- 1School of Food and Bioengineering, Chengdu University, Chengdu, China
- 2Food Safety Detection Key Laboratory of Sichuan, Technical Center of Chengdu Customs, Chengdu, China
- 3School of Environment and Energy, Peking University Shenzhen Graduate School, Shenzhen, China
Members of the families Thermosynechococcaceae and Thermostichaceae are well-known unicellular thermophilic cyanobacteria and a non-thermophilic genus Pseudocalidococcus was newly classified into the former. Analysis of the codon usage bias (CUB) of cyanobacterial species inhabiting different thermal and non-thermal niches will benefit the understanding of their genetic and evolutionary characteristics. Herein, the CUB and codon context patterns of protein-coding genes were systematically analyzed and compared between members of the two families. Overall, the nucleotide composition and CUB indices were found to differ between thermophiles and non-thermophiles. The thermophiles showed a higher G/C content in the codon base composition and tended to end with G/C compared to the non-thermophiles. Correlation analysis indicated significant associations between codon base composition and CUB indices. The results of the effective number of codons, parity-rule 2, neutral and correspondence analyses indicated that mutational pressure and natural selection primarily account for CUB in these cyanobacterial species, but the primary driving forces exhibit variation among genera. Moreover, the optimal codons identified based on relative synonymous codon usage values were found to differ among genera and even within genera. In addition, codon context pattern analysis revealed the specificity of the sequence context of start and stop codons among genera. Intriguingly, the clustering of codon context patterns appeared to be more related to thermotolerance than to phylogenomic relationships. In conclusion, this study facilitates the understanding of the characteristics and sources of variation of CUB and the evolution of the surveyed cyanobacterial clades with different thermotolerance and provides insights into their adaptation to different environments.
Introduction
Codons (64 nucleotide triplets) have been deciphered as the genetic code of the DNA in organisms (Koonin and Novozhilov, 2009). The standard amino acids are encoded by sixty-one codons, and the remaining three codons represent translation termination signals. An amino acid can be encoded by several synonymous codons due to the degeneracy of the genetic code (Yu et al., 2021), with the exception of tryptophan and methionine. Nevertheless, it is known that certain synonymous codons are preferentially used to encode an amino acid (Yang et al., 2021). Codon usage bias (CUB) is used to describe such variable usage frequencies of synonymous codons and has been widely observed across species in microbial organisms (Arella et al., 2021). Codon usage (CU) bias exists among specific genes and genomes. Although synonymous or silent mutations have no functional consequences, they cause synonymous codon variations in genomes during evolution. Therefore, CUB happens because of biased mutational patterns, whereby some codons may be more susceptible to mutation than others and sustained by selection (Bailey et al., 2021; Parvathy et al., 2022). In addition, CUB could be influenced by GC heterogeneity and GC-biased gene conversion (gBGC) (Dilucca et al., 2021). Thus, the evolution of synonymous CU represents a balance between genetic drift, mutation, and natural selection that results in the translational efficiency of genes, which could be considerably responsible for genome evolution (Mazumdar et al., 2017; Yao et al., 2019). The mutational mechanism speculates that codon bias is caused by nucleotide biases generated by point mutations, or in the rates or repairs of point mutations and is responsible for the interspecific variation of CU (Wang et al., 2011; Gómez et al., 2020). Natural selection and evolution promote or suppress synonymous mutations that affect the adaptability of an organism, leading to CU variation across a genome or a gene (Krasovec and Filatov, 2019; Parvathy et al., 2022).
Codon biases are considered to be associated with extensive broad processes. Codon bias can determine transcription levels by influencing chromatin structure and mRNA folding (Kokate et al., 2021). It can also affect translation efficiency by regulating the elongation rate of translation (Liu, 2020). Thus, codon bias can result from genomic adaptation to transcription and/or translation or adaptation alone (Biswas et al., 2019). In light of similar patterns of CU among closely related organisms, codon bias analysis can unravel horizontal gene transfer (HGT) and evolutionary relationships between organisms (Parvathy et al., 2022). Moreover, the majority of proteins with high expression levels are encoded by genes composed of optimal codons (Frumkin et al., 2018). Thus, codon optimization can be applied to improve heterologous gene expression in transgenic studies.
Members of the family Thermosynechococcaceae and the family Thermostichaceae are known as unicellular thermophilic Synechococcus-like cyanobacterial strains. They are essential components of high-temperature microbial mats and major producers of geothermal habitats and have been discovered to be cosmopolitan in diverse thermal environments (Tang et al., 2018a; Tang et al., 2018b; Alcorta et al., 2020; Prondzinsky et al., 2021). The family Thermosynechococcaceae represents a distinctive cyanobacterial clade characterized by solitary cells and very rarely short filaments (composed of 2–4 connected cells) (Komárek et al., 2020). Initially, Thermosynechococcus was the only described genus within this family (Katoh et al., 2001). Recently, another thermophilic genus, Parathermosynechococcus (Tang et al., 2024b) which was previously regarded as a member of Thermosynechococcus, has been identified. More intriguingly, several Synechococcus-like strains recovered from freshwater lakes were newly classified into the family as the genus Pseudocalidococcus (Luz et al., 2023). Such habitat distribution and genus etymology indicate that Pseudocalidococcus is not thermophilic. In contrast to the recently diverged Thermosynechococcaceae clade (Shih et al., 2017), Thermostichaceae is regarded as early-branching/early-divergent Synechococcus, closer to the root of the cyanobacterial phylogram, typically Gloeobacter (Blank and Sanchez Baracaldo, 2010). This clade represented by the single genus Thermostichus is the most thermophilic cyanobacteria described to date (Kees et al., 2022).
The thermophilic cyanobacteria of the two families naturally thrive in selective environments characterized by extremes of pH, temperature, etc., (Kees et al., 2022; Pierpont, 2022; Tang et al., 2022c). It is well known that the association of these factors is deleterious to the majority of organisms and could be hypothesized to be the main driving force to shape these evolutionarily adapted thermophilic cyanobacteria. Although previous studies have been carried out on CU in certain cyanobacterial genomes (Prabha et al., 2012; Yu et al., 2012; Xu et al., 2013; Prabha et al., 2017), a comprehensive genome-wide profile representing the taxonomic group of thermophilic cyanobacteria is still lacking in understanding the genetic characteristics of codon bias in thermophilic species. The analysis of CU in genomes of cyanobacterial species inhabiting different thermal and non-thermal niches will thus provide insights into their adaptation to distinct environments and evolutionary diversification. Therefore, the investigation of the base bias of members from the families Thermosynechococcaceae and Thermostichaceae will benefit the understanding of their genetic and evolutionary characteristics.
Herein, the CUB and codon context patterns of genomic CDSs were systematically characterized and compared among members of the families Thermosynechococcaceae and Thermostichaceae. These results revealed features and sources of variation of CUB and phylogenetic relationships concerning their lineages. In addition, CU analysis may be useful in applications of improving gene expression efficiency in genetic transformation research through codon optimization.
Materials and methods
Dataset construction
Following the taxonomy of the families Thermosynechococcaceae and Thermostichaceae in the literature (Komárek et al., 2020; Luz et al., 2023; Tang et al., 2024b), representatives were first selected based on genome availability and genome quality (near completeness, >95%; low contamination, <2%; number of ambiguous bases) as previously described (Tang et al., 2022b). Furthermore, genome dereplication was performed using dRep v2.3.2 (Olm et al., 2017) with default settings to eliminate overrepresented species. Only one representative from each species was retained based on the score generated by dRep. Finally, a dataset of 19 genomes was established (Table 1). The genomes of the surveyed cyanobacterial species were retrieved from the NCBI genome database. To avoid gene annotation bias, all the genomes were annotated using the RAST annotation system (Brettin et al., 2015).
CU and CUB indices
A total of 10 indices were determined to evaluate the CU and CUB in each genome used. Excluding Met, Trp, and termination codons, the frequency of guanine and cytosine at the third synonymous position was evaluated by the GC3s parameter (Huo et al., 2021). Hydrophobic (positive values) and hydrophilic (negative values) proteins were represented by general average hydropathicity (GRAVY) values ranging from −2 to 2, respectively. The frequency of aromatic amino acids was represented by the aromaticity (AROMO) value. The RSCU (Relative Synonymous Codon Usage) values > 1 and <1 suggested positive codon bias and negative codon bias, respectively, whereas a random or equal CU was suggested when the RSCU value was equal to 1 (Franzo et al., 2021). The codon adaptation index (CAI) refers to the relative adaptation of the CU of a gene to the CU of highly expressed genes. CAI values range between 0 and 1.0, with higher values representing greater codon usage bias (Sharp and Li, 1987). The Codon Bias Index (CBI) quantifies the degree to which preferred codons are utilized in genetic sequences (Bahiri-Elitzur and Tuller, 2021). The frequency of optimal codons (FOP) was evaluated as described (Li et al., 2023). The values of ENC (Effective Number of Codons) estimated the codon bias for each gene, varying from 20 to 61. Only one codon was used for the amino acid in genes when the ENC value was equal to 20, while 61 suggested no CU preference (Sharp and Li, 1987). In the case of an ENC value <36, the gene was susceptible to strong CU preference (Novembre, 2002). All the indices mentioned above were calculated using CodonW1.4.21 and the CAIcal server (Puigbò et al., 2008).
Analysis of CUB sources
The neutrality plot was illustrated using GC12, which represents the average ratio of GC content in the first (GC1) and second positions (GC2) of the codons, and GC3, which represents the GC content in the third position. If a statistically strong correlation was found between GC12 and GC3, mutational pressure was the dominant driving force. Conversely, natural selection is the major driving force (Parvathy et al., 2022).
The ENC vs. GC3s was plotted to estimate whether the CU of a specific gene is influenced only by mutation or also by other factors, e.g., natural selection. Further, the expected curve on the ENC-GC3s plot was calculated using the equation below. If the corresponding point was distributed around the expected curve, mutational pressure was concluded to be the independent force in the formation of codon bias. Otherwise, certain other factors, e.g., natural selection, played a key role in the formation of codon bias (Sueoka, 1988).
The ENCRatio index was further calculated to represent the variations between the expected value and the actual value of ENC using the following equation.
The frequency of each nucleotide at the third position of the codon (A3, U3, G3, and C3) was collected to plot the Parity Rule 2 bias (PR2-Bias) using the following data: A3/(A3 + U3) vs. G3/(G3 + C3) (Wang et al., 2016).
Correspondence analysis of CU
The multivariate statistical analysis of CU patterns was determined by correspondence analysis (COA). Excluding the unique Met and Trp codons from the 61 codons, the genes comprise 59 sense codons. All the genes were placed into a 59-dimensional hyperspace in the plot. The method indicates the prevailing trend of CU variation in the CDS of the genomes and allocates codons along the axis based on the RSCU value.
Optimal codon identification
According to the CAI values, the top and bottom 5% of all the surveyed genes were refined to generate a high and low-expression gene dataset for each genome. The D-value between the mean RSCU for each codon of the two datasets (ΔRSCU) was calculated to define codons with ΔRSCU greater than 0.08 as high expression. Codons with RSCU values >1 were considered high-frequency codons. The optimal codon was defined as a codon with ΔRSCU >0.08 and RSCU >1.
Codon context analysis
All adjacent codon pairs were quantified and subjected to statistical analysis using the residual analysis tool in Anaconda V2.0 software (Moura et al., 2007). The clustering of codon context patterns was depicted using Anaconda.
Phylogenetic analysis
The phylogenetic inference of the surveyed cyanobacterial species was constructed and further compared based on CU and sequences of single-copy bacterial genes respectively. The hierarchical clustering based on the RSCU values was performed using SPSS v19.0 software. The phylogenetic relationship was reconstructed based on the concatenated sequences of the 120 single-copy genes generated from the GTDB-tk analysis (Chaumeil et al., 2020). Multisequence alignment and ML phylogenetic inference were conducted using MAFFT v7.453 (Katoh and Standley, 2013) and IQ-TREE v2.1.3 (Minh et al., 2020), respectively. Model selection and parameter setting in IQ-TREE and bootstrap analysis were carried out as described (Tang et al., 2022a).
Results
Nucleotide composition
Detailed information regarding genome characteristics and ecology of cyanobacterial species from the families Thermosynechococcaceae and Thermostichaceae is summarized in Table 1. The genomes studied showed distinct base compositions among the four cyanobacterial genera, while intragenic variation was limited (Table 2). The non-thermophilic Pseudocalidococcus had the lowest contents of GC1, GC2 and GC3, while Thermostichus had the highest. Parathermosynechococcus and Thermosynechococcus showed a similar base composition. The GC3 (48.11%) of Pseudocalidococcus was found to be significantly lower (54.73%–64.06%) than that of the other three thermophilic genera, implying a different preference for the third position of codons. All the genomes exhibited an uneven base composition, suggesting that the codons of the genes in these genomes tend to start with and/or end in G/C. The average GC content was found to be 48.50% for Pseudocalidococcus, 53.50% for Parathermosynechococcus, 53.55% for Thermosynechococcus, and 56.83% for Thermostichus. This result indicates a different overall preference for codons containing G and C among the four genera, particularly between thermophilic species and non-thermophilic species.
The CU and CUB indices are shown in Table 2. Similar to the patterns of base composition, the Pseudocalidococcus had the lowest CAI value (0.20), while Thermostichus had the highest (0.22–0.24). Parathermosynechococcus and Thermosynechococcus had similar CAI values (0.21–0.22). Notably, Pseudocalidococcus had a significantly lower CBI value (−0.05 to −0.04) compared to the other three thermophilic genera (0.00–0.13), indicating different levels of gene expression between thermophiles and non-thermophiles. Similarly, the lowest FOP values (0.38) were observed in Pseudocalidococcus and the highest (0.44–0.48) in Thermostichus. Furthermore, more than 61% of the genes in each genome showed negative GRAVY values, indicating their hydrophilic character, while the remaining genes could be hydrophobic proteins. Interestingly, the same AROMO values (0.08) were found to be present in all the genomes studied. Additionally, Thermostichus had a lower ENC value (47.34 on average) compared to the other three genera (48.35–49.91), showing a different CU preference among the genera. Collectively, these indices reveal different CU patterns especially between thermophilic species and non-thermophilic species.
Correlation of the CU and CUB indices
The results of the correlation analysis of the CU and CUB indices are summarized in Figure 1. In general, GC1 was found to be significantly correlated with GC2, GC3, GC3s and GC content in all four cyanobacterial genera except for Thermostichus sp. M44. GC2 was found to be significantly associated with GC3, GC3s and GC in all genera except for four Thermostichus genomes, while GC3 was significantly related to GC3s and GC in all four genera. The correlations of the other indices were found to be quite variable among the genomes, indicating that CUB is affected by a variety of factors.
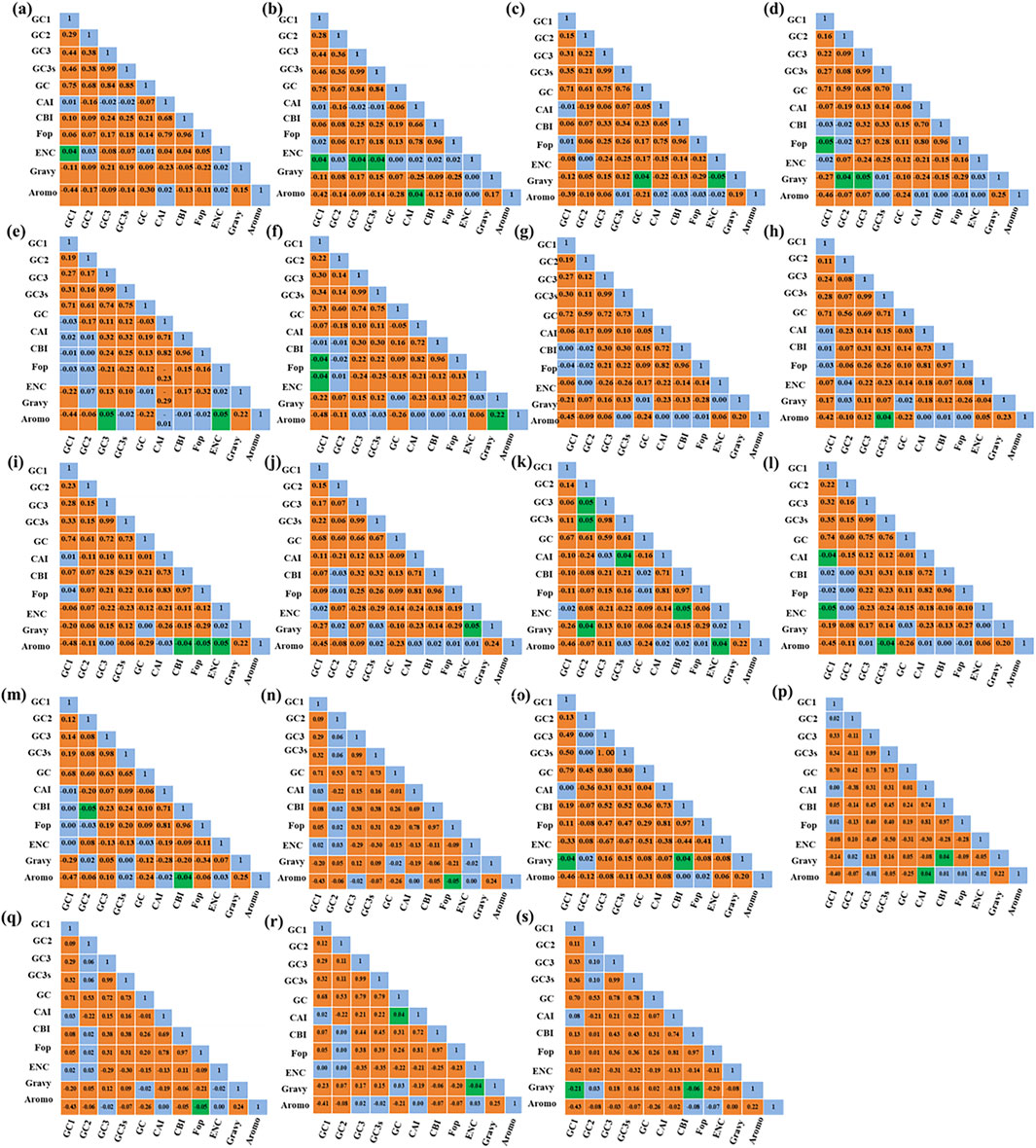
Figure 1. Pearson’s correlation analysis of different CU and CUB indices of the studied cyanobacterial species. Two significance levels were applied, namely 0.05 (green) and 0.01 (orange). (A) Pseudocalidococcus BACA0444; (B) Pseudocalidococcus PCC 6312; (C) Parathermosynechococcus PCC 6715; (D) Thermosynechococcus NK55; (E) Thermosynechococcus E542; (F) Thermosynechococcus CL-1; (G) Thermosynechococcus TA-1; (H) Thermosynechococcus BP-1; (I) Thermosynechococcus HN-54; (J)Thermosynechococcus KatS; (K) Thermosynechococcus M55; (L) Thermosynechococcus PP45; (M) Thermosynechococcus Uc; (N) Thermostichus JA-2-3Ba; (O) Thermostichus JA-3-3Ab; (P) Thermostichus M44; (Q) Thermostichus MAXBIN; (R) Thermostichus Nb3U1; (S) Thermostichus Rupite.
Neutral analysis
A neutrality plot can clarify the impact of mutational pressure and the role of natural selection on CUB. A regression slope of 0, with no significant correlation between GC12 and GC3, indicates complete dependence on natural selection. Conversely, a slope approaching or equal to 1 with a significant correlation suggests that mutational pressure substantially influences the gene (Sueoka, 1988). As shown in Supplementary Table S1, the regression slopes varied from 0.0966 to 0.8388, with R2 values ranging from 0.0053 to 0.2605. Pearson’s correlation analysis identified a significant correlation (P < 0.01) between GC12 and GC3 across the 19 genomes examined. The collective data indicates that mutational pressure predominantly influences CUB in Pseudocalidococcus and Parathermosynechococcus, whereas natural selection is the principal factor driving CUB in Thermosynechococcus. In Thermostichus, CUB is primarily affected by mutational pressure in JA-2-3Ba, JA-3-3Ab, and Nb3U1, while natural selection is the driving force of CUB in the remaining three genomes. These differing influences may shape the evolutionary trajectories of these cyanobacterial genera.
Influence of ENC on CUB
The average ENC values of the four cyanobacterial genera ranged from 47.34 to 49.74 and were found to be all higher than 35, indicating that these genera do not have a strong codon usage preference (Supplementary Figure S1). The ENC-GC3s plots show that the genes of these cyanobacterial species deviate significantly from the expected ENC plot curve. Hence, mutational pressure alone does not account for CUB; other factors, such as natural selection, also contribute significantly to CUB formation. The ENCratio values for these cyanobacteria ranged from 3.18% to 8.49% (Supplementary Figure S2). Furthermore, the ENC values were suggested to be influenced by the GC3s values as per the calculation formula, highlighting the crucial role of GC3s in CUB formation. Thus, the findings confirm that factors beyond mutational pressure, such as natural selection, play a key role in shaping the CUB in these cyanobacterial genera.
Preference for the third codon position
The PR2-bias plot analysis (Supplementary Figure S3) suggests that the genes of the 19 genomes were not uniformly distributed among the four quadrants. The genes of Pseudocalidococcus, Parathermosynechococcus and Thermosynechococcus were found to be predominantly distributed in the quadrants of G3/(G3 + C3) < 0.5 and A3/(A3 + T3) < 0.5, whereas the genes of Thermostichus were found to be predominantly distributed in the quadrants of G3/(G3 + C3) > 0.5 and A3/(A3 + T3) < 0.5. All the results suggest a strong preference for the third base of the codons in these cyanobacterial species. Thus, other factors, such as natural selection, play a key part in the process of CUB in these cyanobacteria.
Correspondence analysis
RSCU values-based correspondence analysis indicates that the four axes contributed the most to the variance, with average contributions of 14.67%, 10.66%, 10.03%, and 9.67%, respectively (Supplementary Figure S4). Among them, axis 1 contributed the most to the variance. The correlation between axis 1 and GC, GC3s, ENC, CAI, CBI and FOP was further analyzed, and the results show that only in Thermostichus (except for Thermostichus MAXBIN) axis 1 significantly correlated (P < 0.01) with all these investigated indicators. In addition, there were large differences among the RSCU values of genes, suggesting that the synonymous CUs of the genes are differentiated.
Optimal codon identification
As shown in Figure 2, the RSCU values for specific codons were found to be different among the genera. The codon CGC encoding arginine (Arg) had a high RSCU value (2.37) and was therefore strongly preferred in the three thermophilic genera. RSCU analysis shows that Pseudocalidococcus contained an average of 25 high-frequency codons (RSCU > 1.0), followed by 23 in Thermosynechococcus, 22 in Thermostichus, and 20 in Parathermosynechococcus (Figure 2). In total, 15 high-frequency codons were found to be common to all the studied cyanobacterial genomes. Of these 15 common codons, 10 end with C/G and five end with T/A, suggesting that these common codons tend to end in G/C. Except for Pseudocalidococcus, intraspecies variation of optimal codons was evident within the other three thermophilic genera (Figure 3). The optimal codons of the thermophilic genera end mainly in G/C, while A/T is preferred in that of the non-thermophilic genera. Among the optimal codons, ACC was found to be the most frequently used, followed by CTG, AGC, GCC, and CGC. In addition, genus-specific optimal codons were present, such as AAA and GTT in Pseudocalidococcus.
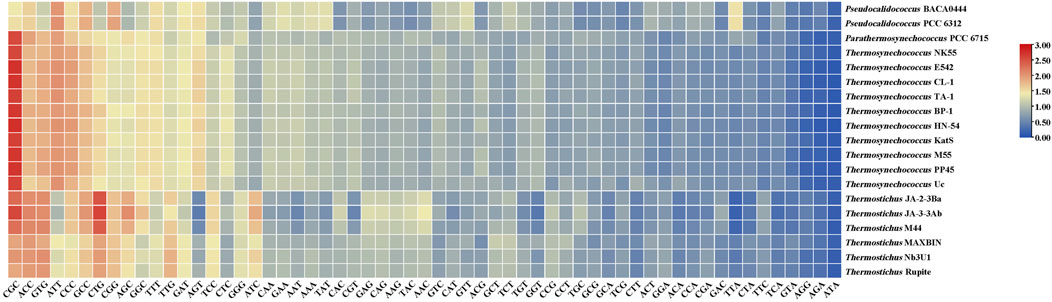
Figure 2. The RSCU values of the genomes of the studied cyanobacterial species. A gradient from blue to red indicates that the average RSCU value of the codon is from low to high.
Codon context pattern
The type of codon pair identified was 3,904, 3,900, 3,898, and 3,899 on average in Pseudocalidococcus, Parathermosynechococcus, Thermosynechococcus, and Thermostichus, respectively (Supplementary Table S2). The most used codon pair differed among the four genera, namely CAG-GCC in Pseudocalidococcus, GAU-CGC in Parathermosynechococcus and Thermosynechococcus, and CUG-CUG in Thermostichus. The top 10 of the most used codon pairs also differed among genera. All codons in each genome were arranged into a 64 × 64 matrix, where each codon was paired with 63 other codons (Supplementary Figure S5). These findings reveal different codon context patterns among these cyanobacterial genera. Moreover, the co-codon context results for the 3′ sequence of the start codon and the 5′ sequence of the stop codon indicate that specific sequences are often used as contexts for the start and stop codons of these cyanobacterial species (Supplementary Table S3). First, GCC or CCC is the most common codon in the 5′-context of stop codons UAA and UAG for the vast majority of the species, while the 5′-codon of the stop codon UGA varies among species. Second, the 5′- context most frequently avoided by stop codons varies considerably among species. Third, GUG and GCC are respectively the most common 3′-contexts of the start codon AUG for Pseudocalidococcus and Thermostichus and Parathermosynechococcus and Thermosynechococcus, whereas the UAA and UGA codons are the most frequently avoided 3′-contexts for AUG. All the results suggest a degree of non-random use of start and stop signal codon settings in these cyanobacterial species.
Phylogenetic relationship
The ML phylogenomic tree of the cyanobacterial species (Figure 4A) was found to be similar to the clustering inferred based on the RSCU values (Figure 4B). Both topologies indicate that Parathermosynechococcus and Thermosynechococcus are more closely related genera, from which Pseudocalidococcus was divergent within the family Thermosynechococcaceae. Intriguingly, the clustering inferred by codon context patterns (Figure 4C) was different from the other two phylograms. The Pseudocalidococcus is even more divergent from Parathermosynechococcus and Thermosynechococcus than Thermostichus. Thus, codon context patterns may be more related to thermotolerance than to phylogenetic relationships.
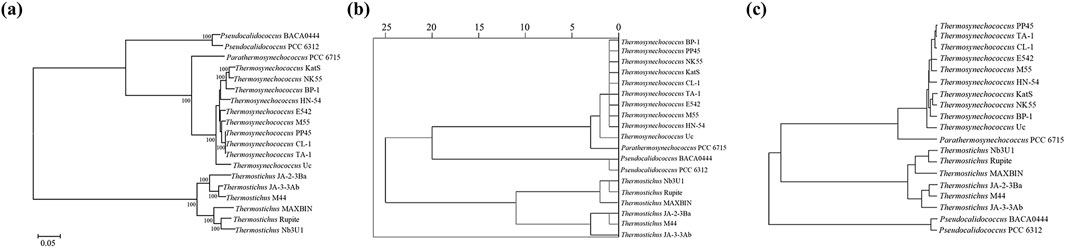
Figure 4. Relationship inferences of the studied cyanobacterial species. (A) Phylogenomic tree based on multiple sequence alignment of 120 bacterial marker genes; (B) hierarchical clustering generated from the RSCU values; (C) clustering inferred from codon context patterns.
Discussion
Genetic codons are a core component that is associated with genetic material, amino acids, and proteins in organisms. Researching CUB will provide reliable information for the investigation of genetic structure and evolutionary trends, along with protein expression and related functions (Wang et al., 2018; Chakraborty et al., 2020). Numerous biological factors have been proven to affect CUB. Among them, mutation and natural selection are the primary factors influencing CUB (Sundararajan et al., 2016; Meyer, 2021). Recently, the availability of more and more cyanobacterial genomes has facilitated the rapid investigation of the molecular components of various systems and the structural traits from a genome perspective (Tang et al., 2023a; Tang et al., 2023b; Tang et al., 2024a). Nevertheless, the characteristics of CUB for the genomes of thermophilic cyanobacteria and the closely related non-thermophilic cyanobacteria have not been fully understood.
The current study comprehensively analyzed the characteristics and sources of variation of CUB and the phylogenetic relationships of the 19 genomes from four cyanobacterial genera. Nucleotide composition showed distinct patterns between non-thermophiles and thermophiles (Table 2). In addition, the most thermophilic cyanobacterium Thermostichus exhibited a different nucleotide composition than the thermophilic cyanobacteria Parathermosynechococcus and Thermosynechococcus. The latter always showed similar patterns of CU and CUB indices. These findings indicate that the CU and CUB appear to be related to thermotolerance. The genes of the three thermophilic genera tend to end in G/C, while those of Pseudocalidococcus usually end in A/T. This observation is consistent with previous findings that codons ending in A and/or T are commonly used in the genomes of marine and freshwater cyanobacteria (Yu et al., 2012) and that higher genomic GC content is important for thermophilic organisms to maintain nucleic acid structure (Musto et al., 2006). Moreover, this suggests that factors in different life modes may influence the pattern of synonymous codon usage in cyanobacterial genomes (Rajneesh et al., 2017).
Significant correlations are present between codon base composition and CAI, CBI and FOP (Figure 1), suggesting that base composition affects CUB. In addition, as shown by the results of RSCU analysis (Figure 2), both high-frequency codons and optimal codons prefer to end in G/C, which further demonstrates the base preference of the surveyed cyanobacterial species at the third position of the codon. The CUB among the four genera are distinct based on the RSCU values suggests that Parathermosynechococcus and Thermosynechococcus have similar pattern of CUB, which is distinct from that of the other genera. Since the two genera are phylogenetically close (Figure 4A), the data presented here is in line with the proposal that genetically related species usually have very analogous CUB (Sharp et al., 1988). Furthermore, different genera vary in the types of codons identified as both high-frequency codons and optimal codons. Thus, this finding could serve as a benchmark for optimizing codons for heterologous expression.
Regarding the origin of codon variation, the results of the ENC-GC3s and PR2 plot analysis (Supplementary Figures S1, S3) suggest that both mutational pressure and natural selection significantly influence CUB in these cyanobacterial species. The results of neutrality analyses (Supplementary Table S1) suggest that the primary driving force of CUB differs among cyanobacterial species, which may result in different modulation of codon usage for environmental adaptation of microorganisms (Arella et al., 2021). The observed divergence in the driving force within Thermostichus may be attributed to niche temperature, which can dramatically vary from 50 to 72°C among Thermostichus strains (Pierpont et al., 2024). The strength of the driving force may also impact the strength of the preference (Xu et al., 2024). It is noteworthy that although the methods here have been widely used in CUB studies, they are based on the assumption of strand symmetry in composition and mutation (Sueoka, 1995). Future work could take into account context-dependent mutational dynamics, which use different assumptions to examine how the context-dependence of mutations affects CUB (Morton, 2022).
Codon context refers to the representation of the arrangement of consecutive codon pairs, revealing the preference for these pairs within an organism (Parvathy et al., 2022). This preference may be conserved or genus/species-specific. The codon pair pattern (Supplementary Table S2) is considerably distinguishable among the four genera. Furthermore, specific codon sequences are preferentially used in the 3′ and 5′ contexts of the start codon and the stop codons, respectively (Supplementary Table S3). The biased usage of the sequence context of start and stop codons may affect the initiation and termination of gene translation (Behura and Severson, 2012; Krafczyk et al., 2021). Moreover, mostly homogeneous codon pairs are ubiquitously frequent in these cyanobacterial species (Supplementary Figure S5). From an economic perspective, using a homogeneous codon context during translation may be energetically less expensive than using a context with distinct codon sequences corresponding to ribosomal A and P sites (Behura and Severson, 2012). Interestingly, the codon pair pattern classified Pseudocalidococcus as the most divergent group (Figure 4C) from the other three thermophilic genera, which is entirely different from the phylogenomic relationship (Figure 4A). This is consistent with previous observations that phenotypic traits, rather than phylogenetic relatedness, underlie similarities in CUB among organisms (Botzman and Margalit, 2011). Indeed, it has been shown that species with given phenotypic traits and living in similar environmental conditions, e.g., thermal niches versus non-thermal niches here, show similar codon preferences, indicating an evolutionary convergence of CUB and adaptation in groups of organisms sharing similar physiology and/or living in similar habitats (Masłowska-Górnicz et al., 2022). Several processes, e.g., lateral gene transfer, have been suggested to contribute to the convergence of codon adaptation to environmental parameters (like pressure, salinity and temperature) (Carbone et al., 2005).
Noteworthy is the significant difference in genome size between thermophiles and non-thermophiles or among thermophiles (Table 1). However, genome size is considered to either play a subsidiary role or to rely indirectly on different mutator genes to fine-tune the GC content (Wu et al., 2012). Thus, it is difficult to predict a priori selection on CUB based on genome size (LaBella et al., 2019). In addition, although CU can vary not only between organisms but also within different regions of a genome and even within a gene (Hooper and Berg, 2000), it is known that a specific codon usage characterizes each bacterial species and that the majority of its genes share such a bias (Plotkin and Kudla, 2011), indicating that the whole genome, rather than individual genes, is the unit of selection. Moreover, it has been suggested that codon bias in specific categories of genes is a re-modulation of the different CUB of the species (Dilucca et al., 2015).
In conclusion, this study revealed that CUB is a crucial characteristic of genome evolution in cyanobacterial species. The information obtained from this study may be helpful for a better understanding of translational selection between non-thermophiles and thermophiles and between Thermosynechococcaceae and Thermostichaceae. Moreover, transgenic technology is expected in the future to explore genes with underlying important traits in Thermosynechococcaceae and Thermostichaceae species, further facilitating in-depth studies on the biotechnological potentials of these cyanobacterial species.
Data availability statement
The data presented in this study are openly available in the National Center for Biotechnology Information https://www.ncbi.nlm.nih.gov/genome/.
Author contributions
JT and JZ designed the research study. Q-HM and ZH performed the research and analyzed the data. MD provided help and advice on the analysis. Q-HM and JT wrote the manuscript. JZ and MD reviewed and edited the manuscript. All authors contributed to the article and approved the submitted version.
Funding
The author(s) declare that financial support was received for the research, authorship, and/or publication of this article. Supported by the National Natural Science Foundation of China (31970092, 32071480, and 32250610204), Chengdu Customs Technology Center (2024TCKY01), and Tenure-Track Fund to M.D.
Conflict of interest
The authors declare that the research was conducted in the absence of any commercial or financial relationships that could be construed as a potential conflict of interest.
Supplementary material
The Supplementary Material for this article can be found online at: https://www.frontierspartnerships.org/articles/10.3389/abp.2024.13825/full#supplementary-material
Footnotes
1https://codonw.sourceforge.net/
References
Alcorta, J., Alarcón-Schumacher, T., Salgado, O., and Díez, B. (2020). Taxonomic novelty and distinctive genomic features of hot spring cyanobacteria. Front. Genet. 11 (1391), 568223. doi:10.3389/fgene.2020.568223
Arella, D., Dilucca, M., and Giansanti, A. (2021). Codon usage bias and environmental adaptation in microbial organisms. Mol. Genet. Genomics 296 (3), 751–762. doi:10.1007/s00438-021-01771-4
Bahiri-Elitzur, S., and Tuller, T. (2021). Codon-based indices for modeling gene expression and transcript evolution. Comput. Struct. Biotechnol. J. 19, 2646–2663. doi:10.1016/j.csbj.2021.04.042
Bailey, S. F., Alonso Morales, L. A., and Kassen, R. (2021). Effects of synonymous mutations beyond codon bias: The evidence for adaptive synonymous substitutions from microbial evolution experiments. Genome Biol. Evol. 13 (9), evab141. doi:10.1093/gbe/evab141
Behura, S. K., and Severson, D. W. (2012). Comparative analysis of codon usage bias and codon context patterns between dipteran and hymenopteran sequenced genomes. PLoS One 7 (8), e43111. doi:10.1371/journal.pone.0043111
Biswas, K. K., Palchoudhury, S., Chakraborty, P., Bhattacharyya, U. K., Ghosh, D. K., Debnath, P., et al. (2019). Codon usage bias analysis of citrus tristeza virus: Higher codon adaptation to citrus reticulata host. Viruses 11 (4), 331. doi:10.3390/v11040331
Blank, C., and Sanchez Baracaldo, P. (2010). Timing of morphological and ecological innovations in the cyanobacteria–a key to understanding the rise in atmospheric oxygen. Geobiology 8 (1), 1–23. doi:10.1111/j.1472-4669.2009.00220.x
Botzman, M., and Margalit, H. (2011). Variation in global codon usage bias among prokaryotic organisms is associated with their lifestyles. Genome Biol. 12 (10), R109. doi:10.1186/gb-2011-12-10-r109
Brettin, T., Davis, J. J., Disz, T., Edwards, R. A., Gerdes, S., Olsen, G. J., et al. (2015). RASTtk: A modular and extensible implementation of the RAST algorithm for building custom annotation pipelines and annotating batches of genomes. Sci. Rep. 5, 8365. doi:10.1038/srep08365
Carbone, A., Képès, F., and Zinovyev, A. (2005). Codon bias signatures, organization of microorganisms in codon space, and lifestyle. Mol. Biol. Evol. 22 (3), 547–561. doi:10.1093/molbev/msi040
Chakraborty, S., Yengkhom, S., and Uddin, A. (2020). Analysis of codon usage bias of chloroplast genes in Oryza species. Planta 252 (4), 67. doi:10.1007/s00425-020-03470-7
Chaumeil, P.-A., Mussig, A. J., Hugenholtz, P., and Parks, D. H. (2020). GTDB-tk: A toolkit to classify genomes with the genome taxonomy database. Bioinformatics 36 (6), 1925–1927. doi:10.1093/bioinformatics/btz848
Dilucca, M., Cimini, G., Forcelloni, S., and Giansanti, A. (2021). Co-evolution between codon usage and protein-protein interaction in bacteria. Gene 778, 145475. doi:10.1016/j.gene.2021.145475
Dilucca, M., Cimini, G., Semmoloni, A., Deiana, A., and Giansanti, A. (2015). Codon bias patterns of E. coli’s interacting proteins. PLoS One 10 (11), e0142127. doi:10.1371/journal.pone.0142127
Franzo, G., Tucciarone, C. M., Legnardi, M., and Cecchinato, M. (2021). Effect of genome composition and codon bias on infectious bronchitis virus evolution and adaptation to target tissues. BMC Genomics 22 (1), 244. doi:10.1186/s12864-021-07559-5
Frumkin, I., Lajoie, M. J., Gregg, C. J., Hornung, G., Church, G. M., and Pilpel, Y. (2018). Codon usage of highly expressed genes affects proteome-wide translation efficiency. Proc. Natl. Acad. Sci. U. S. A. 115 (21), E4940–E4949. doi:10.1073/pnas.1719375115
Gómez, M. M., de Mello Volotão, E., Assandri, I. R., Peyrou, M., and Cristina, J. (2020). Analysis of codon usage bias in potato virus Y non-recombinant strains. Virus Res. 286, 198077. doi:10.1016/j.virusres.2020.198077
Hooper, S. D., and Berg, O. G. (2000). Gradients in nucleotide and codon usage along Escherichia coli genes. Nucleic Acids Res. 28 (18), 3517–3523. doi:10.1093/nar/28.18.3517
Huo, X., Liu, S., Li, Y., Wei, H., Gao, J., Yan, Y., et al. (2021). Analysis of synonymous codon usage of transcriptome database in Rheum palmatum. PeerJ 9, e10450. doi:10.7717/peerj.10450
Katoh, H., Itoh, S., Shen, J.-R., and Ikeuchi, M. (2001). Functional analysis of psbV and a novel c-type cytochrome gene psbV2 of the thermophilic cyanobacterium Thermosynechococcus elongatus strain BP-1. Plant Cell Physiology 42 (6), 599–607. doi:10.1093/pcp/pce074
Katoh, K., and Standley, D. M. (2013). MAFFT multiple sequence alignment software version 7: Improvements in performance and usability. Mol. Biol. Evol. 30, 772–780. doi:10.1093/molbev/mst010
Kees, E., Murugapiran, S., Bennett, A., and Hamilton, T. (2022). Distribution and genomic variation of thermophilic cyanobacteria in diverse microbial mats at the upper temperature limits of photosynthesis. mSystems 7 (5), e0031722. doi:10.1128/msystems.00317-22
Kokate, P. P., Techtmann, S. M., and Werner, T. (2021). Codon usage bias and dinucleotide preference in 29 Drosophila species. G3 (Bethesda, Md.) 11 (8), jkab191. doi:10.1093/g3journal/jkab191
Komárek, J., Johansen, J., Smarda, J., and Strunecký, O. (2020). Phylogeny and taxonomy of Synechococcus-like cyanobacteria. Fottea 20, 171–191. doi:10.5507/fot.2020.006
Koonin, E. V., and Novozhilov, A. S. (2009). Origin and evolution of the genetic code: the universal enigma. IUBMB Life 61 (2), 99–111. doi:10.1002/iub.146
Krafczyk, R., Qi, F., Sieber, A., Mehler, J., Jung, K., Frishman, D., et al. (2021). Proline codon pair selection determines ribosome pausing strength and translation efficiency in bacteria. Commun. Biol. 4 (1), 589. doi:10.1038/s42003-021-02115-z
Krasovec, M., and Filatov, D. A. (2019). Evolution of codon usage bias in diatoms. Genes 10 (11), 894. doi:10.3390/genes10110894
LaBella, A. L., Opulente, D. A., Steenwyk, J. L., Hittinger, C. T., and Rokas, A. (2019). Variation and selection on codon usage bias across an entire subphylum. PLoS Genet. 15 (7), e1008304. doi:10.1371/journal.pgen.1008304
Li, Q., Luo, Y., Sha, A., Xiao, W., Xiong, Z., Chen, X., et al. (2023). Analysis of synonymous codon usage patterns in mitochondrial genomes of nine Amanita species. Front. Microbiol. 14, 1134228. doi:10.3389/fmicb.2023.1134228
Liu, Y. (2020). A code within the genetic code: Codon usage regulates co-translational protein folding. Cell Commun. Signal. 18 (1), 145. doi:10.1186/s12964-020-00642-6
Luz, R., Cordeiro, R., Kaštovský, J., Fonseca, A., Urbatzka, R., Vasconcelos, V., et al. (2023). Description of Pseudocalidococcus azoricus gen. sp. nov. (Thermosynechococcaceae, Cyanobacteria), a rare but widely distributed coccoid cyanobacteria. Diversity 15 (12), 1157. doi:10.3390/d15121157
Masłowska-Górnicz, A., van den Bosch, M. R. M., Saccenti, E., and Suarez-Diez, M. (2022). A large-scale analysis of codon usage bias in 4868 bacterial genomes shows association of codon adaptation index with GC content, protein functional domains and bacterial phenotypes. Biochimica Biophysica Acta (BBA) - Gene Regul. Mech. 1865 (6), 194826. doi:10.1016/j.bbagrm.2022.194826
Mazumdar, P., Binti Othman, R., Mebus, K., Ramakrishnan, N., and Ann Harikrishna, J. (2017). Codon usage and codon pair patterns in non-grass monocot genomes. Ann. Bot. 120 (6), 893–909. doi:10.1093/aob/mcx112
Meyer, M. M. (2021). Revisiting the relationships between genomic G + C Content, RNA secondary structures, and optimal growth temperature. J. Mol. Evol. 89 (3), 165–171. doi:10.1007/s00239-020-09974-w
Minh, B. Q., Schmidt, H. A., Chernomor, O., Schrempf, D., Woodhams, M. D., von Haeseler, A., et al. (2020). IQ-TREE 2: new models and efficient methods for phylogenetic inference in the genomic era. Mol. Biol. Evol. 37 (5), 1530–1534. doi:10.1093/molbev/msaa015
Morton, B. R. (2022). Context-dependent mutation dynamics, not selection, explains the codon usage bias of most angiosperm chloroplast genes. J. Mol. Evol. 90 (1), 17–29. doi:10.1007/s00239-021-10038-w
Moura, G., Pinheiro, M., Arrais, J., Gomes, A. C., Carreto, L., Freitas, A., et al. (2007). Large scale comparative codon-pair context analysis unveils general rules that fine-tune evolution of mrna primary structure. PLoS One 2 (9), e847. doi:10.1371/journal.pone.0000847
Musto, H., Naya, H., Zavala, A., Romero, H., Alvarez-Valín, F., and Bernardi, G. (2006). Genomic GC level, optimal growth temperature, and genome size in prokaryotes. Biochem. Biophysical Res. Commun. 347 (1), 1–3. doi:10.1016/j.bbrc.2006.06.054
Novembre, J. A. (2002). Accounting for background nucleotide composition when measuring codon usage bias. Mol. Biol. Evol. 19 (8), 1390–1394. doi:10.1093/oxfordjournals.molbev.a004201
Olm, M. R., Brown, C. T., Brooks, B., and Banfield, J. F. (2017). dRep: a tool for fast and accurate genomic comparisons that enables improved genome recovery from metagenomes through de-replication. ISME J. 11 (12), 2864–2868. doi:10.1038/ismej.2017.126
Parvathy, S. T., Udayasuriyan, V., and Bhadana, V. (2022). Codon usage bias. Mol. Biol. Rep. 49 (1), 539–565. doi:10.1007/s11033-021-06749-4
Pierpont, C. (2022). An integrative investigation of the Synechococcus A/B clade during adaptive radiation at the upper thermal limit of phototrophy. Missoula, MT: Master, University of Montana.
Pierpont, C. L., Baroch, J. J., Church, M. J., and Miller, S. R. (2024). Idiosyncratic genome evolution of the thermophilic cyanobacterium Synechococcus at the limits of phototrophy. ISME J. 18 (1), wrae184. doi:10.1093/ismejo/wrae184
Plotkin, J. B., and Kudla, G. (2011). Synonymous but not the same: The causes and consequences of codon bias. Nat. Rev. Genet. 12 (1), 32–42. doi:10.1038/nrg2899
Prabha, R., Singh, D. P., Gupta, S., Farooqi, S., and Rai, A. (2012). Synonymous codon usage in Thermosynechococcus elongatus (cyanobacteria) identifies the factors shaping codon usage variation. Bioinformation 8, 622–628. doi:10.6026/97320630008622
Prabha, R., Singh, D. P., Sinha, S., Ahmad, K., and Rai, A. (2017). Genome-wide comparative analysis of codon usage bias and codon context patterns among cyanobacterial genomes. Mar. Genomics 32, 31–39. doi:10.1016/j.margen.2016.10.001
Prondzinsky, P., Berkemer, S. J., Ward, L. M., and McGlynn, S. E. (2021). The Thermosynechococcus genus: Wide environmental distribution, but a highly conserved genomic core. Microbes Environ. 36 (2), ME20138. doi:10.1264/jsme2.ME20138
Puigbò, P., Bravo, I. G., and Garcia-Vallve, S. (2008). CAIcal: a combined set of tools to assess codon usage adaptation. Biol. Direct 3 (1), 38. doi:10.1186/1745-6150-3-38
Rajneesh, , Pathak, J., Kannaujiya, V. K., Singh, S. P., and Sinha, R. P. (2017). Codon usage analysis of photolyase encoding genes of cyanobacteria inhabiting diverse habitats. 3 Biotech. 7 (3), 192. doi:10.1007/s13205-017-0826-2
Sharp, P. M., Cowe, E., Higgins, D. G., Shields, D. C., Wolfe, K. H., and Wright, F. (1988). Codon usage patterns in Escherichia coli, Bacillus subtilis, Saccharomyces cerevisiae, Schizosaccharomyces pombe, Drosophila melanogaster and Homo sapiens; a review of the considerable within-species diversity. Nucleic Acids Res. 16 (17), 8207–8211. doi:10.1093/nar/16.17.8207
Sharp, P. M., and Li, W. H. (1987). The codon adaptation index--a measure of directional synonymous codon usage bias, and its potential applications. Nucleic Acids Res. 15 (3), 1281–1295. doi:10.1093/nar/15.3.1281
Shih, P. M., Hemp, J., Ward, L. M., Matzke, N. J., and Fischer, W. W. (2017). Crown group Oxyphotobacteria postdate the rise of oxygen. Geobiology 15 (1), 19–29. doi:10.1111/gbi.12200
Sueoka, N. (1988). Directional mutation pressure and neutral molecular evolution. Proc. Natl. Acad. Sci. U. S. A. 85 (8), 2653–2657. doi:10.1073/pnas.85.8.2653
Sueoka, N. (1995). Intrastrand parity rules of DNA base composition and usage biases of synonymous codons. J. Mol. Evol. 40 (3), 318–325. doi:10.1007/BF00163236
Sundararajan, A., Dukowic-Schulze, S., Kwicklis, M., Engstrom, K., Garcia, N., Oviedo, O. J., et al. (2016). Gene evolutionary trajectories and gc patterns driven by recombination in Zea mays. Front. Plant Sci. 7, 01433. doi:10.3389/fpls.2016.01433
Tang, J., Hu, Z., Zhang, J., and Daroch, M. (2024a). Genome-scale identification and comparative analysis of transcription factors in thermophilic cyanobacteria. BMC Genomics 25 (1), 44. doi:10.1186/s12864-024-09969-7
Tang, J., Jiang, D., Luo, Y., Liang, Y., Li, L., Shah, M., et al. (2018a). Potential new genera of cyanobacterial strains isolated from thermal springs of western Sichuan, China. Algal Res. 31, 14–20. doi:10.1016/j.algal.2018.01.008
Tang, J., Jiang, Y., Hu, Z., Zhou, H., You, D., and Daroch, M. (2024b). Genomic and phenotypic characterization of Thermosynechococcus-like strains reveals eight species within the genus Thermosynechococcus and a novel genus Parathermosynechococcus gen. nov. Mol. Phylogenetics Evol. 197, 108094. doi:10.1016/j.ympev.2024.108094
Tang, J., Liang, Y., Jiang, D., Li, L., Luo, Y., Shah, M. M. R., et al. (2018b). Temperature-controlled thermophilic bacterial communities in hot springs of western Sichuan, China. BMC Microbiol. 18 (1), 134. doi:10.1186/s12866-018-1271-z
Tang, J., Shah, M. R., Yao, D., Jiang, Y., Du, L., Zhao, K., et al. (2022a). Polyphasic identification and genomic insights of Leptothermofonsia sichuanensis gen. sp. nov., a novel thermophilic cyanobacteria within Leptolyngbyaceae. Front. Microbiol. 13, 765105. doi:10.3389/fmicb.2022.765105
Tang, J., Yao, D., Zhou, H., Du, L., and Daroch, M. (2022b). Reevaluation of Parasynechococcus-like strains and genomic analysis of their microsatellites and compound microsatellites. Plants 11 (8), 1060. doi:10.3390/plants11081060
Tang, J., Yao, D., Zhou, H., Wang, M., and Daroch, M. (2023a). Distinct molecular patterns of two-component signal transduction systems in thermophilic cyanobacteria as revealed by genomic identification. Biology 12 (2), 271. doi:10.3390/biology12020271
Tang, J., Zhou, H., Yao, D., Du, L., and Daroch, M. (2023b). Characterization of molecular diversity and organization of phycobilisomes in thermophilic cyanobacteria. Int. J. Mol. Sci. 24 (6), 5632. doi:10.3390/ijms24065632
Tang, J., Zhou, H., Yao, D., Riaz, S., You, D., Klepacz-Smółka, A., et al. (2022c). Comparative genomic analysis revealed distinct molecular components and organization of CO2-concentrating mechanism in thermophilic cyanobacteria. Front. Microbiol. 13, 876272. doi:10.3389/fmicb.2022.876272
Wang, B., Yuan, J., Liu, J., Jin, L., and Chen, J.-Q. (2011). Codon usage bias and determining forces in green plant mitochondrial genomes. J. Integr. Plant Biol. 53 (4), 324–334. doi:10.1111/j.1744-7909.2011.01033.x
Wang, H., Liu, S., Zhang, B., and Wei, W. (2016). Analysis of synonymous codon usage bias of zika virus and its adaption to the hosts. PLoS One 11 (11), e0166260. doi:10.1371/journal.pone.0166260
Wang, L., Xing, H., Yuan, Y., Wang, X., Saeed, M., Tao, J., et al. (2018). Genome-wide analysis of codon usage bias in four sequenced cotton species. PLoS One 13 (3), e0194372. doi:10.1371/journal.pone.0194372
Wu, H., Zhang, Z., Hu, S., and Yu, J. (2012). On the molecular mechanism of GC content variation among eubacterial genomes. Biol. Direct 7 (1), 2. doi:10.1186/1745-6150-7-2
Xu, P., Zhang, L., Lu, L., Zhu, Y., Gao, D., and Liu, S. (2024). Patterns in genome-wide codon usage bias in representative species of Lycophytes and Ferns. Genes 15 (7), 887. doi:10.3390/genes15070887
Xu, Y., Ma, P., Shah, P., Rokas, A., Liu, Y., and Johnson, C. (2013). Non-optimal codon usage is a mechanism to achieve circadian clock conditionality. Nature 495, 116–120. doi:10.1038/nature11942
Yang, C., Zhao, Q., Wang, Y., Zhao, J., Qiao, L., Wu, B., et al. (2021). Comparative analysis of genomic and transcriptome sequences reveals divergent patterns of codon bias in wheat and its ancestor species. Front. Genet. 12, 732432. doi:10.3389/fgene.2021.732432
Yao, H., Chen, M., and Tang, Z. (2019). Analysis of synonymous codon usage bias in Flaviviridae virus. BioMed Res. Int. 2019, 1–12. doi:10.1155/2019/5857285
Yu, T., Li, J., Yang, Y., Qi, L., Chen, B., Zhao, F., et al. (2012). Codon usage patterns and adaptive evolution of marine unicellular cyanobacteria Synechococcus and Prochlorococcus. Mol. Phylogenetics Evol. 62 (1), 206–213. doi:10.1016/j.ympev.2011.09.013
Keywords: thermosynechococcaceae, thermostichaceae, codon usage, evolution, natural selection
Citation: Mou Q-H, Hu Z, Zhang J, Daroch M and Tang J (2025) Comparative genomics of thermosynechococcaceae and thermostichaceae: insights into codon usage bias. Acta Biochim. Pol 71:13825. doi: 10.3389/abp.2024.13825
Received: 19 September 2024; Accepted: 20 December 2024;
Published: 08 January 2025.
Edited by:
Grzegorz Wegrzyn, University of Gdansk, PolandReviewed by:
Andrzej Zielezinski, Adam Mickiewicz University, PolandGrzegorz Wegrzyn, University of Gdansk, Poland
Copyright © 2025 Mou, Hu, Zhang, Daroch and Tang. This is an open-access article distributed under the terms of the Creative Commons Attribution License (CC BY). The use, distribution or reproduction in other forums is permitted, provided the original author(s) and the copyright owner(s) are credited and that the original publication in this journal is cited, in accordance with accepted academic practice. No use, distribution or reproduction is permitted which does not comply with these terms.
*Correspondence: Jie Tang, dGFuZ2ppZUBjZHUuZWR1LmNu; Jing Zhang, emplYW5lQDE2My5jb20=