte Antigen and Donor-specific Antibodies Detected by Luminex Posttransplant Serve as Biomarkers for Chronic Rejection of Renal Allografts. Transplantation (2009) 87:1505–13. doi:10.1097/tp.0b013e3181a44206
59. Lachmann, N, Niemann, M, Reinke, P, Budde, K, Schmidt, D, Halleck, F, et al. Donor-Recipient Matching Based on Predicted Indirectly Recognizable HLA Epitopes Independently Predicts the Incidence ofDe NovoDonor-specific HLA Antibodies Following Renal Transplantation. Am J Transplant (2017) 17:3076–86. doi:10.1111/ajt.14393
60. Liefeldt, L, and Budde, K. Risk Factors for Cardiovascular Disease in Renal Transplant Recipients and Strategies to Minimize Risk. Transpl Int (2010) 23:1191–204. doi:10.1111/j.1432-2277.2010.01159.x
61. Moreso, F, Crespo, M, Ruiz, JC, Torres, A, Gutierrez-Dalmau, A, Osuna, A, et al. Treatment of Chronic Antibody Mediated Rejection with Intravenous Immunoglobulins and Rituximab: A Multicenter, Prospective, Randomized, Double-Blind Clinical Trial. Am J Transplant (2018) 18:927–35. doi:10.1111/ajt.14520
62. Eskandary, F, Regele, H, Baumann, L, Bond, G, Kozakowski, N, Wahrmann, M, et al. A Randomized Trial of Bortezomib in Late Antibody-Mediated Kidney Transplant Rejection. J Am Soc Nephrol (2018) 29:591–605. doi:10.1681/asn.2017070818
63. Sautenet, B, Blancho, G, Büchler, M, Morelon, E, Toupance, O, Barrou, B, et al. One-year Results of the Effects of Rituximab on Acute Antibody-Mediated Rejection in Renal Transplantation. Transplantation (2016) 100:391–9. doi:10.1097/tp.0000000000000958
64. Rostaing, L, Hertig, A, Albano, L, Anglicheau, D, Durrbach, A, Vuiblet, V, et al. Fibrosis Progression According to Epithelial-Mesenchymal Transition Profile: a Randomized Trial of Everolimus versus CsA. Am J Transplant (2015) 15:1303–12. doi:10.1111/ajt.13132
65. De Fijter, JW, Holdaas, H, Øyen, O, Sanders, J-S, Sundar, S, Bemelman, FJ, et al. Early Conversion from Calcineurin Inhibitor- to Everolimus-Based Therapy Following Kidney Transplantation: Results of the Randomized ELEVATE Trial. Am J Transplant (2017) 17:1853–67. doi:10.1111/ajt.14186
66. Bailly, E, Ville, S, Blancho, G, Morelon, E, Bamoulid, J, Caillard, S, et al. An Extension of the RITUX‐ERAH Study, Multicenter Randomized Clinical Trial Comparing Rituximab to Placebo in Acute Antibody‐mediated Rejection after Renal Transplantation. Transpl Int (2020) 33:786–95. doi:10.1111/tri.13613
67. Sharma, A, Lewis, JR, Lim, WH, Palmer, S, Strippoli, G, Chapman, JR, et al. Renal Transplant Outcomes and De Novo Donor-specific Anti-human Leukocyte Antigen Antibodies: a Systematic Review. Nephrol Dial Transplant (2018) 33:1472–80. doi:10.1093/ndt/gfy077
68. Loupy, A, Vernerey, D, Tinel, C, Aubert, O, Duong van Huyen, J-P, Rabant, M, et al. Subclinical Rejection Phenotypes at 1 Year post-transplant and Outcome of Kidney Allografts. J Am Soc Nephrol (2015) 26:1721–31. doi:10.1681/asn.2014040399
69.European Medicines Agency/CHMP/500825/2016. Guideline on the Clinical Investigation of Medicinal Products to Prevent Development/slow Progression of Chronic Renal Insufficiency. Available at https://www.ema.europa.eu/en/documents/scientific-guideline/guideline-clinical-investigation-medicinal-products-prevent-development/slow-progression-chronic-renal-insufficiency_en.pdf (Accessed December 20, 2021).
70. Levey, AS, Inker, LA, Matsushita, K, Greene, T, Willis, K, Lewis, E, et al. GFR Decline as an End point for Clinical Trials in CKD: a Scientific Workshop Sponsored by the National Kidney Foundation and the US Food and Drug Administration. Am J Kidney Dis (2014) 64:821–35. doi:10.1053/j.ajkd.2014.07.030
71. Kaboré, R, Haller, MC, Harambat, J, Heinze, G, and Leffondré, K. Risk Prediction Models for Graft Failure in Kidney Transplantation: a Systematic Review. Nephrol Dial Transplant (2017) 32:ii68–ii76. doi:10.1093/ndt/gfw405
72. Kasiske, BL, Israni, AK, Snyder, JJ, Skeans, MA, Peng, Y, and Weinhandl, ED. A Simple Tool to Predict Outcomes after Kidney Transplant. Am J Kidney Dis (2010) 56:947–60. doi:10.1053/j.ajkd.2010.06.020
73. Foucher, Y, Daguin, P, Akl, A, Kessler, M, Ladrière, M, Legendre, C, et al. A Clinical Scoring System Highly Predictive of Long-Term Kidney Graft Survival. Kidney Int (2010) 78:1288–94. doi:10.1038/ki.2010.232
74. Moore, J, He, X, Shabir, S, Hanvesakul, R, Benavente, D, Cockwell, P, et al. Development and Evaluation of a Composite Risk Score to Predict Kidney Transplant Failure. Am J Kidney Dis (2011) 57:744–51. doi:10.1053/j.ajkd.2010.12.017
75. Shabir, S, Halimi, J-M, Cherukuri, A, Ball, S, Ferro, C, Lipkin, G, et al. Predicting 5-year Risk of Kidney Transplant Failure: a Prediction Instrument Using Data Available at 1 Year Posttransplantation. Am J Kidney Dis (2014) 63:643–51. doi:10.1053/j.ajkd.2013.10.059
76. Gonzales, MM, Bentall, A, Kremers, WK, Stegall, MD, and Borrows, R. Predicting Individual Renal Allograft Outcomes Using Risk Models with 1-year Surveillance Biopsy and Alloantibody Data. J Am Soc Nephrol (2016) 27:3165–74. doi:10.1681/asn.2015070811
77. Prémaud, A, Filloux, M, Gatault, P, Thierry, A, Büchler, M, Munteanu, E, et al. An Adjustable Predictive Score of Graft Survival in Kidney Transplant Patients and the Levels of Risk Linked to De Novo Donor-specific Anti-HLA Antibodies. PLoS One (2017) 12:e0180236. doi:10.1371/journal.pone.0180236
78. Srinivas, TR, Taber, DJ, Su, Z, Zhang, J, Mour, G, Northrup, D, et al. Big Data, Predictive Analytics, and Quality Improvement in Kidney Transplantation: a Proof of Concept. Am J Transplant (2017) 17:671–81. doi:10.1111/ajt.14099
79. DuBay, DA, Su, Z, Morinelli, TA, Baliga, P, Rohan, V, Bian, J, et al. Development and Future Deployment of a 5 Years Allograft Survival Model for Kidney Transplantation. Nephrology (2019) 24:855–62. doi:10.1111/nep.13488
80. Vincenti, F, Blancho, G, Durrbach, A, Grannas, G, Grinyó, J, Meier-Kriesche, H-U, et al. Ten-year Outcomes in a Randomized Phase II Study of Kidney Transplant Recipients Administered Belatacept 4-weekly or 8-weekly. Am J Transplant (2017) 17:3219–27. doi:10.1111/ajt.14452
81. Pascual, J, Berger, SP, Witzke, O, Tedesco, H, Mulgaonkar, S, Qazi, Y, et al. Everolimus with Reduced Calcineurin Inhibitor Exposure in Renal Transplantation. J Am Soc Nephrol (2018) 29:1979–91. doi:10.1681/asn.2018010009
82. Aubert, O, Divard, G, Pascual, J, Oppenheimer, F, Sommerer, C, Citterio, F, et al. Application of the iBox Prognostication System as a Surrogate Endpoint in the TRANSFORM Randomised Controlled Trial: Proof-Of-Concept Study. BMJ Open (2021) 11:e052138. doi:10.1136/bmjopen-2021-052138
83.Critical Path Institute Transplant Therapeutics Consortium. The Integrative Box (iBox) Scoring System as a Reasonably Likely Surrogate Endpoint for Five-Year Risk of Allograft Loss in Kidney Transplant Recipients for Use in Clinical Trials Evaluating the Safety and Efficacy of Novel Immunosuppressive Drug Therapies (2020). Available at https://www.fda.gov/media/139301/download (Accessed December 20, 2021).
Keywords: rejection, outcome, graft function, conditional marketing authorization, iBox
Citation: Naesens M, Budde K, Hilbrands L, Oberbauer R, Bellini MI, Glotz D, Grinyó J, Heemann U, Jochmans I, Pengel L, Reinders M, Schneeberger S and Loupy A (2022) Surrogate Endpoints for Late Kidney Transplantation Failure. Transpl Int 35:10136. doi: 10.3389/ti.2022.10136
Received: 21 October 2021; Accepted: 18 January 2022;
Published: 20 May 2022.
Copyright © 2022 Naesens, Budde, Hilbrands, Oberbauer, Bellini, Glotz, Grinyó, Heemann, Jochmans, Pengel, Reinders, Schneeberger and Loupy. This is an open-access article distributed under the terms of the Creative Commons Attribution License (CC BY). The use, distribution or reproduction in other forums is permitted, provided the original author(s) and the copyright owner(s) are credited and that the original publication in this journal is cited, in accordance with accepted academic practice. No use, distribution or reproduction is permitted which does not comply with these terms.
*Correspondence: Maarten Naesens, bWFhcnRlbi5uYWVzZW5zQGt1bGV1dmVuLmJl