- 1Unit of Infectious Diseases, Hospital Universitario “12 de Octubre”, Instituto de Investigación Sanitaria Hospital “12 de Octubre” (imas12), Madrid, Spain
- 2Centro de Investigación Biomédica en Red de Enfermedades Infecciosas (CIBERINFEC), Instituto de Salud Carlos III, Madrid, Spain
- 3Department of Medicine, School of Medicine, Universidad Complutense, Madrid, Spain
- 4Department of Nephrology, Hospital Universitario “12 de Octubre”, Instituto de Investigación Sanitaria Hospital “12 de Octubre” (imas12), Madrid, Spain
The QuantiFERON-Monitor assay (QTF-Monitor) is intended to assess innate and adaptive immune responses by quantifying interferon (IFN)-γ release upon whole blood stimulation with a TLR7/8 agonist and an anti-CD3 antibody. We performed the QTF-Monitor in 126 kidney transplant recipients (KTRs) at different points during the first 6 post-transplant months. The primary outcome was overall infection, whereas secondary outcomes included bacterial infection, opportunistic infection and de novo cancer. The association between IFN-γ production and outcomes was analyzed as “low” immune responses (<15 IU/mL) and as a continuous variable to explore alternative thresholds. There were no significant differences in the occurrence of overall infection according to the QTF-Monitor at any monitoring point. Regarding secondary outcomes, KTRs with a low response at week 2 experienced a higher incidence of bacterial infection (50.8% versus 24.4%; P-value = 0.006). Low response at month 1 was also associated with opportunistic infection (31.6% versus 14.3%; P-value = 0.033). The discriminative capacity of IFN-γ levels was poor (areas under the ROC curve: 0.677 and 0.659, respectively). No differences were observed for the remaining points or post-transplant cancer. In conclusion, the QTF-Monitor may have a role to predict bacterial and opportunistic infection in KTRs when performed early after transplantation.
Introduction
The excellent results achieved with current immunosuppressive regimens in terms of graft function and patient survival after solid organ transplantation (SOT) are threatened by the development of complications such as infections or cancer [1, 2]. Therefore, the discovery and validation of biomarkers capable of informing on the net state of immunosuppression constitutes a research priority [3]. Many of these assays are designed to quantify the adaptive response against specific pathogens, typically cytomegalovirus (CMV) [4]. In addition, some non-pathogen-specific parameters have been proven to predict the occurrence of post-transplant infection [5] or cancer [6]. None of these approaches, however, provides a comprehensive assessment of the functionality of the innate and adaptive components of the immune system.
The innate immunity is triggered through various families of pattern recognition receptors (PRRs) that detect distinct evolutionarily conserved structural motifs present in microorganisms. Toll-like receptors (TLRs) are central actors in the orchestration of the innate immunity and its interplay with the adaptive arm [7]. The activation of TLR signaling pathways leads to the transcriptional upregulation of genes involved in inflammatory responses, such as proinflammatory cytokines or type I interferons (IFNs) [8]. Research efforts have been focused on the effect exerted by immunosuppressive agents on T-cell and B-cell responses, given their role in allorecognition and graft rejection. In addition, a renewed interest has emerged on the contribution of innate responses to post-transplant events [9]. Multiple studies have shown that polymorphisms in genes encoding for PRRs modulate individual susceptibility to bacterial, viral and fungal pathogens [10–12]. It may be hypothesized that the relative contribution of innate immunity to the host defense becomes more evident upon abrogation of adaptive responses by long-term immunosuppression.
The QuantiFERON-Monitor (QTF-Monitor) is a commercial enzyme-linked immunosorbent assay (ELISA)-based IFN-γ release assay (IGRA) intended to quantify innate and adaptive immune responses following incubation of heparinized whole blood with an agonist of TLR7/8 (R848 or resiquimod) and an anti-CD3 monoclonal antibody [13]. Despite the advantages of this comprehensive approach, only a few studies have investigated the usefulness of QTF-Monitor to predict infectious complications after KT [14, 15], liver transplantation (LT) [16] or lung transplantation (LuT) [17]. In addition, no previous studies have evaluated the potential application of this assay to evaluate the risk of de novo malignancies after transplantation. The pathogenesis of this complication is multifactorial, with the participation of host (older age, sun exposure, pre-transplant history of cancer, smoking and alcohol consumption, latent infection by oncogenic viruses) and transplant-related factors (such as donor-transmitted cancer) [18]. Nevertheless, the deleterious effect of immunosuppressive therapy on cancer immune surveillance and the assumed concept that post-transplant cancer acts as a marker of over-immunosuppression provide the rationale to investigate whether an assay able to interrogate innate and adaptive responses may be also useful to predict the occurrence of malignancy.
With these research gaps in mind, we have assessed the functional immune status of a single-center cohort of KT recipients by means of the QTF-Monitor assay performed at multiple points throughout the first 6 months in order to characterize the dynamics of IFN-γ levels and their correlation with the development of infection and cancer.
Patients and Methods
Study Design and Setting
We included consecutive adult patients that underwent KT at our institution between February 2018 and July 2019. Patients experiencing primary graft non-function or early (first week) graft loss were excluded. All participants provided written informed consent at study entry, which was carried out in accordance with the ethical standards laid down in the Declarations of Helsinki and Istanbul. The study protocol was approved by the local Clinical Research Ethics Committee (reference 14/030).
All the participants were prospectively followed-up for at least 12 months, unless graft loss or death occurred earlier. Immunosuppression and prophylaxis regimens are described in Supplementary Methods. A number of pre-transplant, transplant-related and post-transplant variables were collected by means of a standardized case report form.
The QTF-Monitor assay was performed at week 2 (±4 days) and months 1, 3, and 4 (± 1 week) and 6 (±3 weeks). Peripheral blood lymphocyte subpopulations (CD3+, CD4+, and CD8+ T-cell counts) were assessed at months 1, 3, and 6 with an automated multicolor flow cytometry system (BD Multitest™ six-color TBNK reagent with acquisition on the BD FACSCanto II instrument using BD FACSCanto clinical software, all from BD Biosciences, San Jose, CA).
Study Outcomes
The primary study outcome was the incidence of post-transplant infection during the follow-up according to the functional immune competence (low versus moderate or high responses) as assessed by the QTF-Monitor assay. As secondary outcomes we separately analyzed the incidence of bacterial and opportunistic infection, as well as post-transplant de novo malignancy. For those outcomes for which a significant association with the presence of a low response (as defined by the manufacturer) was observed, alternative cut-off values for IFN-γ levels were explored on the basis of the best combination of sensitivity and specificity, as detailed below. Finally, as an additional secondary outcome we investigated the clinical variables that were associated with a low immune response at the different times after transplantation.
Procedure for the QTF-Monitor Assay
The QTF-Monitor assay (Qiagen GmbH, Hilden, Germany) was performed according to manufacturer’s recommendations. Whole blood samples were obtained by venipuncture in lithium heparin vacuum blood collection tubes, stored at room temperature and processed within less than 6 h. 1-mL aliquots were transferred to the QTF-Monitor blood collection tubes for stimulation and incubation. The QTF-Monitor lyophilized stimulants (LyoSpheres) containing the immune ligands anti-CD3 and R848 were equilibrated to room temperature, and one LyoSphere was transferred to the blood collection tube, which was gently shaken 5–10 times to ensure complete dissolution. The QTF-Monitor tubes were immediately placed into a 37°C incubator for 16–24 h. After incubation, plasma was harvested by centrifugation at 2,000 to 3,000 × g for 15 min, and stored at −80°C until analysis. The amount of IFN-γ produced was quantified in undiluted and diluted (1:10 and 1:100) plasma samples by means of the QTF-Monitor ELISA kit and given as international units (IU)/mL by means of the QTF-Monitor Analysis Software (all from Qiagen). The lyophilized IFN-γ standard was reconstituted with distilled water to prepare the standard curve. All these procedures were performed by a single technician that was blind to patient characteristics. Results were interpreted according to the cut-off values for IFN-γ proposed in the package insert: low (<15 IU/mL), moderate (15–1,000 IU/mL) and high (>1,000 IU/mL) immune responses.
Study Definitions
The diagnosis of post-transplant infection was based on microbiological findings in association with a compatible clinical syndrome according to the definitions proposed by the Centers for Disease Control and Prevention’s National Healthcare Safety Network (CDC/NHSN) [19]. Febrile episodes with no microbiological documentation that resolved spontaneously without antimicrobial treatment were excluded, as were asymptomatic bacteriuria and lower urinary tract infection. The diagnosis of CMV disease (viral syndrome or end-organ disease) required the demonstration of CMV replication by real-time PCR in the presence of attributable symptoms [20]. Opportunistic infection was operationally defined according to previous studies [21, 22] and included tuberculosis, listeriosis, infections due to facultatively intracellular bacteria (e.g., Rhodococcus), herpes simplex virus and varicella-zoster virus (shingles), proven/presumptive BK polyomavirus-associated nephropathy (BKPyVAN) [23], proven/probable invasive fungal disease (IFD) [24], Pneumocystis jirovecii pneumonia, toxoplasmosis and visceral leishmaniasis. The diagnosis of de novo cancer required histological confirmation and the absence of a pre-transplant history of such malignancy (i.e., type and site). Additional definitions are provided in Supplementary Methods.
Statistical Analysis
Quantitative data were expressed with the mean ± standard deviation (SD) or the median with interquartile range (IQR). Categorical variables were compared with the χ2 test. Student’s t-test or U Mann-Whitney test were applied for continuous variables. Repeated QTF-Monitor results within the same patient were compared with the Wilcoxon test, whereas paired proportions were compared with the McNemar test. Correlations were assessed using either Pearson’s r or Spearman’s rho. The association between the QTF-Monitor assay at each point and subsequent outcomes was explored by stratifying IFN-γ levels as per the interpretative cut-off values offered in the assay package insert (low versus moderate-high responses). Alternative cut-off values were subsequently evaluated for those primary or secondary outcomes depicting significant associations in the previous approach by means of the Youden’s J statistic, which combines sensitivity and specificity into a single measure (J = sensitivity + specificity − 1). The discriminative capacity of IFN-γ levels analyzed as a continuous variable was explored with the area under the receiving operating characteristic (auROC) curve. We estimated the diagnostic accuracy (sensitivity, specificity, positive [PPV] and negative predictive values [NPV] with the corresponding 95% confidence intervals [CIs]). Time-to-event curves were plotted by the Kaplan-Meier method and inter-group differences were compared with the log-rank test. IFN-γ levels were log10-transformed for statistical analyses. Statistical analysis was performed with SPSS version 29.0.1.0 (IBM Corp., Armonk, NY).
Results
Clinical Characteristics
The study cohort comprised 126 KT recipients (Table 1). The QTF-Monitor assay was performed at 439 different instances, with a median of 4 (IQR: 3–4) measurements per patient. In detail, the assay was available for 112 patients at week 2 (91.1% of those that survived with a functioning graft at that point), 108 patients at month 1 (87.8%), 67 patients at month 3 (54.9%), 52 patients at month 4 (42.9%), and 100 patients at month 6 (82.6%).
Post-Transplant Kinetics and Clinical Determinants of IFN-γ Production
Overall, median IFN-γ levels showed a significant increase from week 2 [0.9 (IQR: 0.1–1.8) log10 IU/mL] to month 1 [1.5 (IQR: 0.9–2.2) log10 IU/mL; P-value < 0.0001] and month 3 [1.9 (IQR: 1.4–2.7) log10 IU/mL; P-value < 0.0001], to reach a plateau beyond that point. In accordance, the proportion of patients with a low immune response (<15 IU/mL) decreased from week 2 [58.9% (66/112)] to month 6 [24.0% (24/100); P-value < 0.0001] (Figure 1).
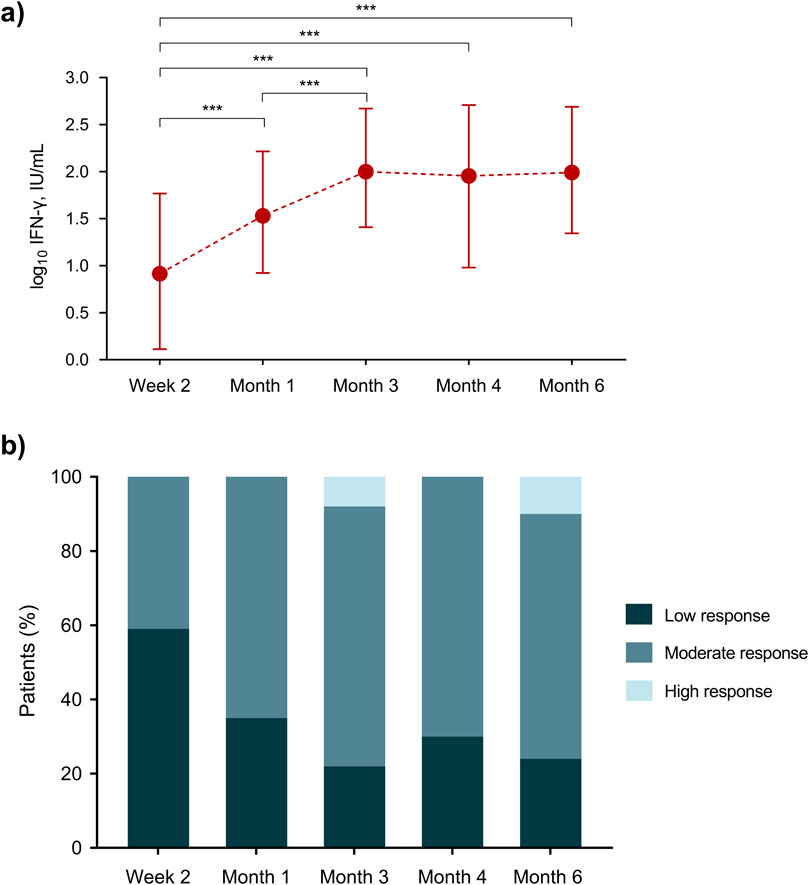
Figure 1. (A) Kinetics of IFN-γ levels measured by the QTF-Monitor assay; points and bars show the median and interquartile range, respectively. (B) Proportion of patients with different immune responses according to the cut-off values for IFN-γ production proposed by the manufacturer [low (<15 IU/mL), moderate (15–1,000 IU/mL) and high (>1,000 IU/mL)]. *** P-value for repeated measures <0.0001. IFN-γ: interferon-γ; IU: international unit.
We explored the clinical variables predictive of a low immune response. Pre-transplant dialysis [93.9% (62/66) versus 78.3% (36/46); P-value = 0.014], induction therapy with antithymocyte globulin (ATG) [59.1% (39/66) versus 28.3% (13/46); P-value = 0.001] and delayed graft function [45.5% (30/66) versus 21.7% (10/46); P-value = 0.016] were more common in KT recipients exhibiting a low response at week 2 (Supplementary Table S1). The associations with pre-transplant dialysis and ATG induction were also observed for the results of the assay at month 1. Living donation was less likely in recipients with low responses at that point [5.3% (2/38) versus 27.1% (19/70); P-value = 0.006]. In addition, absolute lymphocyte and CD3+ and CD4+ T-cell counts were lower in this group (Supplementary Table S2). No significant associations were found between clinical features or laboratory values and the assay results at month 6 (Supplementary Table S3).
To further investigate the effect of induction therapy, we analyzed IFN-γ levels as a continuous variable. Patients treated with ATG showed a significantly lower production of IFN-γ at week 2 and month 1 as compared to those that received basiliximab or no induction (Figure 2). In accordance with the lymphocyte-depleting effect of ATG, a significant correlation was observed between IFN-γ levels and CD3+, CD4+ and CD8+ T-cell counts at month 1 (but not at months 3 or 6), with Spearman’s Rho coefficients ranging from 0.346 to 0.378 (Supplementary Figure S1).
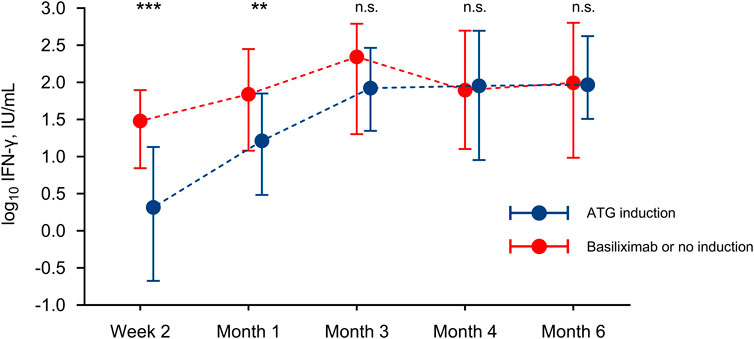
Figure 2. Kinetics of IFN-γ levels according to the administration of induction therapy with ATG; points and bars show the median and interquartile range, respectively. ** P-value < 0.001, *** P-value < 0.0001. ATG, antithymocyte globulin; IFN-γ, interferon-γ; IU, international unit; ns, not significant.
We also investigated whether IFN-γ production was correlated with concurrent measurements of tacrolimus trough levels. We only found a weak inverse correlation at month 6 after transplantation (Pearson’s r: −0.338; P-value = 0.010), whereas no correlations were observed for week 2 (r: −0.181; P-value = 0.152), month 1 (r: −0.001; P-value = 0.993), month 3 (r: −0.049; P-value = 0.771) or month 4 (r: 0.033; P-value = 0.876).
Post-Transplant Infection and Cancer
Overall, 72 patients (57.1%) experienced 145 episodes of post-transplant infection (primary outcome). The median interval to the first episode was 83.5 days (IQR: 26.5–227.8). Acute graft pyelonephritis [51 episodes (35.2%)] and pneumonia [17 (11.7%)] were the most common types. Enterobacterales accounted for most of the microbiologically documented cases, with predominance of Escherichia coli [33 episodes (22.7%)] and Klebsiella pneumoniae [24 (16.5%)] (Supplementary Table S4).
Regarding secondary outcomes, 50 patients (39.7%) were diagnosed with 105 episodes of bacterial infection [median interval to the first episode of 64.5 days (IQR: 17.8–196)]. On the other hand, 28 episodes in 26 patients (20.6%) met the definition of opportunistic infection [median interval of 167.5 days (IQR: 82.8–295.8)], with CMV disease [12 episodes (42.9%)] and herpes zoster [6 (21.4%)] as the most common forms (Supplementary Table S5). Eleven patients (8.7%) developed de novo cancer at a median of 364 days (IQR: 169.5–594). In detail, there were six cases of non-melanoma skin cancer and six cases of solid cancer (one patient had both) (Supplementary Table S6).
Association Between the Functionality of Immune Response and Overall Post-transplant Infection
There were no significant differences in the cumulative incidence of overall infection between KT recipients exhibiting a low immune response (IFN-γ <15 IU/mL) and those with a moderate or high response at each monitoring point. We only found a non-significant trend towards a higher risk among patients with low responses at month 1 [65.8% (25/38) versus 47.1% (33/70); P-value = 0.063] (Figure 3A). There were no significant differences in IFN-γ levels (taken as a continuous variable) between patients with or without infection (Figure 3B).
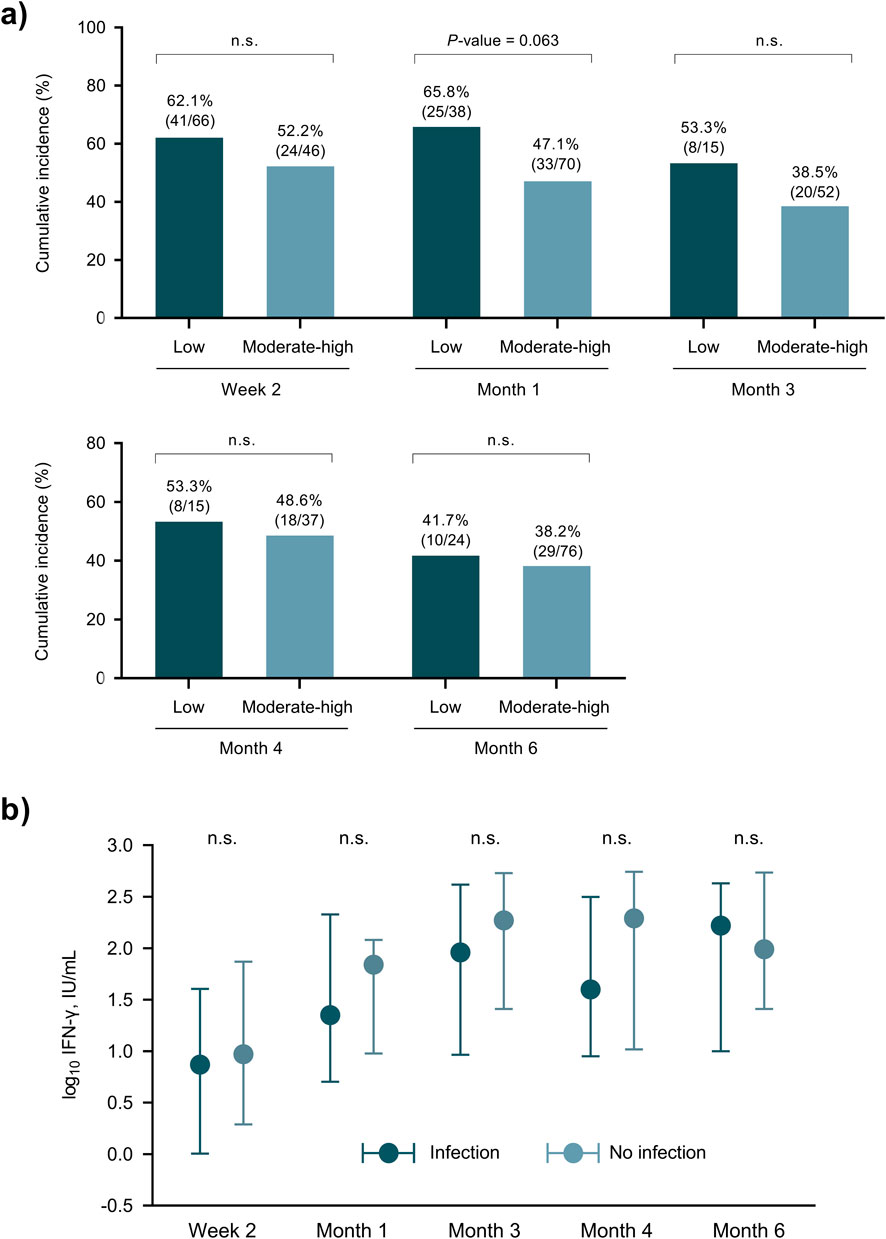
Figure 3. (A) Cumulative incidence of post-transplant infection (primary outcome) according to the immune response in the QTF-Monitor assay at different time points after transplantation. (B) IFN-γ levels according to the subsequent occurrence of infection; points and bars show the median and interquartile range, respectively. ns, not significant; IU, international unit.
As a measure of sustained over-immunosuppression, we compared the incidence of infection between KT recipients with responses categorized as low in all the assays performed throughout the first post-transplant months and the rest of the cohort. There were no significant differences for persistent low responses either during the first 3 [45.5% (10/22) versus 43.0% (43/100); P-value = 1.000] or 6 months [33.3% (6/18) versus 35.9% (37/103), respectively; P-value = 1.000].
Association Between the Functionality of Immune Response and Secondary Outcomes
Patients with a low response at the early (2-week) assessment had a higher cumulative incidence of bacterial infection than those with an intermediate response [50.8% (33/65) versus 24.4% (11/45), respectively; P-value = 0.006] (Figure 4A). IFN-γ production at week 2 was accordingly lower among patients developing bacterial infection (Figure 4B). One-year bacterial infection-free survival was significantly lower in the presence of a low response (Figure 4C). On the contrary, there were no differences for the remaining points in terms of the magnitude of response (low versus intermediate-high) (Figure 4A; Supplementary Table S7) or the absolute IFN-γ level (Supplementary Table S8).
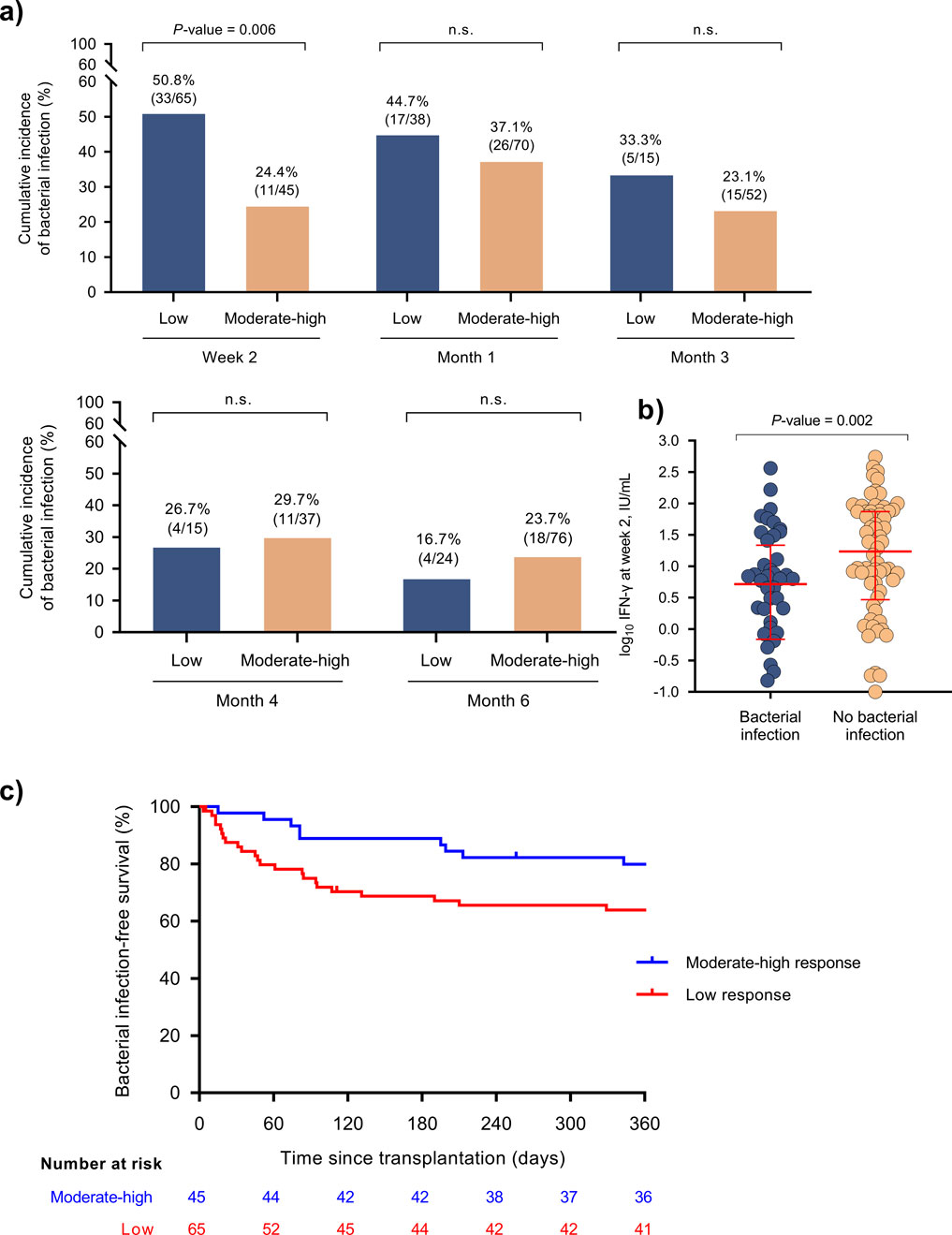
Figure 4. (A) Cumulative incidence of post-transplant bacterial infection (secondary outcome) according to the immune response in the QTF-Monitor assay at different time points after transplantation. (B) IFN-γ levels at week 2 according to the subsequent occurrence of bacterial infection; points and bars show the median and interquartile range, respectively. (C) Bacterial infection-free survival according to the immune response at week 2 (log-rank test P-value = 0.009). IFN-γ, interferon-γ; IU, international unit.
Regarding opportunistic infection, the presence of a low response at month 1 was associated with the subsequent development of this secondary outcome [31.6% (12/38) versus 14.3% (10/70); P-value = 0.033] (Figure 5A). The IFN-γ level at this point was also lower in patients developing opportunistic infection (Figure 5B), as was the 1-year event-free survival in patients with a low response (Figure 5C). No differences were observed for the remaining time points (Supplementary Tables S7 and S8).
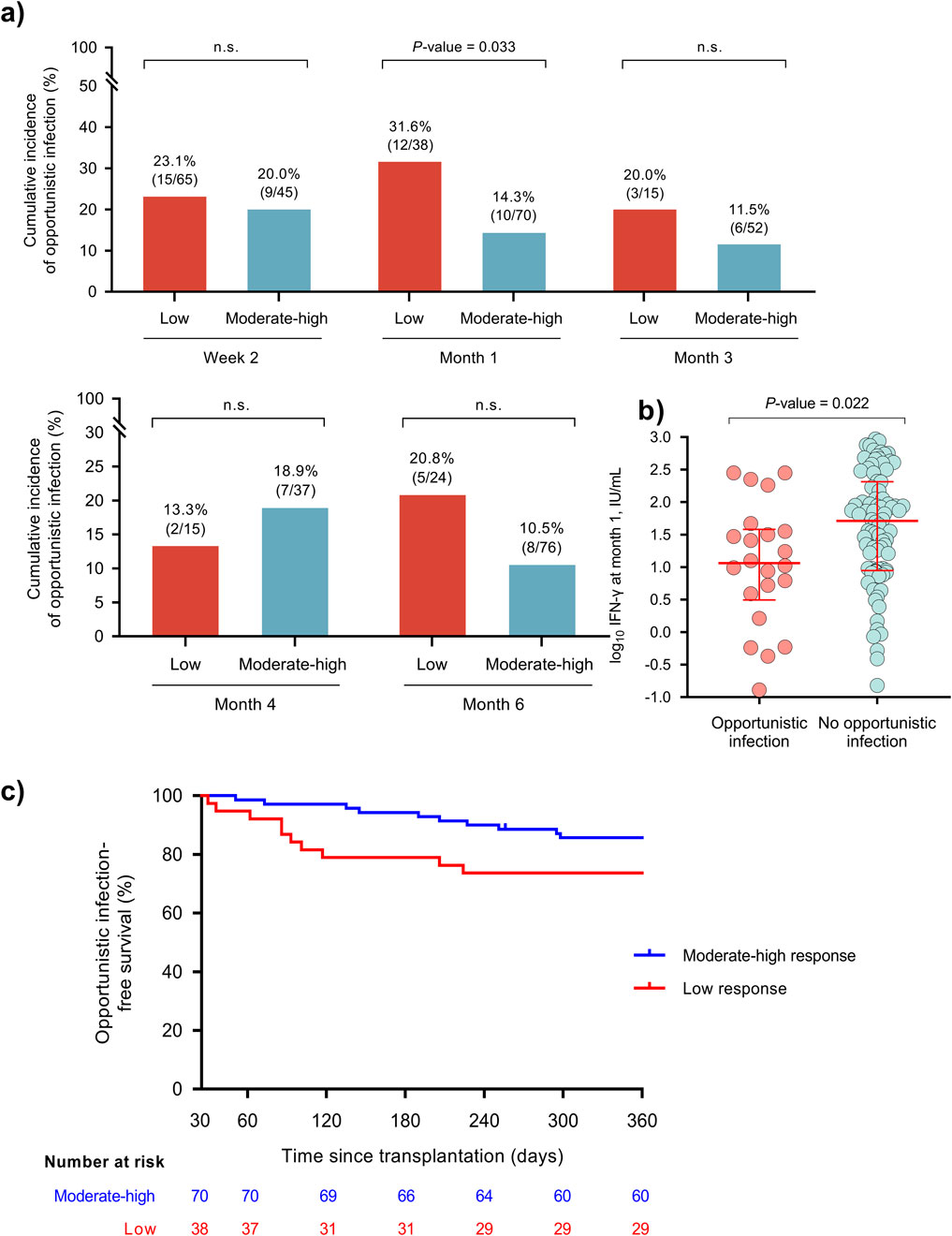
Figure 5. (A) Cumulative incidence of post-transplant opportunistic infection (secondary outcome) according to the immune response in the QTF-Monitor assay at month 1. (B) IFN-γ levels at month 1 according to the subsequent occurrence of bacterial infection; points and bars show the median and interquartile range, respectively. (C) Opportunistic infection-free survival according to the immune response at week 2 (log-rank test P-value = 0.026). IFN-γ, interferon-γ; IU, international unit.
Finally, there were no differences in the incidence of de novo malignancy according to the functionality of immune responses (Figure 5A; Supplementary Table S7) or IFN-γ levels (Supplementary Table S8).
Diagnostic Accuracy of the QTF-Monitor Assay to Predict Bacterial and Opportunistic Infection
In view of the associations found at the early assessment, we further explored the diagnostic accuracy for the secondary outcomes of bacterial and opportunistic infection. By applying the cut-off value proposed by the manufacturer (IFN-γ <15 IU/mL), we obtained a sensitivity of 75.0% (95% CI: 59.7–86.8) and specificity of 51.5% (95% CI: 38.9–64.0) to predict bacterial infection beyond week 2. The corresponding values for the development of opportunistic infection beyond month 1 were 54.6% (95% CI: 32.2–75.6) and 69.8% (95% CI: 58.9–79.2), respectively (Supplementary Table S9). The discriminative capacity of IFN-γ levels was overall low, with auROCs for predicting bacterial and opportunistic infection of 0.677 (95% CI: 0.576–0.778) and 0.659 (95% CI: 0.539–0.779), respectively.
We also explored alternative cut-off values according to the optimal balance between sensitivity and specificity. By using a threshold at week 2 of 7.9 IFN-γ IU/mL, the 1-year bacterial infection-free survival curve of patients with low response was more clearly separated from those above the cut-off (Supplementary Figure S2), yielding improved specificity [66.7% (95% CI: 53.9–77.8)] and PPV [57.7% (95% CI: 47.8–66.9)] at the expense of a loss of sensitivity [68.2% (95% CI: 52.4–81.4)]. On the other hand, the optimal cut-off value to predict opportunistic infection beyond month 1 was set at 47.3 IU/mL, which also resulted in more clearly separated even-free survival curves (Supplementary Figure S3). As compared to the manufacturer’s criterion, this alternative cut-off resulted in improved sensitivity [81.8% (95% CI: 59.7–94.8)] and NPV [91.7% (95% CI: 81.6–96.5)], but poorer specificity (51.2% 95% CI: 40.1–62.1) (Supplementary Table S9).
Discussion
Most of the biomarkers proposed to determine the net state of immunosuppression after SOT share two limitations: the lack of functional measurements ―as is the case with immunoglobulin levels or lymphocyte counts [3]― and the sole interrogation of virus-specific adaptive responses [4]. The QTF-Monitor assay offers the possibility of a broader functional assessment by measuring IFN-γ release upon in vitro stimulation of the innate and adaptive arms [13]. In the present experience the assay’s performance was moderate at best, since no association could be demonstrated between IFN-γ production (either categorized as “low” immune responses or as a continuous variable) at different points during the first 6 months and the primary outcome of overall infection. Nevertheless, we found that the QTF-Monitor results obtained during the first weeks may still be valuable to specifically predict the occurrence of bacterial or opportunistic infection, although this finding should be taken with caution due to the non-negligible false positive risk in the assessment of secondary outcomes. On the other hand, no apparent associations were found for de novo cancer.
The performance of the QTF-Monitor assay to predict post-transplant infection has been investigated by a few groups, with variable reported accuracy [14–17]. In a mixed cohort of 137 SOT recipients, Mian et al. observed that IFN-γ levels measured between months 1 and 6 were significantly lower in patients that developed subsequent infection and proposed an optimal threshold of ≤10 IU/mL. Urinary tract infection and pneumonia were the most common syndromes during the early post-transplant period, with a shift to predominance of viral pathogens beyond month 3. No multivariate analysis was performed to confirm the predictive value of IFN-γ production [14]. In contrast, a cross-sectional study at a mean of 2.6 post-transplant years failed to show differences in IFN-γ levels between stable KT recipients and those with infection. A subgroup analysis revealed that patients with bacterial infection had a significantly decreased IFN-γ release. Such an association, however, was not confirmed after adjustment for steroid dose and tacrolimus levels [15]. In a single-center cohort of LT recipients, IFN-γ levels at week 1 exhibited a fairly good capacity to predict infection through the first month, with the majority of the events being classified as opportunistic [16]. Finally, a recent study recruited 80 LuT recipients in which the QTF-Monitor was performed at 2, 6, 12, 24, and 52 weeks. The presence of IFN-γ levels <10 and <60 IU/mL at weeks 12 and 24, respectively, was associated with the diagnosis of opportunistic infection (mainly CMV viremia and IFD). Similar results were not observed for earlier monitoring points [17].
The discordant results from the existing literature, including those reported herein, may be partially attributable to differences across studies in outcomes and definitions, as well as in the timing and frequency of monitoring. Taken together, they would suggest that the QTF-Monitor assay may perform better for predicting some specific types of infection ―particularly of bacterial origin [15]― and when performed early after transplantation. Indeed, we have only identified differences in the assay results obtained at week 2 and month 1 according to the subsequent diagnosis of bacterial and opportunistic infection, respectively (with the latter mostly represented by CMV disease and herpes zoster). These results are in line with those previously observed among LT recipients [16]. Of note, the discriminative capacity for both outcomes was low, as indicated by auROC values below 0.700. Sood et al. reported a slightly better accuracy for the results obtained at week 1 after LT (auROC of 0.740) [16]. To put these findings into context, our group has reported higher discriminative capacities for other non-pathogen-specific biomarkers, such as the CD8+ T-cell count at month 1 (auROC of 0.739) or the total lymphocyte count at month 6 (auROC of 0.820) to predict opportunistic infection [25], Torque Teno virus (TTV DNAemia) at month 1 for predicting opportunistic infection and/or cancer (auROC of 0.704) [22], or serum sCD30 at month 1 for predicting bacterial infection (auROC of 0.846) [26]. Therefore, the potential contribution of the assay to the existing prediction models for post-transplant infection ―such as the externally validated SIMPLICITY Score [5]― should be explored in future studies.
Our results align with the cross-sectional study by Margeta et al [15] in that the performance of QTF-Monitor assay decreases at late periods after transplantation, once the amount of immunosuppression has been stabilized in most recipients. No differences in IFN-γ levels beyond month 1 were observed for any of the outcomes analyzed. Interestingly, we found no association between the QTF-Monitor results and the development of post-transplant cancer, a complication that usually results from the long-term effect of sustained over-immunosuppression [27]. No previous studies have investigated the role of QTF-Monitor assay to predict de novo malignancy. Although the number of events was low (n = 14), this negative finding would point to a lower relative contribution to the assay results of T-cell responsiveness (as compared to TLR-mediated innate responses), taken into account the pivotal role of cellular immunity in cancer immune surveillance. In contrast, we and others have shown that certain immune biomarkers assessed within the first months are useful to identify SOT recipients at increased risk of developing cancer in the mid- and long-term follow-up, such as CD4+ and CD8+ T-cell counts [6, 28], monocytic myeloid-derived suppressor cells [29] or TTV DNAemia [22].
The kinetics of IFN-γ levels measured by the QTF-Monitor assay was comparable to previous studies, which typically describe a sharp decline from the pre-transplant assessment followed by a progressive recovery through months 3–6 and a plateau thereafter [13, 14, 16, 17]. This pattern is in line with the accepted timing for immune reconstitution after SOT, as validated with other biomarkers such as TTV DNA load [30, 31]. The clinical factors influencing assay results have been only partially investigated. The association between the use of ATG as induction therapy and a lower IFN-γ production has been reported by other authors [14]. In our experience this effect persisted until month 1 and was supported by the inverse correlation observed between IFN-γ levels and T-cell counts. The impact of tacrolimus levels is less consistent, with studies reporting either strong [17] or borderline correlations [15], or even no apparent association [14]. We only found a weak inverse correlation with tacrolimus levels at month 6. Mian et al. also reported an association with daily doses of prednisone and mycophenolate [14], which were not recorded in our database. Although beyond the scope of our research, we found no significant association between the immune status measured by the QTF-Monitor assay at the different monitoring points and the subsequent occurrence of biopsy-proven acute rejection (data not shown). Patients with a low response at week 2 were more likely to have received pre-transplant dialysis and to have experienced delayed graft function (defined by the early requirement of renal replacement therapy). The deleterious effect of dialysis on the T-cell ability to produce IFN-γ after specific stimulation is well established for IGRAs used to detect Mycobacterium tuberculosis infection due to insufficient mitogen response and premature immune aging [32, 33]. Inversely, living donation was associated with a more robust immune response, which may be explained by the lower recipient age and the immediate graft function in this subgroup.
What may be the position of the QTF-Monitor assay for immune monitoring in the clinical arena? With the limitations inherent to multiple secondary outcome analyses and the lack of consistent associations at later points, our results would point out to the potential usefulness of the early assessment within the first weeks with the specific aim of predicting bacterial infection. By decreasing the IFN-γ threshold to <7.9 IU/mL we obtained a sensible improvement in specificity without a major impact in sensitivity, although the resulting estimates (66.7% and 68.2%, respectively) were far from excellent. Sood et al. proposed a clinical threshold of <1.30 IU/mL as the most discriminative to predict infection beyond the first week after LT, with a diagnostic accuracy in the line of our results (sensitivity of 71.4% and specificity of 63.0%) [16]. On the other hand, an alternative threshold (<47.3 IU/mL) at month 1 yielded a reasonable sensitivity (81.8%) to predict opportunistic infection, at the expense of a poor specificity (51.2%). Gardiner et al. also found a relatively low discriminative ability for different outcomes (overall infection, severe infection or opportunistic infection) and monitoring points after LuT [17]. In our opinion, any decision regarding the implementation of the QTF-Monitor assay in daily practice must balance diagnostic accuracy (which was found to be suboptimal in our experience), requirement of laboratory resources and economic costs with those of alternative biomarkers [3]. For instance, the observed impact on IFN-γ production of ATG induction and CD4+ and CD8+ T-cell counts would suggest that low responses may ultimately act as a surrogate for the presence of lymphocytopenia, which constitutes a well-established biomarker for opportunistic infection [25, 34–38].
Our study is based on a large cohort of KT recipients with regular monitoring, and it is strengthened by the assessment of immunosuppression-related complications which comprised infections and malignancies. We also provided an insight into the clinical determinants of the IFN-γ kinetics, including peripheral blood lymphocyte subpopulations. Nevertheless, a number of limitations must be noted, such as the relatively low number of some events, which may have limited statistical power. Due to logistical reasons, the assay could not be tested in certain patients at all the scheduled points. Although the minimum follow-up was set at post-transplant month 12, the last monitoring point was performed at month 6. In addition to budgetary considerations, the rationale for such decision was that most events would have occurred within the first 6 months, according to the classical timeline for post-transplant infection [39]. In addition, the overall amount of immunosuppression (i.e., prednisone dose and targeted trough tacrolimus levels) is usually stabilized beyond that point in most KT recipients. Therefore, it is not to be expected major changes in the results of the QTF-Monitor assay beyond month 6, as supported by the plateau between months 3 and 6 observed for IFN-γ levels (Figure 1). In addition, any conclusion on the potential usefulness of the QTF-Monitor assay for predicting bacterial or opportunistic infection should take into account that both events were considered as secondary outcomes.
In this cohort of KT recipients we found no significant association between IFN-γ production measured with the QTF-Monitor assay and the primary outcome of overall post-transplant infection. Secondary outcome analysis would suggest that the usefulness of this assay is presumably limited to the prediction of bacterial and opportunistic infection when performed within the first weeks after transplantation. Further studies are needed to establish the role of this promising method in the available repertoire of non-pathogen-specific immune monitoring biomarkers.
Data Availability Statement
The raw data supporting the conclusions of this article will be made available by the authors, without undue reservation.
Ethics Statement
The studies involving humans were approved by the Clinical Research Ethics Committee of the University Hospital “12 de Octubre” (reference 14/030). The studies were conducted in accordance with the local legislation and institutional requirements. The participants provided their written informed consent to participate in this study.
Author Contributions
MF-R, JMA, and NR designed research; TR-M, PP, and NR performed laboratory experiments; MF-R, IR-G, JMC, FL-M, RSJ, NP, EG, and AA performed patient selection and management; MF-R, TR-M, IR-G, JMC, FL-M, RSJ, NP, and EG performed data collection; MF-R and NR performed data analysis; MF-R wrote the paper; and AA, JMA, and NR critically reviewed and completed the final draft of the manuscript. All authors contributed to the article and approved the submitted version.
Funding
The author(s) declare that financial support was received for the research, authorship, and/or publication of this article. This work was supported by the Instituto de Salud Carlos III (ISCIII) through the projects PIE13/00045, PI19/01300, and PI22/01062, and co-funded by the European Union. MF-R has hold a contract “Miguel Servet” (CP18/00073) from the ISCIII, Spanish Ministry of Science and Innovation. This study was partially presented at the 32nd European Congress of Clinical Microbiology and Infectious Diseases (ECCMID), which was held in Lisbon, Portugal, from 23 to 26 April 2022 (oral communication O0842).
Conflict of Interest
The authors declare that the research was conducted in the absence of any commercial or financial relationships that could be construed as a potential conflict of interest.
Supplementary Material
The Supplementary Material for this article can be found online at: https://www.frontierspartnerships.org/articles/10.3389/ti.2024.13551/full#supplementary-material
Abbreviations
ATG, antithymocyte globulin; auROC, area under the receiving operating characteristic curve; BKPyVAN, BK polyomavirus-associated nephropathy; CI, confidence interval; CMV, cytomegalovirus; ELISA, enzyme-linked immunosorbent assay; IFD, invasive fungal disease; IFN, interferon; IGRA, interferon-γ release assay; IQR, interquartile range; IU, international unit; KT, kidney transplantation; LT, liver transplantation; LuT, lung transplantation; NPV, negative predictive value; PRR, pattern recognition receptor; PPV, positive predictive value; QTF-Monitor, QuantiFERON Monitor; SD, standard deviation; SOT, solid organ transplantation; TLR, toll-like receptor; TTV, Torque Teno virus.
References
1. Ying, T, Shi, B, Kelly, PJ, Pilmore, H, Clayton, PA, and Chadban, SJ. Death After Kidney Transplantation: An Analysis by Era and Time Post-Transplant. J Am Soc Nephrol (2020) 31(12):2887–99. doi:10.1681/ASN.2020050566
2. Awan, AA, Niu, J, Pan, JS, Erickson, KF, Mandayam, S, Winkelmayer, WC, et al. Trends in the Causes of Death Among Kidney Transplant Recipients in the United States (1996-2014). Am J Nephrol (2018) 48(6):472–81. doi:10.1159/000495081
3. Fernandez-Ruiz, M, Lopez-Medrano, F, and Aguado, JM. Predictive Tools to Determine Risk of Infection in Kidney Transplant Recipients. Expert Rev Anti Infect Ther (2020) 18(5):423–41. doi:10.1080/14787210.2020.1733976
4. Yong, MK, Lewin, SR, and Manuel, O. Immune Monitoring for CMV in Transplantation. Curr Infect Dis Rep (2018) 20(4):4. doi:10.1007/s11908-018-0610-4
5. Fernández-Ruiz, M, Seron, D, Alonso, Á, Lora, D, Hernández, D, González, E, et al. Derivation and External Validation of the SIMPLICITY Score as a Simple Immune-Based Risk Score to Predict Infection in Kidney Transplant Recipients. Kidney Int (2020) 98(4):1031–43. doi:10.1016/j.kint.2020.04.054
6. Thibaudin, D, Alamartine, E, Mariat, C, Absi, L, and Berthoux, F. Long-Term Kinetic of T-Lymphocyte Subsets in Kidney-Transplant Recipients: Influence of Anti-T-cell Antibodies and Association With Posttransplant Malignancies. Transplantation (2005) 80(10):1514–7. doi:10.1097/01.tp.0000181193.98026.3f
7. Fitzgerald, KA, and Kagan, JC. Toll-like Receptors and the Control of Immunity. Cell (2020) 180(6):1044–66. doi:10.1016/j.cell.2020.02.041
8. Takeuchi, O, and Akira, S. Pattern Recognition Receptors and Inflammation. Cell (2010) 140(6):805–20. doi:10.1016/j.cell.2010.01.022
9. Pontrelli, P, Grandaliano, G, and Van Kooten, C. Editorial: Kidney Transplantation and Innate Immunity. Front Immunol (2020) 11:603982. doi:10.3389/fimmu.2020.603982
10. Lee, SO, Brown, RA, Kang, SH, Abdel Massih, RC, and Razonable, RR. Toll-Like Receptor 4 Polymorphisms and the Risk of Gram-Negative Bacterial Infections After Liver Transplantation. Transplantation (2011) 92(6):690–6. doi:10.1097/TP.0b013e31822b589f
11. Redondo, N, Navarro, D, Aguado, JM, and Fernández-Ruiz, M. Human Genetic Polymorphisms and Risk of Viral Infection After Solid Organ Transplantation. Transpl Rev (Orlando) (2022) 36(1):100669. doi:10.1016/j.trre.2021.100669
12. Wojtowicz, A, Gresnigt, MS, Lecompte, T, Bibert, S, Manuel, O, Joosten, LAB, et al. IL1B and DEFB1 Polymorphisms Increase Susceptibility to Invasive Mold Infection after Solid-Organ Transplantation. J Infect Dis (2015) 211(10):1646–57. doi:10.1093/infdis/jiu636
13. Sood, S, Cundall, D, Yu, L, Miyamasu, M, Boyle, JS, Ong, SY, et al. A Novel Biomarker of Immune Function and Initial Experience in a Transplant Population. Transplantation (2014) 97(8):e50–1. doi:10.1097/TP.0000000000000078
14. Mian, M, Natori, Y, Ferreira, V, Selzner, N, Husain, S, Singer, L, et al. Evaluation of a Novel Global Immunity Assay to Predict Infection in Organ Transplant Recipients. Clin Infect Dis (2018) 66(9):1392–7. doi:10.1093/cid/cix1008
15. Margeta, I, Marekovic, I, Pesut, A, Zelenika, M, Dorotić, M, Mrnjec, I, et al. Evaluation of Cell-Mediated Immune Response by QuantiFERON Monitor Assay in Kidney Transplant Recipients Presenting With Infective Complications. Medicine (Baltimore) (2020) 99(27):e21010. doi:10.1097/MD.0000000000021010
16. Sood, S, Haifer, C, Yu, L, Pavlovic, J, Churilov, L, Gow, PJ, et al. A Novel Immune Function Biomarker Identifies Patients at Risk of Clinical Events Early Following Liver Transplantation. Liver Transpl (2017) 23(4):487–97. doi:10.1002/lt.24730
17. Gardiner, BJ, Lee, SJ, Cristiano, Y, Levvey, BJ, Sullivan, LC, Snell, GI, et al. Evaluation of Quantiferon®-Monitor as a Biomarker of Immunosuppression and Predictor of Infection in Lung Transplant Recipients. Transpl Infect Dis (2021) 23(3):e13550. doi:10.1111/tid.13550
18. Turshudzhyan, A. Post-Renal Transplant Malignancies: Opportunities for Prevention and Early Screening. Cancer Treat Res Commun (2021) 26:100283. doi:10.1016/j.ctarc.2020.100283
19. Centers for Disease Control and Prevention/National Healthcare Safety Network. CDC/NHSN Surveillance Definitions for Specific Types of Infections (2024). Available from: https://www.cdc.gov/nhsn/pdfs/pscmanual/17pscnosinfdef_current.pdf (Accessed October 25, 2024).
20. Ljungman, P, Boeckh, M, Hirsch, HH, Josephson, F, Lundgren, J, Nichols, G, et al. Definitions of Cytomegalovirus Infection and Disease in Transplant Patients for Use in Clinical Trials. Clin Infect Dis : official Publ Infect Dis Soc America (2017) 64(1):87–91. doi:10.1093/cid/ciw668
21. Fernandez-Ruiz, M, Kumar, D, Husain, S, Lilly, L, Renner, E, Mazzulli, T, et al. Utility of a Monitoring Strategy for Human Herpesviruses 6 and 7 Viremia after Liver Transplantation: A Randomized Clinical Trial. Transplantation (2015) 99(1):106–13. doi:10.1097/TP.0000000000000306
22. Fernandez-Ruiz, M, Albert, E, Gimenez, E, Ruiz-Merlo, T, Parra, P, López-Medrano, F, et al. Monitoring of Alphatorquevirus DNA Levels for the Prediction of Immunosuppression-Related Complications After Kidney Transplantation. Am J Transpl (2019) 19(4):1139–49. doi:10.1111/ajt.15145
23. Hirsch, HH, Randhawa, PS, and Practice, ASTIDC. BK Polyomavirus in Solid Organ Transplantation-Guidelines from the American Society of Transplantation Infectious Diseases Community of Practice. Clin Transpl (2019) 33(9):e13528. doi:10.1111/ctr.13528
24. Donnelly, JP, Chen, SC, Kauffman, CA, Steinbach, WJ, Baddley, JW, Verweij, PE, et al. Revision and Update of the Consensus Definitions of Invasive Fungal Disease from the European Organization for Research and Treatment of Cancer and the Mycoses Study Group Education and Research Consortium. Clin Infect Dis (2020) 71(6):1367–76. doi:10.1093/cid/ciz1008
25. Fernandez-Ruiz, M, Lopez-Medrano, F, Allende, LM, Andrés, A, García-Reyne, A, Lumbreras, C, et al. Kinetics of Peripheral Blood Lymphocyte Subpopulations Predicts the Occurrence of Opportunistic Infection after Kidney Transplantation. Transpl Int (2014) 27(7):674–85. doi:10.1111/tri.12321
26. Fernandez-Ruiz, M, Parra, P, Lopez-Medrano, F, Ruiz-Merlo, T, González, E, Polanco, N, et al. Serum sCD30: A Promising Biomarker for Predicting the Risk of Bacterial Infection after Kidney Transplantation. Transpl Infect Dis (2017) 19(2). doi:10.1111/tid.12668
27. Cheung, CY, and Tang, SCW. An Update on Cancer after Kidney Transplantation. Nephrol Dial Transpl (2019) 34(6):914–20. doi:10.1093/ndt/gfy262
28. Fernandez-Ruiz, M, Lopez-Medrano, F, Allende, LM, Andrés, A, Paz-Artal, E, and Aguado, JM. Assessing the Risk of De Novo Malignancy in Kidney Transplant Recipients: Role for Monitoring of Peripheral Blood Lymphocyte Populations. Transplantation (2014) 98(5):e36–7. doi:10.1097/TP.0000000000000297
29. Utrero-Rico, A, Laguna-Goya, R, Cano-Romero, F, Chivite-Lacaba, M, Gonzalez-Cuadrado, C, Rodríguez-Sánchez, E, et al. Early Posttransplant Mobilization of Monocytic Myeloid-Derived Suppressor Cell Correlates With Increase in Soluble Immunosuppressive Factors and Predicts Cancer in Kidney Recipients. Transplantation (2020) 104(12):2599–608. doi:10.1097/TP.0000000000003179
30. Redondo, N, Navarro, D, Aguado, JM, and Fernández-Ruiz, M. Viruses, Friends, and Foes: The Case of Torque Teno Virus and the Net State of Immunosuppression. Transpl Infect Dis (2022) 24(2):e13778. doi:10.1111/tid.13778
31. Jaksch, P, Gorzer, I, Puchhammer-Stockl, E, and Bond, G. Integrated Immunologic Monitoring in Solid Organ Transplantation: The Road toward Torque Teno Virus-Guided Immunosuppression. Transplantation (2022) 106(10):1940–51. doi:10.1097/TP.0000000000004153
32. Lee, SS, Chou, KJ, Dou, HY, Huang, TS, Ni, YY, Fang, HC, et al. High Prevalence of Latent Tuberculosis Infection in Dialysis Patients Using the Interferon-Gamma Release Assay and Tuberculin Skin Test. Clin J Am Soc Nephrol (2010) 5(8):1451–7. doi:10.2215/CJN.01790210
33. Putri, DU, Chen, CL, Wang, CH, Sue, YM, Tseng, PC, Lin, CF, et al. Hemodialysis Acutely Altered Interferon-Gamma Release Assay Test Result and Immune Cell Profile. J Microbiol Immunol Infect (2022) 55(2):332–5. doi:10.1016/j.jmii.2021.03.022
34. Gardiner, BJ, Nierenberg, NE, Chow, JK, Ruthazer, R, Kent, DM, and Snydman, DR. Absolute Lymphocyte Count: A Predictor of Recurrent Cytomegalovirus Disease in Solid Organ Transplant Recipients. Clin Infect Dis (2018) 67(9):1395–402. doi:10.1093/cid/ciy295
35. Yoon, M, Oh, J, Chun, KH, Lee, CJ, and Kang, SM. Post-Transplant Absolute Lymphocyte Count Predicts Early Cytomegalovirus Infection after Heart Transplantation. Sci Rep (2021) 11(1):1426. doi:10.1038/s41598-020-80790-4
36. Perry, WA, Paulus, JK, Price, LL, Snydman, DR, and Chow, JK. Association Between Lymphopenia at 1 Month Posttransplant and Infectious Outcomes or Death in Heart Transplant Recipients. Clin Infect Dis (2021) 73(11):e3797–e3803. doi:10.1093/cid/ciaa1800
37. Schoeberl, AK, Zuckermann, A, Kaider, A, Aliabadi-Zuckermann, A, Uyanik-Uenal, K, Laufer, G, et al. Absolute Lymphocyte Count as a Marker for Cytomegalovirus Infection After Heart Transplantation. Transplantation (2023) 107(3):748–52. doi:10.1097/TP.0000000000004360
38. Calarota, SA, Chiesa, A, De Silvestri, A, Morosini, M, Oggionni, T, Marone, P, et al. T-Lymphocyte Subsets in Lung Transplant Recipients: Association between Nadir CD4 T-Cell Count and Viral Infections after Transplantation. J Clin Virol (2015) 69:110–6. doi:10.1016/j.jcv.2015.06.078
Keywords: kidney transplantation, infection, immune monitoring, functional, prediction
Citation: Fernández-Ruiz M, Ruiz-Merlo T, Rodríguez-Goncer I, Caso JM, López-Medrano F, Parra P, San Juan R, Polanco N, González E, Andrés A, Aguado JM and Redondo N (2024) Performance of a Global Functional Assay Based on Interferon-γ Release to Predict Infectious Complications and Cancer After Kidney Transplantation. Transpl Int 37:13551. doi: 10.3389/ti.2024.13551
Received: 17 July 2024; Accepted: 22 October 2024;
Published: 30 October 2024.
Copyright © 2024 Fernández-Ruiz, Ruiz-Merlo, Rodríguez-Goncer, Caso, López-Medrano, Parra, San Juan, Polanco, González, Andrés, Aguado and Redondo. This is an open-access article distributed under the terms of the Creative Commons Attribution License (CC BY). The use, distribution or reproduction in other forums is permitted, provided the original author(s) and the copyright owner(s) are credited and that the original publication in this journal is cited, in accordance with accepted academic practice. No use, distribution or reproduction is permitted which does not comply with these terms.
*Correspondence: Mario Fernández-Ruiz, bWFyaW9fZmRlenJ1aXpAeWFob28uZXM=
†ORCID: Mario Fernández-Ruiz, orcid.org/0000-0002-0315-8001; Tamara Ruiz-Merlo, orcid.org/0000-0002-8261-6057; Isabel Rodríguez-Goncer, orcid.org/0000-0003-2150-5748; José María Caso, orcid.org/0000-0001-5087-0299; Francisco López-Medrano, orcid.org/0000-0001-5333-7529; Patricia Parra, orcid.org/0000-0002-5852-5884; Rafael San-Juan, orcid.org/0000-0003-3446-1991; Amado Andrés, orcid.org/0000-0003-0238-1364; José María Aguado, orcid.org/0000-0002-9520-8255; Natalia Redondo, orcid.org/0000-0001-9356-8102