- 1School of Medicine, Quzhou College of Technology, Quzhou, China
- 2Tianjin Key Laboratory on Technologies Enabling Development of Clinical Therapeutics and Diagnostics, School of Pharmacy, Tianjin Medical University, Tianjin, China
Based on the crystal structure of the 3C-like protease/Nsp5 (PDB ID 6W63), virtual hits were screened from a natural product compounds database—containing 407270 natural products—by using the high-throughput virtual screening (HTVS) module of Discovery Studio software, and then filtering by “Lipinski’s rule of five” from the top 20 virtual hits. Two star-hits were selected by CDOCKER results and the protein-ligand interactions with the 3CLpro were analyzed. Finally, a 100 ns molecular dynamics simulation was carried out to verify the stability of the receptor-ligand complexes. We screened potent broad-spectrum non-covalent inhibitors that could bind to the SARS-CoV-2 3CLpro active binding site from the natural product compounds library through HTVS and molecular dynamics simulations methods. The LibDock scores and -CDOCKER energy value of the star-hits were higher than the original ligands (X77) bound to 3CLpro. CNP0348829 and CNP0474002, as star-hits, can bind stably to the active site of 3CLpro, which are promising candidate compounds for the treatment of SARS-CoV-2 and provide a theoretical basis for the development of antiviral drugs. The results of the present study may be useful in the prevention and therapeutic perspectives of COVID-19. However, further in vitro and in vivo validation tests are required in the future.
Introduction
In December 2019, an outbreak of a severe respiratory disease occurred in Wuhan, Hubei Province, China. On 11 February 2020, the World Health Organization announced that the new coronavirus pneumonia was named “COVID-19” (World Health Organization, 2022). The International Committee on Classification of Viruses has named the new coronavirus “SARS-CoV-2” (Wang et al., 2020). It is a zoonotic pathogen belonging to the genus β-coronavirus (β-CoV) of the Coronaviridae subfamily of ortho-coronaviruses. The virus initially attacks the respiratory system and causes flu-like symptoms such as cough and fever, and in severe cases, patients may develop acute respiratory distress syndrome and respiratory failure (Sahebnasagh et al., 2020). In addition, novel coronavirus pneumonia can cause systemic inflammation and acute cardiac damage, leading to arrhythmias, heart failure, and multi-organ dysfunction in critically ill patients (Wang et al., 2020). As of December 2022, over 645 million confirmed cases, and over 6.6 million deaths, have been reported globally.1
Nsp5, sometimes referred to as 3C-like protease (3CLpro) or the major protease, is a cysteine protease that breaks down polyproteins at 11 different locations to produce mature, functional proteins. Viral replication depends on 3CLpro, the inhibition of which limits the synthesis of key enzymes for replication like RNA-dependent RNA polymerase (Ullrich and Nitsche, 2020). Furthermore, as the 3CLpro and spike proteins are independent proteins encoded in different parts of the viral genome, the 3CLpro inhibitor’s antiviral activity would probably not be impacted and it does not trigger mutations in the spike protein, which frequently occur in SARS-CoV-2 variations. Consequently, 3CLpro is a potential target for COVID-19 treatment using small-molecule oral therapies (Huang et al., 2021; Unoh et al., 2022).
Natural products have always played a crucial role in drug development against various diseases. Therefore, traditional herbs from diverse geographical locations and various habitats could be considered as potential sources of new drugs for the treatment of viral infections, including those caused by SARS-CoVs and its emergent mutants. After the outbreak of novel coronavirus pneumonia, the National Health Commission of People’s Republic of China promptly issued the “Treatment Protocol for COVID-19,” which has been updated to the ninth edition on a trial basis as of 15 March 2022. In the course of COVID-19 treatment, Traditional Chinese Medicine (TCM) including “XueBiJing injection,” “TanReQing injection,” “JinHuaQingGan granule,” “XiYanPing injection” and others participated in the whole treatment process and played a significant role (Zhuang et al., 2020). On 31 March 2022, the World Health Organization2 published a report stating that “TCM can effectively treat COVID-19, reduce the conversion of mild and common cases to severe disease, shorten the time of virus clearance, and improve the clinical prognosis of mild and common patients” (WHO Team, 2022), which shows that natural products play a very important role in the prevention and treatment of COVID-19. Therefore, screening for candidates from a natural products library3 is a potential strategy for antiviral drug development.
Materials and methods
High-throughput virtual screening
The structures of compounds were obtained from the Coconut database,3 and the crystal structure of 3CLpro (6W63) was downloaded from the PDB database.4 Discovery Studio software (version 4.0, Biovea Inc., Omaha, NE, USA) was applied to molecule docking. The compounds of the Coconut database were prepared using the “Prepare Ligands” module. After removing crystallographic water molecules and ligands, the “Prepare Protein” module was used to remove the conformation of the target protein and to fill the incomplete amino acid residues. Then, the virtual screening was performed in the “LibDock” module, which is a virtual high-throughput algorithm for docking ligands into the receptor active sites. CDOCKER, as an implementation of a CHARMm based docking tool using a rigid receptor, was used to further molecular docking. Ligand conformations were aligned to receptor interaction sites (hotspots) and the best scoring poses were reported (Wu et al., 2003; Mohan et al., 2015).
Molecular dynamics (MD) simulation
In order to verify the time-dependent stability of ligand-receptor complexes, Desmond_maestro 2020.4 with OPLS_2005 force filed5 tools was used to perform a 100-ns molecular dynamics simulation on the receptor-ligand complexes obtained by virtual screening (Okimoto et al., 2009; Gahlawat et al., 2020). Firstly, in an orthorhombic box, the system was solvated with the SPC water model and neutralized by Cl− or Na+ ions. The system energy was minimized at T = 0 K while the force field could be used to regulate the structure of the molecules, followed by ensuring the system stability by the equilibration processes and heating. Subsequently, MD simulation was carried out under periodic boundary conditions and NPT ensemble at T = 300 K and 1 atm pressure using a Nose–Hoover thermostat and Martyna–Tuckerman–Klein barostat (Li et al., 2019; Srivastava et al., 2021). The parameters, such as root-mean-square-deviation (RMSD) for the receptor and ligand, root-mean-square-fluctuation (RMSF) of the protein and ligand, or numbers of hydrogen bonds, were calculated to evaluate the stability of receptor-ligand complexes. The change in conformation can be analyzed by the change in RMSD, showing whether the equilibrium is reached during the simulation. When the shift between the specific frame and the reference frame is 1–3 Å, it can be considered that the shift of the specific frame is not much different from the reference frame. However, it indicates that a larger conformational change has occurred during the simulation when the shift change is apparent. The backbone RMSD curve represents the RMSD of the protein backbone over time. Lig_fit_Prot curve represents the RMSD of the ligand when the protein-ligand complex is first aligned to the protein backbone and then the RMSD of the heavy atom is measured. It is likely that the ligand has diffused away from its initial binding site if a value significantly larger than the RMSD of the protein is observed. The lig_fit_lig curve represents the RMSD of the ligand when the ligand is aligned in its reference conformation which is used to measure the internal fluctuations of the ligand atoms.
ADMET properties prediction
AdmetSAR26 is a website which allows user to search for ADME/T (Absorption, Distribution, Metabolism, Excretion, and Toxicity including Ames toxicity, carcinogenic properties, acute oral toxicity, and rat acute toxicity) properties profiling by name, CASRN, and similarity search (Yang et al., 2019). At the same time, Swiss-ADME online web server7 was used to predict physicochemical properties, water solubility, pharmacokinetics, lipophilicity, drug-likeness drug-like nature (according to drug-likeness rules: Lipinski, Ghose, Veber, Egan, and Muegge), and medicinal chemistry friendliness (PAINS, Brenk, Lead-likeness, Synthetic accessibility) (Daina et al., 2017).
Results and discussion
Molecular docking results and analysis
With the rapid development of various computer methods such as molecular modeling, molecular docking, and molecular dynamics simulations, modern computer tools provide new strategies to explore the scientific basis of TCM (Jiao et al., 2021). Molecular docking is an established in silico structure-based method widely used in drug discovery. Docking enables the identification of novel compounds of therapeutic interest, predicting ligand-target interactions at the atom level, or delineating structure-activity relationships (SAR), without knowing a priori the chemical structure of other target modulators (Pinzi and Rastelli, 2019).
It has been shown that structural domain III (residues 201-301) of the 3CLpro has catalytic activity (Tahir Ul Qamar et al., 2020). X77 can bind at the active site to inhibit the catalytic activity of the 3CLpro (Gahlawat et al., 2020). The interaction diagram of inhibitor X77 bound to 3CLpro is shown in Figure 1A. X77 bound to 3CLpro by two hydrogen bonds between residues GLY143 and GLU166, Pi-Sulfur interaction with residues MET49, amide-Pi stacked with residues LEU141, and other hydrophobic interactions. Very interestingly, as shown in the middle part in Figure 1A, X77 is docked into the active pocket by an “X” shape.
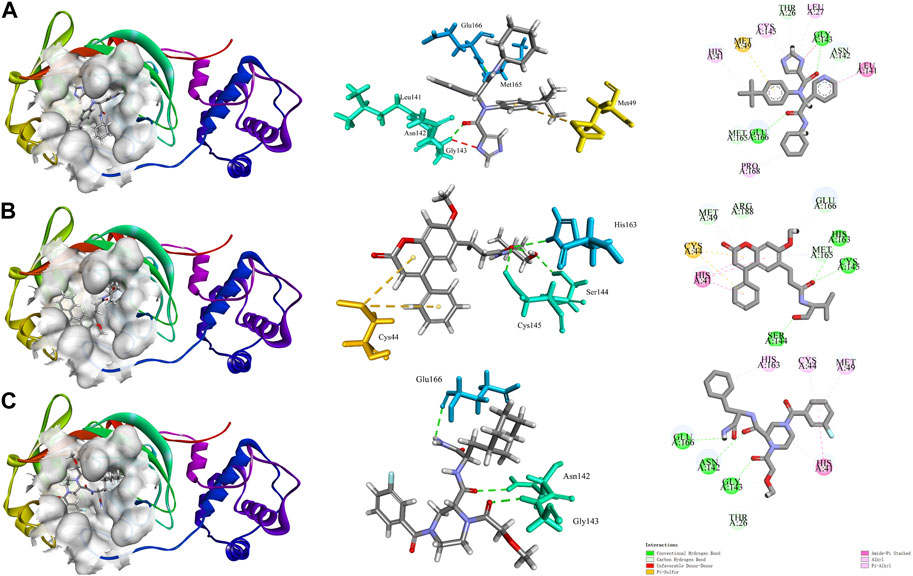
FIGURE 1. Molecular docking diagram of COVID-19 3CLpro bound to X77 and star-hits, generated by Discovery Studio software. (A) Interaction diagram of 3CLpro bound to non-covalent inhibitor X77. (B, C) Action modes of CNP0348829 and CNP0474002 with target 3CLpro respectively.
The top 20 virtual hits with different structural skeletons are listed in Table 1. The binding affinities of all compounds were assessed through LibDock Scores and -CDOCKER energy value. According to the LibDock score results, all compounds had apparent interactions with the target protein. The top 20 virtual hits showed stronger interactions with 3CLpro than the original inhibitor X77. Two -CDOCKER interaction energy values of 38.6734 and 35.6722 kcal/mol, compared to that of X77 (-CDOCKER_ENERGY = 30.4747), were calculated, indicating that CNP0348829 and CNP0474002 are promising candidate compounds for the treatment of SARS-CoV-2. The ADMET property predictions of the virtual hits were demonstrated in Tables 2, 3 in order to investigate how molecules can access the target site of 3CLpro after entering the bloodstream. This analysis is also crucial for analyzing the efficacy of molecules (Fadaka et al., 2022). All parameters were within the Lipinski’s rule of five (ROF) cut-off range for the test compounds except CNP0465303 and CNP0447420 and present no bystander toxicity effects, since managing toxicity is the main task in developing new medications. Ames toxicity, carcinogenic properties, and rat acute toxicity which are important parameters of drug safety, were predicted in the current investigation. According to the results, most of the compounds showed no Ames toxicity, no carcinogenic properties, and low toxicity, meaning they are safe when used as drugs (Fadaka et al., 2021; 2022). The ideal drug molecules fall under such criteria as molecular weight (<500 g/mol), MlogP values (≤4.5), rotatable bonds (≤10), HB acceptors (<10), and number of HB donors (<5) (Lipinski, 2004). We screened out two star-hits based on ROF, while others were eliminated because of the molecular weight or -CDOCKER_ENERGY values.
Interaction diagrams of CNP0348829 and CNP0474002 bound to 3CLpro are shown in Figures 1B, C. As seen in Figure 1B, CNP0348829 was stabilized by three strong hydrogen bond interactions between residues SER144, CYS145, and HIS163. In addition, the ring exhibited very strong Pi–Pi stacked interactions with HIS41, Pi–sulfur interaction with CYS44. CNP0474002-3CLpro bound to 3CLpro by hydrogen bond interactions between C=O of amide with CYS145. The Pi–alkyl stacked interactions between residues MET165 and 18-phenyl and X–sulfur interaction with MET49 like CNP0348829 were also observed (Figure 1C; Table 4). Both CNP0348829 and CNP0474002 bound to the 3CLpro in a spreading conformation, CNP0474002 was docked into the active pocket by an “X” shape, somewhat like X77.
Molecular dynamics simulation results and analysis
To verify the results of molecular docking, molecular dynamics simulations were introduced in this study. Molecular dynamics simulations can show the microscopic evolution of the system at the atomic level and visualize the mechanisms and patterns of experimental phenomena, which is a great advantage in studying the mechanism of ligand-receptor interaction (Adcock and McCammon, 2006).
The change in conformation can be analyzed by the value of RMSD, showing whether the equilibrium is reached during the simulation. When the position-shift between the specific frame and the reference frame is 1–3 Å, it can be considered that the position-shift of the specific frame is not much different from the reference frame. It indicates that a larger conformational change occurred during the simulation when the position-shift change is apparent. The backbone RMSD curve represents the RMSD of the protein backbone over time. Lig_fit_Prot curve represents the RMSD of the ligand when the protein-ligand complex is first aligned to the protein backbone and then the RMSD of the heavy atom is measured. It is likely that the ligand has shifted away from its initial binding site if the value is significantly larger than the RMSD of the protein. The lig_fit_lig curve represents the RMSD of the ligand when the ligand is aligned in its reference conformation which is used to measure the internal fluctuations of the ligand atoms.
The results of molecular dynamics simulations are shown in Figures 2, 3. The major deviation is in RMSD-P, which shows the protein RMSD with a ligand in the active site. The compound CNP0348829 showed initial light fluctuation in RMSD-L, at around 1 Å and 3 Å. However, it showed very strong fluctuations at 52 ns, mainly resulting from the change of conformation at the 12-phenyl, while remaining in the active site of the receptor and stabilized for the remaining period of time in the range of 3.5 Å to 5.5 Å. (Figures 2A, C, D). Unlike CNP0348829, CNP0474002 was stabilized in the range of 3 Å to 5.5 Å at all times under study (Figure 3A). The major initial fluctuation in the 3CLpro was mainly due to C-terminal fluctuations, which was confirmed by the RMSF plot for the protein C (alpha) and backbone, where except for C-terminal, all other residues showed RMSF less than 2.5 Å. Over the period of analysis, C-terminal exhibited RMSF up to 4.5 Å (Figures 2B, 3B). RMSF of the ligand with the protein and the ligand with initial conformation was very stable and was between 1.00 and 2.25 Å and 0.25 to 1.25 Å, respectively (Figure 3C). Protein interactions with the ligands were monitored throughout the simulation, and these interactions can be summarized and classified into four types: hydrogen bonds, hydrophobic interactions, ionic bonds, and water bridges. Among all interactions, hydrogen bonds play an important role in the overall dynamics simulation because of their greater influence on compound specificity, metabolic characteristics, and adsorption parameters (Kruth et al., 2005). The following amino acids, such as HIS41, CYS145, and GLU166 in CNP0474002-3CLpro complex, and CYS44, ASN142, GLY143, SER144, and GLU166 in CNP0348829-3CLpro complex, contribute greatly to the interaction between protein and ligand. These interactions were categorized by type and summarized as shown in Figures 4A, B. The above analysis suggests that the ligand-receptor complexes were maintained well within a relatively stable range with small fluctuations throughout the molecular dynamic simulations, indicating that the binding modes of CNP0348829-3CLpro and CNP0474002-3CLpro complexes are stable during the molecular dynamics simulation.
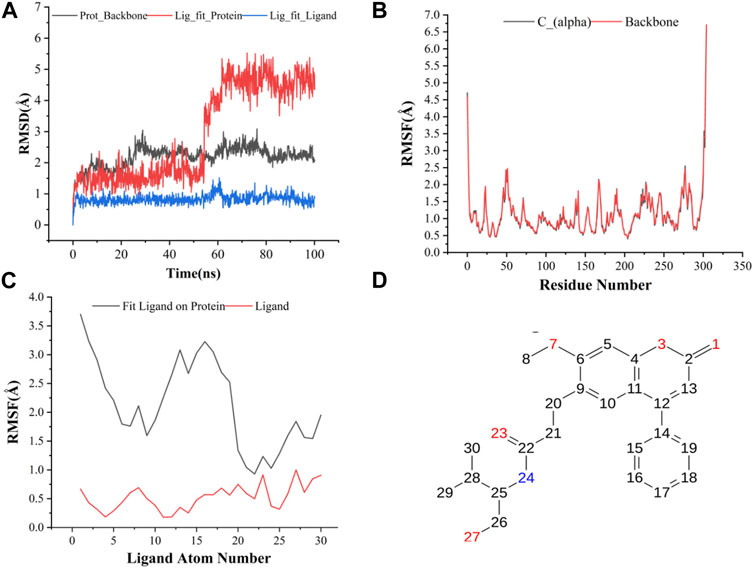
FIGURE 2. (A) RMSD of protein backbone, Lig_fit_Prot and Lig_fit_Lig for CNP0348829-3CLpro complex; (B) RMSF of protein C(alpha) and backbone; (C) RMSF of CNP0348829; (D) The 2D structure of CNP0348829.
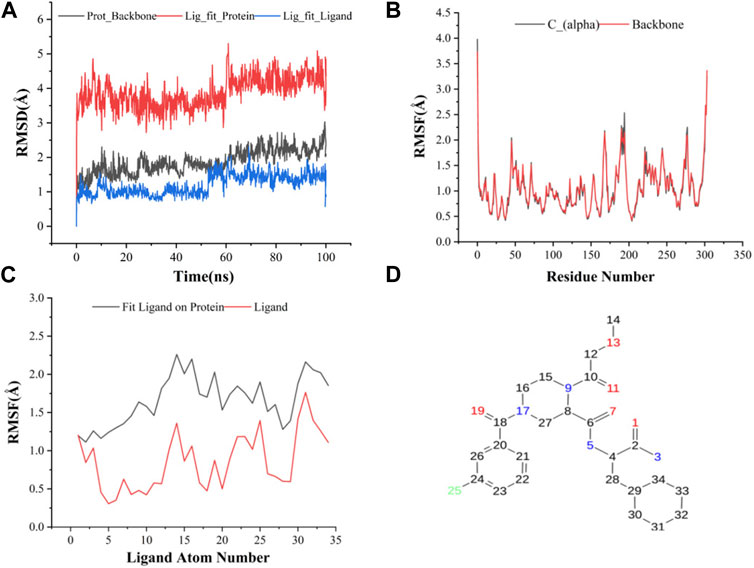
FIGURE 3. (A) RMSD of protein backbone, Lig_fit_Prot and Lig_fit_Lig for CNP0474002-3CLpro complex; (B) RMSF of protein C(alpha) and backbone; (C) RMSF of CNP0474002; (D) The 2D structure of CNP0474002.
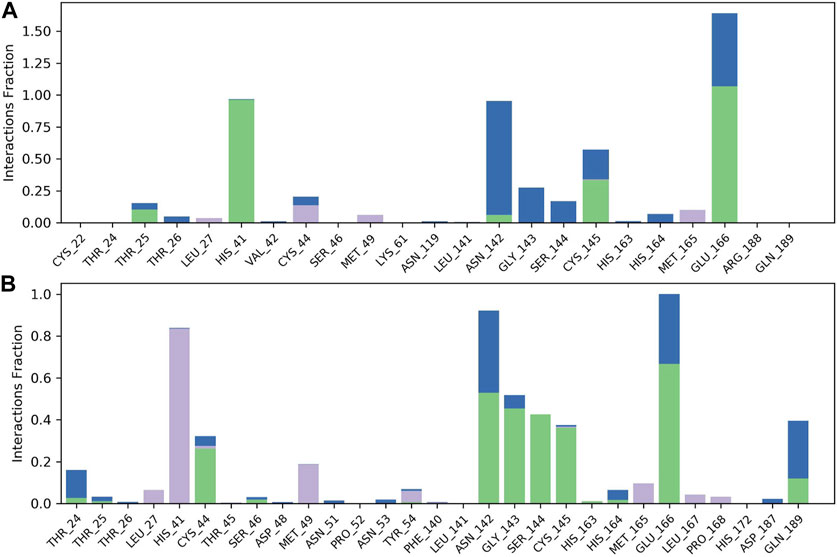
FIGURE 4. Observed CNP0474002-3CLpro and CNP0348829-3CLpro complexes contacts during the 100 ns MD simulation. Interactions include: hydrogen bonds, hydrophobic, and water bridges. Letter codes indicate CNP0474002 (A), CNP0348829 (B).
Discussion
Molecular docking is investigated as a computational tool for virtually screening thousands of drug-like candidates for their affinity toward a specific molecular target, provided that the structure of the target protein is available. Molecular docking serves as the foundation for the structure-based drug design (SBDD) process. It is a fast, cost-effective, and simplified computational tool for predicting the scores associated with the association of putative ligand molecules and the target protein of interest. The obtained docking score offers a basic result for the discovery of leading compounds among the collection and may be taken into consideration for drug development plans (Wang and Zhu, 2016). When combined with results from experimental research, molecular docking-based virtual screening may aid in the process of rational medication design and development (Sethi et al., 2019).
With the thorough virtual screening procedure, molecular dynamics simulation also attracted a lot of attention in the search for potential hit compounds. (Ganesan et al., 2017). The most important feature of using molecular dynamics simulation in drug discovery pipelines is that it offers dynamic structural data on interactions between ligands and target receptors with a collection of complexes conformations (Gioia et al., 2017; Saurabh et al., 2020). According to current trends, molecular docking combined with molecular dynamics simulation is being actively studied to confirm potential interactions between ligand and receptor, which helps develop effective drug candidates.
For many decades, ingredients derived from plants have been thought to be the most explored drug candidates. It is possible to assess the potential use of plant-based metabolites with antiviral activity in the treatment of SARS-CoV-2 infections (Bhuiyan et al., 2020; Kumar Verma et al., 2021). In this context, it is crucial to use computational techniques to discover plant-derived phytochemicals that have an inhibitory effect on SARS-CoV-2 infections. For example, Hesperidin, Theaflavin, Biorobin (Peele et al., 2020); Rutin, (Das et al., 2021); Andrographolide (Enmozhi et al., 2021); Asiatic acid, Avicularin Guaijaverin, Chrysanthemin (Amin et al., 2021); and Hypericin (Pitsillou et al.,2020) have been screened as potential therapeutic drugs for 3CLpro of SARS-CoV-2 by SBDD, and some of which have had protease inhibition activity demonstrated in vitro experiments.
SARS-CoV-3CLpro consists of 306 amino acids and functionally inhibits replicase precursor polyproteins; further preventing COVID-19 gene expression and replication. There are three domains in the structure of 3CLpro, in which 8-101 residues form domain-I, 102-184 residues form domain-II with six-stranded anti-parallel β-barrel, and 201-303 residues form domain-III, which encompasses five a-helices grouped into the anti-parallel globular cluster and is linked to Domain-II by a long loop region (residues 185-200). Firstly, we identified the active pocket of the 3CLpro, which lies in a cleft between Domain-I and II and has a Cys145-His41 catalytic dyad that recognizes P1 Gln and P2 Leu/Met/Phe/Val as substrates, based on the binding site of the known standard inhibitor X77 named N-(4-tert-butylphenyl)-N-[(1R)-2-(cyclohexylamino)-2-oxo-1-(yridine-3-yl)ethyl]-1H-imidazole-4-carbox amide. In this study, we targeted the SARS-CoV-3CLpro; rapidly high-throughput virtual screening was performed to obtain the noncovalent SARS-CoV-2 3CLpro inhibitor from a natural product compounds database that includes 407270 natural products. In the subsequent virtual screening, we used X77 as a positive control and screened star-hits with -CDOCKER scores higher than X77. Amino acids including PHE140, GLN189, GLY143, GLU166, THR190, CYS145, and HIS163 were reported to be crucial in the inhibition process of Mpro through hydrogen bonding, which was also verified in the results of our study (Jin et al., 2020). Gyebi et al. (2021) identified the importance of CYS145 and HIS41 as conserved catalytic dyad residues of SARS-CoV-2 Mpro. Interestingly, compounds CNP0348829 and CNP0474002 interacted with one of the two amino acids by hydrogen bond, and these interactions were also monitored in molecular dynamics simulations for the ligand-receptor complexes. According to the above analysis, we suggest that CNP0348829 and CNP0474002 could be potential inhibitors of 3CLpro. Nevertheless, further experiments are needed for activity validation in the future.
Conclusion
Phytochemical screening in silico is especially alluring since it can digitally screen hundreds of compounds in a certain amount of time and examine the possibility of drug-like molecules. In this study, we performed structure-based virtual screening from a natural products library containing 407270 phytochemicals/traditional Chinese medicinal compounds, based on the crystal structures of the 3CLpro as an attractive target for small-molecule oral therapeutics for treating COVID-19. Through layers of virtual screening, CNP0348829 and CNP0474002, as two selected star-hits, are promising as clinical candidate compounds for the treatment of SARS-CoV-2. Further experimental studies are suggested to check the possible preclinical and clinical efficacy of CNP0348829 and CNP0474002 for the prevention and treatment of COVID-19.
Data availability statement
The original contributions presented in the study are included in the article/supplementary material, further inquiries can be directed to the corresponding author.
Author contributions
All authors listed have made a substantial, direct, and intellectual contribution to the work and approved it for publication.
Conflict of interest
The authors declare that the research was conducted in the absence of any commercial or financial relationships that could be construed as a potential conflict of interest.
Footnotes
1https://www.who.int/publications/m/item/weekly-epidemiological-update-on-covid-19---14-december-2022
2https://www.who.int/publications/m/item/who-expert-meeting-on-evaluation-of-traditional-chinese-medicine-in-the-treatment-of-covid-19
3https://coconut.naturalproducts.net/
5https://www.deshawresearch.com/downloads/download_desmond.cgi/
6http://lmmd.ecust.edu.cn/admetsar2/
References
Adcock, S. A., and McCammon, J. A. (2006). Molecular dynamics: Survey of methods for simulating the activity of proteins. Chem. Rev. 106 (5), 1589–1615. doi:10.1021/cr040426m
Amin, S. A., Ghosh, K., Gayen, S., and Jha, T. (2021). Chemical-informatics approach to COVID-19 drug discovery: Monte Carlo based QSAR, virtual screening and molecular docking study of some in-house molecules as papain-like protease (PLpro) inhibitors. J. Biomol. Struct. Dyn. 39 (13), 4764–4773. doi:10.1080/07391102.2020.1780946
Bhuiyan, F. R., Howlader, S., Raihan, T., and Hasan, M. (2020). Plants metabolites: possibility of natural therapeutics against the COVID-19 pandemic. Front. Med. (Lausanne) 7, 444. doi:10.3389/fmed.2020.00444
Daina, A., Michielin, O., and Zoete, V. (2017). SwissADME: A free web tool to evaluate pharmacokinetics, drug-likeness and medicinal chemistry friendliness of small molecules. Sci. Rep. 7, 42717. doi:10.1038/srep42717
Das, S., Sarmah, S., Lyndem, S., and Singha Roy, A. (2021). An investigation into the identification of potential inhibitors of SARS-CoV-2 main protease using molecular docking study. J. Biomol. Struct. Dyn. 39 (9), 3347–3357. doi:10.1080/07391102.2020.1763201
Enmozhi, S. K., Raja, K., Sebastine, I., and Joseph, J. (2021). Andrographolide as a potential inhibitor of SARS-CoV-2 main protease: An in silico approach. J. Biomol. Struct. Dyn. 39 (9), 3092–3098. doi:10.1080/07391102.2020.1760136
Fadaka, A. O., Aruleba, R. T., Sibuyi, N. R. S., Klein, A., Madiehe, A. M., and Meyer, M. (2022). Inhibitory potential of repurposed drugs against the SARS-CoV-2 main protease: A computational-aided approach. J. Biomol. Struct. Dyn. 40 (8), 3416–3427. doi:10.1080/07391102.2020.1847197
Fadaka, A. O., Sibuyi, N. R. S., Martin, D. R., Klein, A., Madiehe, A., and Meyer, M. (2021). Development of effective therapeutic molecule from natural sources against coronavirus protease. Int. J. Mol. Sci. 22 (17), 9431. doi:10.3390/ijms22179431
Gahlawat, A., Kumar, N., Kumar, R., Sandhu, H., Singh, I. P., Singh, S., et al. (2020). Structure-based virtual screening to discover potential lead molecules for the SARS-CoV-2 main protease. J. Chem. Inf. Model 60 (12), 5781–5793. doi:10.1021/acs.jcim.0c00546
Ganesan, A., Coote, M. L., and Barakat, K. (2017). Molecular dynamics-driven drug discovery: Leaping forward with confidence. Drug Discov. Today 22 (2), 249–269. doi:10.1016/j.drudis.2016.11.001
Gioia, D., Bertazzo, M., Recanatini, M., Masetti, M., and Cavalli, A. (2017). Dynamic docking: a paradigm shift in computational drug discovery. Molecules 22 (11), 2029. doi:10.3390/molecules22112029
Gyebi, G. A., Ogunro, O. B., Adegunloye, A. P., Ogunyemi, O. M., and Afolabi, S. O. (2021). Potential inhibitors of coronavirus 3-chymotrypsin-like protease (3CLpro): An in silico screening of alkaloids and terpenoids from african medicinal plants. J. Biomol. Struct. Dyn. 39 (9), 3396–3408. doi:10.1080/07391102.2020.1764868
Huang, K., Zhang, P., Zhang, Z., Youn, J. Y., Wang, C., Zhang, H., et al. (2021). Traditional Chinese Medicine (TCM) in the treatment of covid-19 and other viral infections: efficacies and mechanisms. Pharmacol. Ther. 225, 107843. doi:10.1016/j.pharmthera.2021.107843
Jiao, X., Jin, X., Ma, Y., Yang, Y., Li, J., Liang, L., et al. (2021). A comprehensive application: molecular docking and network pharmacology for the prediction of bioactive constituents and elucidation of mechanisms of action in component-based Chinese medicine. Comput. Biol. Chem. 90, 107402. doi:10.1016/j.compbiolchem.2020.107402
Jin, Z., Du, X., Xu, Y., Deng, Y., Liu, M., Zhao, Y., et al. (2020). Structure of Mpro from SARS-CoV-2 and discovery of its inhibitors. Nature 582 (7811), 289–293. doi:10.1038/s41586-020-2223-y
Kruth, J., Mercelis, P., Van Vaerenbergh, J., Froyen, L., and Rombouts, M. (2005). Binding mechanisms in selective laser sintering and selective laser melting. Rapid Prototyp. J. 11 (1), 26–36. doi:10.1108/13552540510573365
Kumar Verma, A., Kumar, V., Singh, S., Goswami, B. C., Camps, I., Sekar, A., et al. (2021). Repurposing potential of Ayurvedic medicinal plants derived active principles against SARS-CoV-2 associated target proteins revealed by molecular docking, molecular dynamics and MM-PBSA studies. Biomed. Pharmacother. 137, 111356. doi:10.1016/j.biopha.2021.111356
Li, Z. Y., Su, C. Y., and Ding, B. (2019). Molecular dynamics simulation of β-adrenoceptors and their coupled G proteins. Eur. Rev. Med. Pharmacol. Sci. 23 (14), 6346–6351. doi:10.26355/eurrev_201907_18458
Lipinski, C. A. (2004). Lead- and drug-like compounds: The rule-of-five revolution. Drug Discov. Today Technol. 1 (4), 337–341. doi:10.1016/j.ddtec.2004.11.007
Mandal, A., Jha, A. K., and Hazra, B. (2021). Plant products as inhibitors of coronavirus 3CL protease. Front. Pharmacol. 12, 583387. doi:10.3389/fphar.2021.583387
Mohan, M., James, P., Valsalan, R., and Nazeem, P. A. (2015). Molecular docking studies of phytochemicals from Phyllanthus niruri against Hepatitis B DNA Polymerase. Bioinformation 11 (9), 426–431. doi:10.6026/97320630011426
Okimoto, N., Futatsugi, N., Fuji, H., Suenaga, A., Morimoto, G., Yanai, R., et al. (2009). High-performance drug discovery: Computational screening by combining docking and molecular dynamics simulations. PLoS Comput. Biol. 5 (10), e1000528. doi:10.1371/journal.pcbi.1000528
Peele, K. A., Potla Durthi, C., Srihansa, T., Krupanidhi, S., Ayyagari, V. S., Babu, D. J., et al. (2020). Molecular docking and dynamic simulations for antiviral compounds against SARS-CoV-2: a computational study. Inf. Med. Unlocked 19, 100345. doi:10.1016/j.imu.2020.100345
Pinzi, L., and Rastelli, G. (2019). Molecular docking: shifting paradigms in drug discovery. Int. J. Mol. Sci. 20 (18), 4331. doi:10.3390/ijms20184331
Pitsillou, E., Liang, J., Karagiannis, C., Ververis, K., Darmawan, K. K., Ng, K., et al. (2020). Interaction of small molecules with the SARS-CoV-2 main protease in silico and in vitro validation of potential lead compounds using an enzyme-linked immunosorbent assay. Comput. Biol. Chem. 89, 107408. doi:10.1016/j.compbiolchem.2020.107408
Sahebnasagh, A., Avan, R., Saghafi, F., Mojtahedzadeh, M., Sadremomtaz, A., Arasteh, O., et al. (2020). Pharmacological treatments of COVID-19. Pharmacol. Rep. 72 (6), 1446–1478. doi:10.1007/s43440-020-00152-9
Saurabh, S., Sivakumar, P. M., Perumal, V., et al. (2020). “Molecular dynamics simulations in drug discovery and drug delivery,” in Integrative nanomedicine for new therapies (Berlin, Germany: Springer), 275–301.
Sethi, A., Joshi, K., Sasikala, K., and Alvala, M. (2019). Molecular docking in modern drug discovery: principles and recent applications. Drug Discov. development-new Adv. 2, 1–21. doi:10.5772/intechopen.85991
Srivastava, N., Garg, P., Srivastava, P., and Seth, P. K. (2021). A molecular dynamics simulation study of the ACE2 receptor with screened natural inhibitors to identify novel drug candidate against COVID-19. PeerJ 9, e11171. doi:10.7717/peerj.11171
Tahir Ul Qamar, M., Alqahtani, S. M., Alamri, M. A., and Chen, L. L. (2020). Structural basis of SARS-CoV-2 3CLpro and anti-COVID-19 drug discovery from medicinal plants. J. Pharm. Anal. 10 (4), 313–319. doi:10.1016/j.jpha.2020.03.009
Ullrich, S., and Nitsche, C. (2020). The SARS-CoV-2 main protease as drug target. Bioorg Med. Chem. Lett. 30 (17), 127377. doi:10.1016/j.bmcl.2020.127377
Unoh, Y., Uehara, S., Nakahara, K., Nobori, H., Yamatsu, Y., Yamamoto, S., et al. (2022). Discovery of S-217622, a noncovalent oral SARS-CoV-2 3CL protease inhibitor clinical candidate for treating COVID-19. J. Med. Chem. 65 (9), 6499–6512. doi:10.1021/acs.jmedchem.2c00117
Wang, C., Horby, P. W., Hayden, F. G., and Gao, G. F. (2020). A novel coronavirus outbreak of global health concern. Lancet 395 (10223), 470–473. doi:10.1016/S0140-6736(20)30185-9
Wang, G., and Zhu, W. (2016). Molecular docking for drug discovery and development: A widely used approach but far from perfect. Future Med. Chem. 8 (14), 1707–1710. doi:10.4155/fmc-2016-0143
WHO Team (2022). WHO expert meeting on evaluation of traditional Chinese medicine in the treatment of COVID-19. Geneva: WHO, 4–17.
World Health Organization (2022). Weekly epidemiological update on COVID-19-28 December 2022. [EB/OL]. Available at: https://www.who.int/publications/m/item/weekly-epidemiological-update-on-covid-19---14-december-2022 (Accessed December 14, 2022).
Wu, G., Robertson, D. H., Brooks, C. L., and Vieth, M. (2003). Detailed analysis of grid-based molecular docking: a case study of CDOCKER-A CHARMm-based MD docking algorithm. J. Comput. Chem. 24 (13), 1549–1562. doi:10.1002/jcc.10306
Yang, H., Lou, C., Sun, L., Li, J., Cai, Y., Wang, Z., et al. (2019). admetSAR 2.0: web-service for prediction and optimization of chemical ADMET properties. Bioinformatics 35 (6), 1067–1069. doi:10.1093/bioinformatics/bty707
Keywords: SARS-CoV-2, 3CLpro, natural product compounds, virtual screening, molecular docking
Citation: Gan C, Jia X, Fan S, Wang S, Jing W and Wei X (2023) Virtual screening and molecular dynamics simulation to identify potential SARS-CoV-2 3CLpro inhibitors from a natural product compounds library. Acta Virol. 67:12464. doi: 10.3389/av.2023.12464
Received: 29 March 2023; Accepted: 22 November 2023;
Published: 15 December 2023.
Edited by:
Katarina Polcicova, Slovak Academy of Sciences, SlovakiaCopyright © 2023 Gan, Jia, Fan, Wang, Jing and Wei. This is an open-access article distributed under the terms of the Creative Commons Attribution License (CC BY). The use, distribution or reproduction in other forums is permitted, provided the original author(s) and the copyright owner(s) are credited and that the original publication in this journal is cited, in accordance with accepted academic practice. No use, distribution or reproduction is permitted which does not comply with these terms.
*Correspondence: Xiaopeng Wei, d2VpeGlhb3BlbmdAdG11LmVkdS5jbg==