- 1Department of Clinical Laboratories, Indus Hospital and Health Network, Karachi, Pakistan
- 2Office of Research, Innovation and Commercialization (ORIC), Indus Hospital and Health Network, Karachi, Pakistan
- 3Health Services Academy, Ministry of National Health Services Regulations and Coordination, Islamabad, Pakistan
The epidemic of Monkeypox virus (MPXV), an emerging zoonotic Orthopoxvirus, in beginning OF May 2022, has drawn global attention owing to its increasingly reported cases by the World Health Organization (WHO) in multiple countries. Due to absence of any validated treatments for MPXV infections, the preventive strategies hold significant importance. The current study proposes potential vaccine targets against MPXV by deploying immunoinformatic method. The monkeypox virus encodes 190 different proteins. These proteins were shortlisted on the basis of antigenicity, surface expression, allergenicity, and toxicity. The shortlisted ten proteins were subjected to identification of B- and T-cell epitopes using IEDB, ABCpred, NetMHCpan4.0, and NetMHCIIpan4.0. All the epitopes were further screened for antigenicity, allergenicity, and toxicity properties using VaxiJen, Allertop, and Toxinpred server. All the epitopes have 97.5% worldwide population coverage. Overlapping B-cell, CTL and HTL epitopes were used to design three vaccine construct using suitable linkers and three different adjuvants. Molecular docking was performed for all the three vaccine constructs with TLR4. Based on docking scores and physicochemical profile the vaccine construct V1 with beta defensin adjuvant was selected for further analysis. In silico cloning of V1 into pBAD-DEST49 vector showed maximum expression in bacterial system. Immune simulations also confirmed the high immune responses of V1 within the host cell. The study results may lead towards identification of clinically effective vaccines against MPXV with better safety and potency profile.
Introduction
Monkeypox, a zoonotic viral disease caused by the Monkeypox virus (MPXV), has posed a significant threat to public health globally. On 14 August 2024, WHO has declared monkeypox a health emergency after the cases reported in Africa. While initially identified in the Democratic Republic of Congo in 1970 (Marennikova et al., 1972), the virus has demonstrated its potential for geographical expansion. In recent years, monkeypox outbreaks have occurred in various regions, including Africa, North America, and Asia (Petersen et al., 2019). The May 2022 witnessed an outbreak of Mpox that rapidly propagated to 116 countries with more than 97,745 reported cases and 203 deaths (Organization, 2022). WHO reported that recent surge of monkeypox cases in Africa exceeds the number of all the cases reported during 2023. Pakistan, specifically, has experienced sporadic cases of monkeypox, leading to substantial health risks and economic burdens. The case of MPXV is unusual as it propagated to many countries with sustained chains of transmission where it wasn’t endemic earlier with no apparent epidemiological link and without any documented Mpox transmission.
The recent outbreak of Mpox is notable as the genomic sequences reported from it have formed distinct lineage (IIb) within clade II (Gigante et al., 2022). There has been potential evidence of intra host genomic variation in lieu of de novo mutations in Mpox sequences (Taouk et al., 2023). Previously, gene rearrangements, loss or duplication have been reported in Orthopoxviruses which on seeing in continuity, suggest possible viral adaptive evolution to new host for human-human transmission (Brinkmann et al., 2023; Elde et al., 2012; Senkevich et al., 2021). There are potential knowledge gaps in understanding the articulation of humoral and innate immune responses while fighting with MPVX. There are no vaccines or treatment available for MPVX infection till date (Lozano and Muller, 2023). In this context, many countries have been using small pox vaccines and drugs to counter MPVX infection, however majority of such usage is not sufficiently documented (Kmiec and Kirchhoff, 2022). Currently, the JYNNEOS vaccine get emergency use authorization license on August, 2022 from FDA and administered to the adults above 18 years of age. It is live virus vaccine that contains Modified Vaccinia Ankara-Bavarian Nordic (MVA-BN), a weakened, non-replicating orthopoxvirus. The other vaccine approved for monkey pox is ACAM2000 which is live vaccinia virus vaccine. This vaccine is also used for small pox1. Both of these two vaccines based on the live attenuated approach. The advent of heterologous gene insertion into pox virus genomes enhanced its vaccinable potential not only for its own genera but for its usage in other heterologous diseases such as Rabies, Influenza and Hepatitis B (Esposito et al., 1988; Panicali et al., 1983; Smith et al., 1983a; Smith et al., 1983b).
Traditional pathway of vaccine development can take between 5 and 10 years to get a viable candidate, however the case of COVID-19 pandemic has provided a potential vaccine candidate in the stage of interim analysis of efficacy testing in just under 300 days from the day of availability of first viral SARS CoV2 sequence (Ball, 2021). The emergence of computational methods and bioinformatics in drugs and vaccine designing has unprecedently augmented the discovery pipeline, which have been well utilized in COVID-19 pandemic (Napolitano et al., 2022). Given the constant threat of emerging infectious diseases, this approach holds promise in providing responses to outbreaks and in designing targeted vaccines.
This manuscript aims to present an in silico vaccine design strategy targeting the recently reported genomic sequence of monkeypox virus strain in Pakistan alongwith recently reported sequences from 2022 MPXV epidemic across the globe. By leveraging genetic sequence data of the circulating MPXV strain, we seek to identify potential epitopes and assess their immunogenicity. Furthermore, we will employ computational tools and algorithms to evaluate the binding affinities of these epitopes to major histocompatibility complex (MHC) molecules, enabling T-cell receptor recognition and subsequent immune response activation.
Material and methods
Sequence retrieval
The sequence of monkeypox from Pakistan (GISAID ID: EPI_ISL_17536780) was retrieved from GISAID. The nucleotide sequence is around 200 kb in size and encode 190 proteins. The antigenic potential of all the proteins were determined through VaxiJen web server (Doytchinova and Flower, 2007). The antigenic proteins were further subjected to determine the subcellular localization of proteins using Cell-Ploc-2 web server (Chou and Shen, 2008). The proteins that expressed on the cell membrane and in the extracellular region were further subjected for the identification of signal peptide and their secretory nature using Signal-BLAST web server (Frank and Sippl, 2008).
The shortlisted proteins were subjected for toxicity analysis through Toxinpred web server (Gupta et al., 2015). These proteins were also screened for the allergenicity profile through AllerTop web server (Dimitrov et al., 2013). The proteins were also subjected to BLASTp program for identification of human homologues with percent identity ≤35. The final proteins included in the analysis were antigenic, secretory in nature, non-toxic, non-allergic and non-homologues to humans. The shortlisted proteins were then used for the identification of B- and T-cell epitopes.
B-cell epitope prediction
For identification of B-cell epitopes, Immune Epitope Database (IEDB) and ABCPred were used. The B-cell prediction tool at IEDB predicts the epitopes on the basis of protein flexibility, antigenicity, and surface accessibility (Fleri et al., 2017). The default threshold of 1.00 was used for the epitope prediction. The ABCPred predict the B-cell epitopes on the basis of recurrent neural network (RNN) (Saha and Raghava, 2006). The 16–18 amino acids long peptides with threshold of 1.0 were selected for further analysis. The epitopes found promising in the two platforms were consider for further investigation.
T-cell epitope prediction
T cell (cytotoxic and helper T-cell) epitopes play an important role in eliciting immune response. The T-cell epitopes are recognized by histocompatibility complex (MHC) molecules as MHC-I and MHC-II.
The cytotoxic T-cell epitopes recognized by MHC-I were predicted through NetMHCpan4.0. NetMHCI 4.0 predicts the binding affinity through artificial neural network by schooling 81 distinct HLA-A, -B, -C and -E human MHC alleles (Reynisson et al., 2020). The recommended peptides that elicit MHC-I response were of nine residues long hence the 9-mer peptides with binding affinity ≤100 nM were selected. The T-helper cell epitopes were predicted through NetMHCIIpan4.0. It is based on artificial neural network and predict the binding affinity based on three human MHC class II isotypes HLA-DR, HLA-DQ, HLA-DP (Reynisson et al., 2020). The peptide length for binding of epitopes were of 13–17 amino acid in length hence the 15 amino acid long peptides with binding affinity ≤100 nM were selected.
The selected MHC-II peptides were further scrutinized for their potential to elicit IFN-γ response using IFN epitope server2. The server predicts IFN-γ response on the basis of support vector machine (Dhanda et al., 2013). The peptides with the positive values were considered to be capable of inducing IFN-γ production.
Epitope selection
All the shortlisted B- and T-cell epitopes were subjected for further evaluation for their potential antigenicity, toxicity, and allergenicity potential. The peptides that were antigenic (predicted through VaxiJen) were further evaluated for toxicity through Toxinpred and the non-toxic peptides were further evaluated for allergic potential using AllerTop (Dimitrov et al., 2014). The BLAST-p program was used to identify the human homologues. The non-human homologues with identity <30% were selected for final vaccine construction.
Population coverage
In order to determine which population would get benefits from vaccine epitopes, it is important to predict the population coverage of selected epitopes. To predict the population coverage, we used the Population Coverage3 tool available at IEDB online server was used (Bui et al., 2006). Final selected MHC-I and MHC-II data were used to identify the population coverage across all the regions of the World.
Vaccine construction
The immunogenicity of peptide vaccines can be low, therefore, a multi-epitope peptide vaccine can overcome this problem. Hence, a multiepitope vaccine is created by fusing the overlapping B- and T-cells epitopes with the adjuvant and linkers. Linkers render vaccine constructs stable and permit them to behave independently after being injected into the host while adjuvant augments the immunogenic response (Ismail et al., 2022). Three different vaccine constructs were designed using 50S ribosomal protein L7/L12 adjuvants, TLR4, and β-defensin. The sequence of adjuvants were downloaded from Uniprot. The EAAK linker was used to join the adjuvant at the N-terminus of vaccine construct. The B-cell epiotpes were linked through KK linker while T-cell epitopes were joined through GPGPG linker. The physicochemical properties of the vaccine constructs like molecular weight, GRAVY, PI, stability index and half life were evaluated through ProtParam tool available on ExPASy server (Wilkins et al., 1999). The solubility of vaccine constructs was evaluated through SOLpro web server (Magnan et al., 2009). All the four vaccine constructs were analyzed for their antigenicity, toxicity, allergenicity, and autoimmunity using VacxiJen, Toxinpred, AllerTop and BLAStp Programs.
Secondary and tertiary structure prediction
The secondary structure of the final vaccine construct was predicted via PSIPRED (Jones, 1999). The tertiary structure was predicted through trRosetta and I-TASSER (Du et al., 2021). I-TASEER is an iterative threading program that builds 3D structure by using the hierarchical method. trRosetta is de novo structure prediction tool based on deep neural network. Each method generate five models of each vaccine construct. The models were evaluated using Ramachandran plot, and ERRAT plot (Colovos and Yeates, 1993; Lovell et al., 2003). The protein model with the highest quality factor and accurate geometry according to Ramachandran plot was further undergo refinement using DeepRefiner server (Shuvo et al., 2021).
Molecular dynamics (MD) simulation
Molecular dynamics simulations were conducted for all three vaccine constructs using the GROMACS 2024.3 package, with each construct subjected to a 100 ns simulation. The structures were initially minimized using the Amber force field. Energy minimization was performed for 1,000 steps using the Steepest Descent Method, achieving convergence with a maximum force <1,000 (KJ mol⁻1 nm⁻1) to eliminate steric clashes. The system underwent equilibration under NVT and NPT ensembles for 100 ps (50,000 steps) and 1,000 ps (1,000,000 steps), respectively, with time steps of 0.2 and 0.1 fs at 300 K, ensuring the systems were fully equilibrated for the production run. The 100 ns production run was carried out at a constant temperature of 300 K and pressure of 1 atm (NPT) using weak coupling velocity-rescaling (modified Berendsen thermostat) and Parrinello-Rahman algorithms. The simulation results were analyzed based on root mean square deviation (RMSD), root mean square fluctuation (RMSF), and radius of gyration (RoG).
Molecular docking
In order to generate immune response, the vaccine construct has to interact with the immune cell receptor. Hence, the docking of vaccine construct was studied with the Toll-like receptor 4 (TLR4), Toll-like receptor 3 (TLR3) and Toll-like receptor 7 (TLR7)to evaluate vaccine-immune receptor interactions. Structure of TLR4 (PDB ID: 4G8A), TLR3 (PDB ID: 1ZIW), and TLR7 (PDB ID: 5GMF) were downloaded from protein data bank. All the receptor structures were prepared for docking using Molecular Operating Environment (MOE) software. Briefly, the heteroatoms and co-crystallize ligands were removed followed by energy minimization using Amber12 force field. Molecular docking of all three multi epitope vaccines with TLR4 was performed using ClusPro server (Kozakov et al., 2017). The server performs docking of two interacting proteins. The server uses the rigid body docking approach by exploring billions of initial conformations. After that 1,000 lowest energy structures are shortlisted on the basis of RMSD value in order to find the best cluster that represent the most likely complex. Finally, the refinement of selected complex was performed through energy minimization (Kozakov et al., 2017). The binding flexibility and stability of vaccine-TLR4 complex was predicted through iMODS server. The server utilizes an elastic network model (ENM) to perform Normal Mode Analysis (NMA) in internal (dihedral) coordinates (Lopez-Blanco et al., 2014). For each complex the server provide deformability plots and B-factor, mode variance plot, eigenvalues, elastic network, and covariance map.
Immune simulation
To evaluate the immune profile of vaccine construct, computational immune simulation was performed using online C-ImmSim server. This server uses a position-specific scoring matrix (PSSM) and machine learning techniques for the prediction of the immune response (Rapin et al., 2011). The simulation parameters were set at default for a period of 1, 84, and 168 h with default host HLA MHCI and MHCII alleles. The simulation was run for 1,000 steps.
Codon optimization and in silico cloning
Codon optimization is an important step in reverse vaccinology in-order to avoid the codon bias which in turn enhance the expression of recombinant protein. The Java codon adaptation index (JCAT)4 was used to reverse translate amino acid sequence of vaccine into cDNA for effective expression of vaccine construct in E.coli vector. It provides the Computed Codon Adaptation Index (CAI) values and GC content of the vaccine construct (Grote et al., 2005). These two parameters are important to evaluate the expression of vaccine. The optimal transcriptional and translational efficiency of vaccine achieved with CAI >0.8 and GC content between 30%–70%. The adapted codon sequence was cloned into pET-28a (+) vector using SnapGene5. This vector enables enhanced protein recovery and purification due to its N-terminally 6 × His-tagged proteins.
Results
A total of 190 proteins were coded by the Monkeypox strain reported from Pakistan. The proteins were shortlisted based on antigenicity. Based on the Vaxijen results, 117 proteins were found to be antigenic while 73 proteins were non-antigenic with threshold of <0.4. These 117 proteins were further subjected to predict the subcellular localization using Cell-Ploc-2 server. The proteins with cell membrane location and secretory nature were subjected for identification of signal peptide or the secretory nature. A total of 10 proteins were found to be of secretory nature. All of these proteins are non-allergic and non-toxic. The final list was subjected to BLAST analysis and the results revealed that all the proteins were non-human homologues. The final shortlisted 10 proteins were subjected to epitope prediction (Table 1).
B-cell epitope prediction
On the basis of B-cell epitope prediction server, 16- and 18-mer epitopes were predicted for all the 10 proteins. The epitopes were shortlisted on the basis of their antigenicity and allergenicity. No epitope was predicted for EGF-like domain protein. The epitopes predicted for Envelope protein A28 homolog and Crm-B secreted TNF-alpha-receptor-like protein were non-antigenic and allergen. Hence, not included in the study. 12 epitopes were prioritized from seven proteins based on their high antigenicity, low toxicity, and low allergenic reactions (Table 2).
T-cell epitope prediction
A total of 122 CTL epitopes were predicted with binding affinity ≤100 nM with MHC-I alleles. Among these epitopes, 33 epitopes were predicted to be antigenic, non-toxic and non-allergen (Table 3). A total of 44 helper T-cell (HTL) epitopes were identified with binding affinity ≤100 nM with MHC-II alleles. Out of 44 HTL epitopes, 12 epitopes satisfied the antigenicity, toxicity, and allergenicity tests (Table 4).
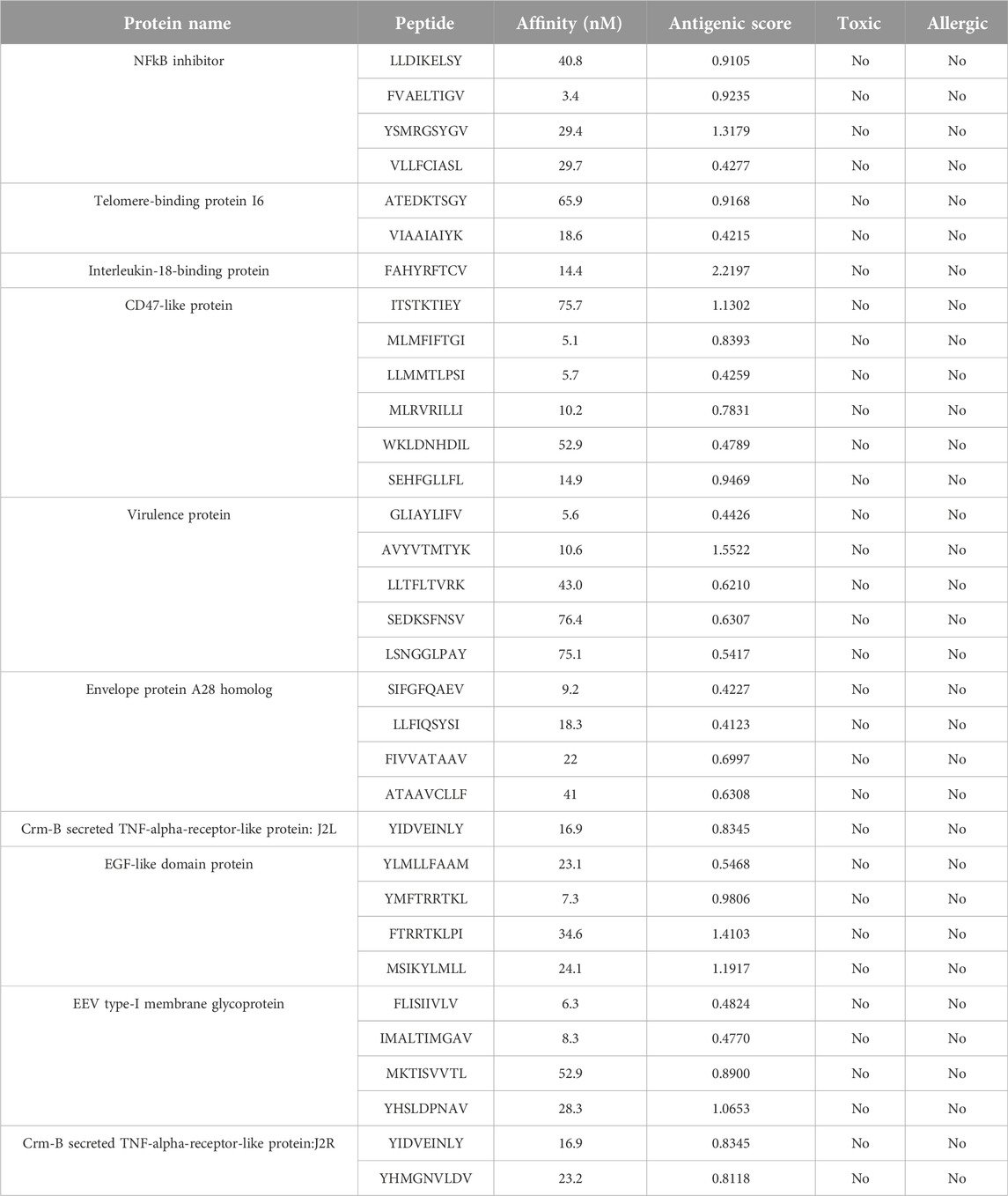
Table 3. Properties of predicted cytotoxic T-cell epitopes having binding affinity with MHC-I alleles.
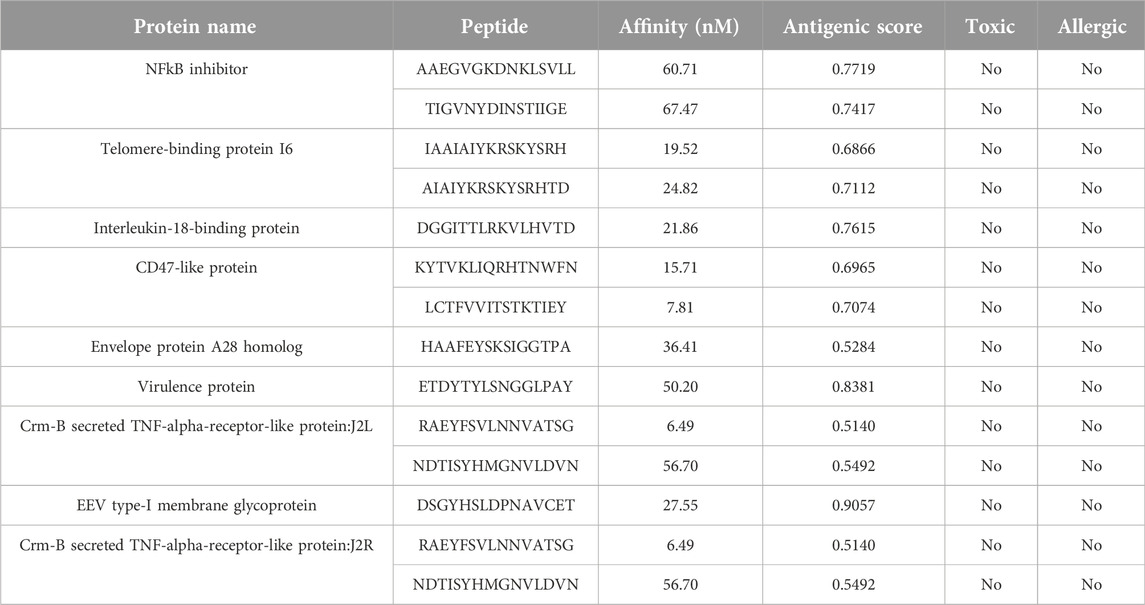
Table 4. Properties of predicted helper T-cell epitopes having binding affinity with MHC-II alleles.
The shortlisted, 12 B-cell, 33 CTL and 12 HTL epitopes were further shortlisted on the basis of overlapping features. 6 B-cell, 8 CTL and 6 HTL overlapping epitopes were used to design vaccine construct (Table 5). The eventual target has been to determine lead epitopes with the potential to prompt humoral and cell mediated immunogenic responses. The selected T-cell epitopes were further subjected to identify the population coverage. The IEDB results revealed that the selected epitopes showed 97.5% worldwide coverage of both the MHC I and MHC II alleles. Population coverage in different regions of world is shown in Figure 1.
Vaccine construct
To design vaccine construct 6 B-cell, 8 CTL and 6 HTL epitopes were used using EAAK, KK and GPGPG linker. Three different vaccine constructs were designed based on three different adjuvants. To enhance protein purification, a 6xHis tag was added to the C terminal. The adjuvants were added to N-terminal to construct the vaccine. On the basis of adjuvant, three vaccine constructs were generated as V1 (adjuvant: beta defensin), V2 (adjuvant: 50S ribosomal protein L7/L12) V3 (adjuvant: TLR4 agonist).
Immunological profile of all the three vaccine constructs showed that these are antigenic, non-toxic and non-allergic. The antigenic score of all the three-vaccine construct >0.8 indicating the antigenic nature of vaccine constructs. The SOLpred score of all the three vaccine constructs >0.6 indicating the solubility upon overexpression (Table 6). The physiochemical properties of all the three vaccine constructs is shown in Table 7. As all the vaccine constructs have similar protein sequence the only difference is the presence of adjuvant hence the physicochemical properties differ slightly among these three constructs. The molecular weight ranges from ∼20 kDa to ∼25 kDa. Theoretical pI values range from 8.8 to 9.3. The stability index lies in the range of 16–29, that indicate the stability of vaccine constructs. GRAVY scores from −0.425 to −0.583 indicate the hydrophilic nature of the vaccine constructs. Aliphatic index (72.2–86.8) describes the thermostability of vaccine constructs. These results indicate that all the three vaccine constructs may be able to initiate immunological response into the host cell.
Secondary and tertiary structure prediction
In order to understand the interactions of vaccine with the host immune cells, stable three dimensional structure is required. Rosetta was used to generate the structure of all the three vaccine constructs and the resulting models were evaluated for structure stability. Five models were generated for each vaccine construct. Top scoring models were further subjected to structural refinement. The results of structural validation showed that ERRAT score >80. The ramachandran plot showed that 78.1% residues of V1, 85.2% residues of V2, and 85.7% residues of V3 fall in favored region of plot. Hence, these results indicate that the predicted 3D structure of vaccine constructs are of good quality and used for further investigation (Figure 2).
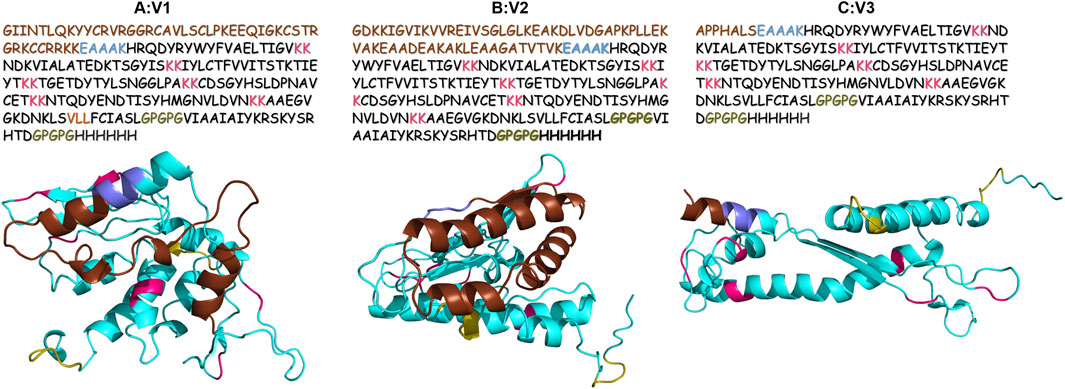
Figure 2. Three dimensional structure of (A) V1; (B) V2; (C) V3 vaccine constructs along with primary sequence. Adjuvant is highlighted in brown color. While linkers are highlighted in blue, pink and olive green color.
Molecular dynamics simulation
The RMSD, RMSF, and radius of gyration analyses for all three vaccine constructs indicate that V1 is the most stable (Figure 3). The RMSD of V1 increases during the first 50 ns but stabilizes thereafter. In contrast, the V2 construct remains unstable throughout the 100 ns simulation, with its RMSD continuing to rise. For V3, the RMSD stabilizes after 70 ns, but it remains higher than that of V1.
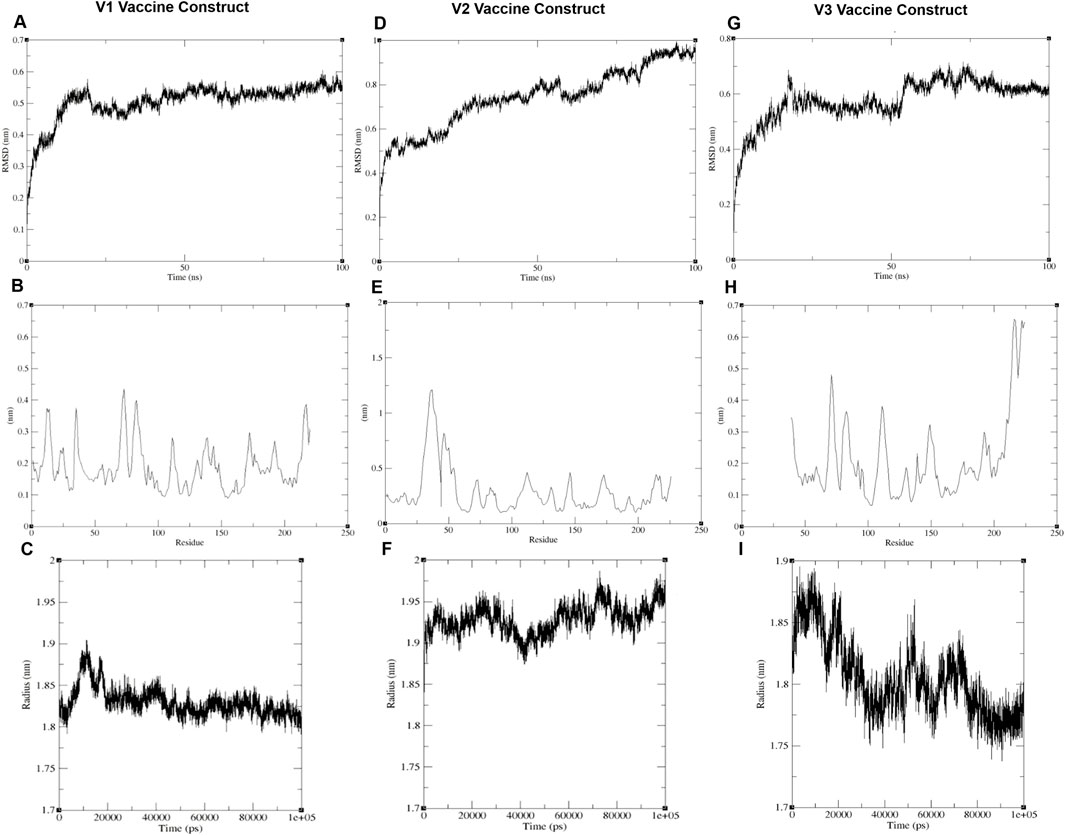
Figure 3. The compactness of vaccine constructs indicated by (A, D, G) Root mean square deviation, (B, E, H) Root mean square fluctuation, and (C, F, I) radii of gyration.
Regarding the radius of gyration, V1 maintains a value of around 1.8 nm, indicating compactness. V2 has a higher radius of gyration, approximately 1.95 nm, suggesting a more expanded structure. V3 fluctuates between 1.75 and 1.8 nm, showing moderate compactness, but still less stable than V1.
Molecular docking
The binding of all the three vaccine constructs with TLR4 was evaluated using ClusPro. For each vaccine complex, Cluspro generated 120 structures. Top ten complexes of all the three vaccine constructs were subjected to protein binding energy prediction using PRODIGY. The server predicts the binding affinity in the complexes. On the basis of binding energy, the V1 vaccine construct showed the lowest binding energy among all the three complexes (Table 8). The interaction analysis revealed that 11 residues of V1 are directly involved in interactions with TLR4 (Figure 4).
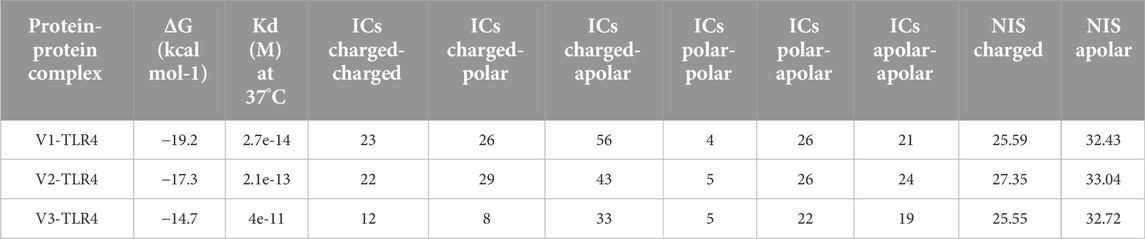
Table 8. Binding energy and number of interface residues of docked complexes (vaccine constructs with TLR4).
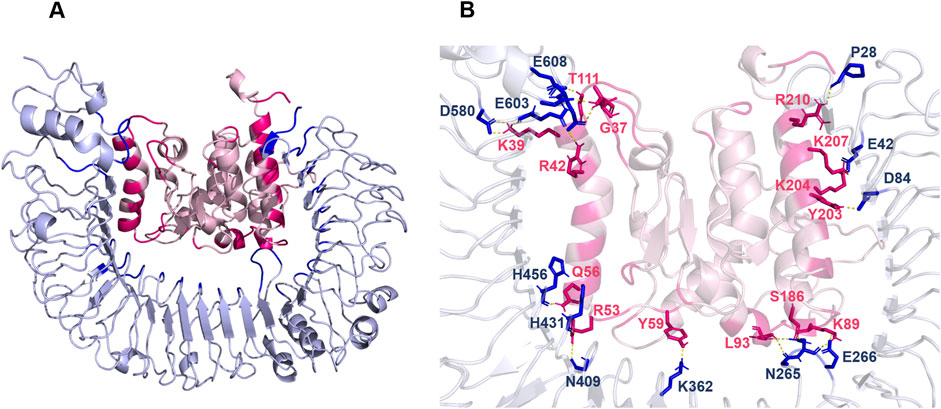
Figure 4. (A, B) Docking of V1 with TLR4. TLR4 is shown in silver color while vaccine construct is represented with pink color.
All the three complexes were further subjected for deformability analysis. All the three complexes have relatively similar deformability in the residues (Figures 5A, E, I). Furthermore, the force required to deform the complex is measured through Eigenvalue. Lower the Eigenvalue, easier the structure will deform. According to graphs reported in Figures 5B, F, J, V1 complex have high eigenvalue compared to V2 and V3. Hence, the complexes of V2 and V3 with TLR4 are easier to deform. The covariance matrix also showed the correlated movements of atoms in case of V1 compared to V2 and V3 complexes as there is relatively more red region in V1 compared to V2 and V3 (Figures 5C, G, K). The elasticity of vaccine constructs is represented in Figures 5D, H, and L.
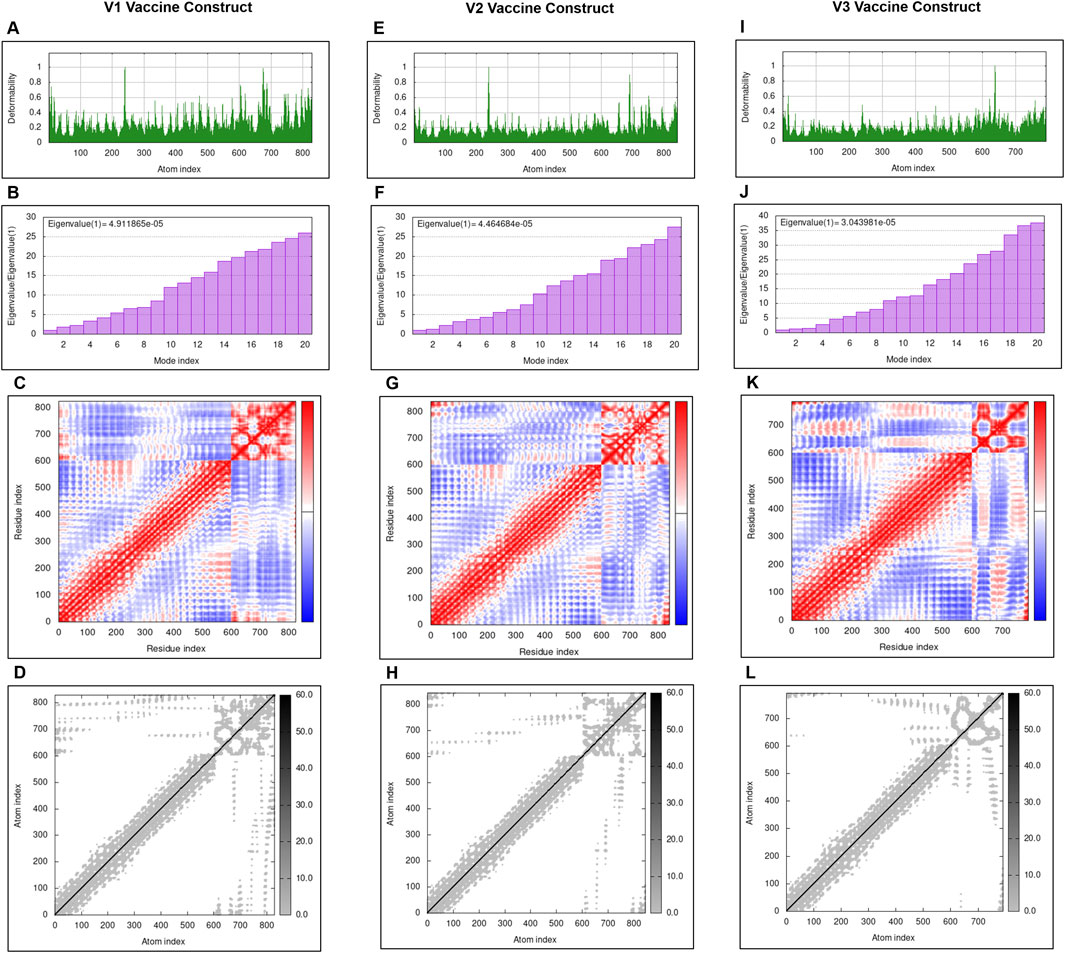
Figure 5. The results of molecular dynamics simulation of all the three vaccine constructs and TLR4 complex achieved by iMODS server. deformability of V1, V2, and V3 is represented in (A, E, I); eigenvalues (E) of V1, V2, and V3 are represented in (B, F, J); Covariance matrix of V1, V2, and V3 are represented in (C, G, K). Coviarnace matrix indicates correlated (red), uncorrelated (white), and anti-correlated (blue) motions of paired residues; elastic network model of V1, V2, and V3 are represented in (D, H, L). Grey regions indicates stiffer regions.
The interactions of V1 vaccine construct were further studied with TLR3 and TLR7 (Figure 6). The binding affinity of V1 with TLR3 is lower than with the TLR7 (Table 9). The interaction analysis revealed that there relatively strong interactions of V1 with TLR3 as compared to TLR7. The immune simulation results of V1 with TLR3 and TLR7 is also show a string immune response (Figure 7).
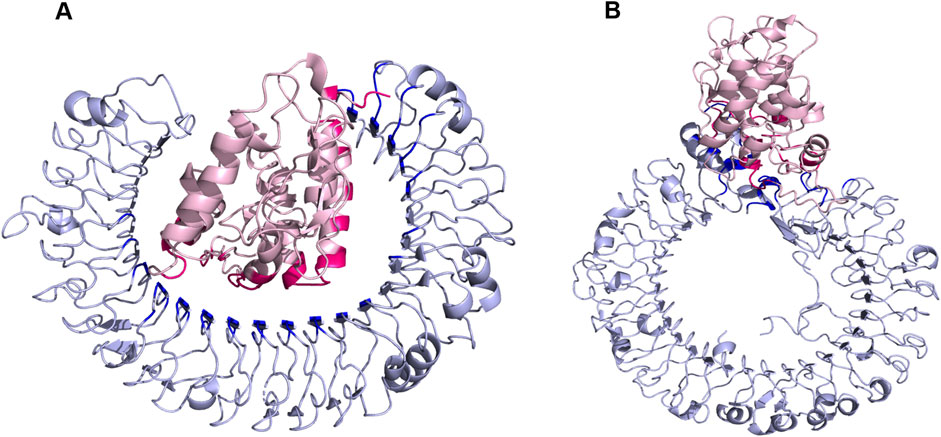
Figure 6. Docking of V1 with (A) TLR3 and (B) TLR7. TLR3 and TLR7 is shown in silver color while vaccine construct is represented with pink color.

Table 9. Binding energy and number of interface residues of docked complexes (V1 vaccine construct with TLR3 and TLR7).
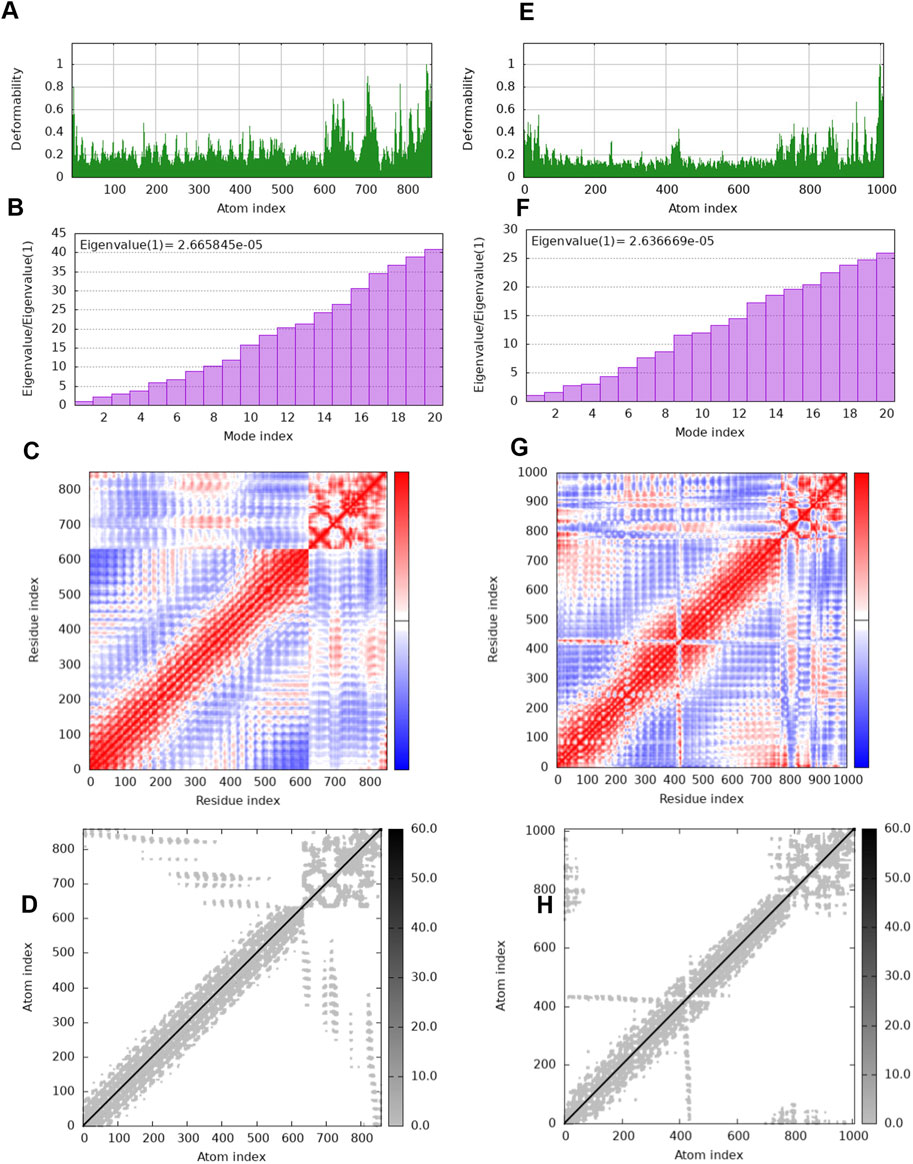
Figure 7. The results of molecular dynamics simulation of V1 vaccine constructs and TLR3 and TLR7 complex achieved by iMODS server. deformability of V1-TLR3 and V1-TLR7 is represented in (A, E); eigenvalues (E) of V1-TLR3 and V1-TLR7 are represented in (B, F); Covariance matrix of V1-TLR3 and V1-TLR7 are represented in (C, G). Coviarnace matrix indicates correlated (red), uncorrelated (white), and anti-correlated (blue) motions of paired residues; elastic network model of V1-TLR3 and V1-TLR7 are represented in (D, H). Grey regions indicates stiffer regions.
Codon optimization and in silico cloning
Final vaccine construct (V1) was subjected to codon optimization using JCat web server. To obtain high expression in the expression system, the peptide sequence of V1 was reverse translated into DNA. The GC content and predicted CAI value of vaccine construct was 48.4% and 1.0, respectively. Snapgene was used to perform the in silico cloning. The multiepitope vaccine construct exhibits PstI and RsrII restriction sites at the start and end, respectively. The pBAD-DEST49 vector was used for cloning as it exhibits both the PstI and RsrII sites. The multiepiotpe vaccine construct (V1) was cloned into pBAD-DEST49 vector in-order to ensure the expression in the E.coli expression system (Figure 8A). In order to check the insertion of peptide into vector, the vector was digested with PstI and RsrII enzymes. The virtual agarose gel simulation showed the presence of insert along with vector (Figure 8B).
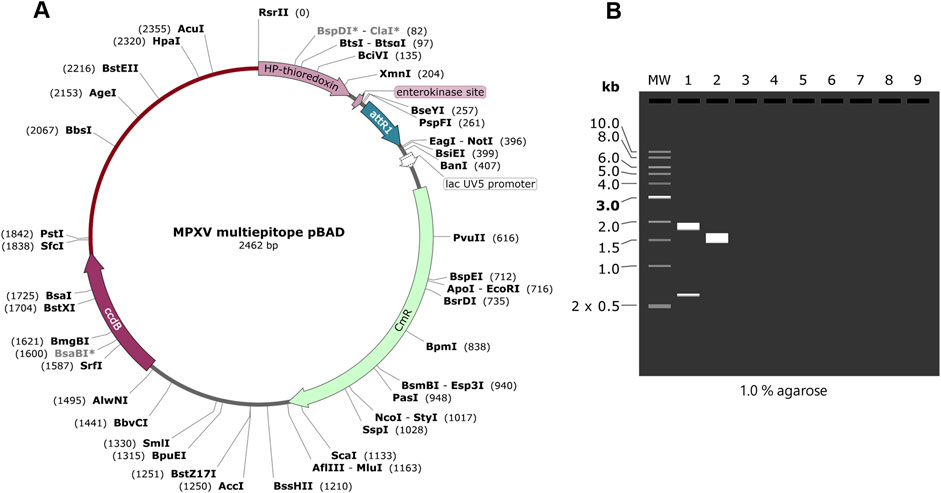
Figure 8. (A) In silico cloning of V1 vaccine into pBAD-DEST49 vector. The vaccine construct cloned into restriction sites of PstI and RsrII enzymes. (B) Virtual agarose gel of vaccine construct.
Immune simulation
To understand the immune response of vaccine construct, immune simulation was performed. The results showed that there was high level of IgM in the initial phase of vaccine administration followed by IgG1 and IgG2 (Figure 9A). The same trend observed in human body immune responses where the primary immune response is generated by IgM and secondary and tertiary immune response is generated by B-cell, IgG, and IgM. As the antibody response is generated there is decrease in the antigen level as well. The exposure of antigen in the vaccine construct also led to rapid increase in population of memory B-cells (Figure 9B). Along with the memory B-cell population there was an increase in cytotoxic and helper T-cell population (Figures 9C, D). Like real-time immunization, there was constant elevated levels of natural killer cells, dendritic cells, and macrophages (Figures 9E–G). Increased level of cytokines and interleukins were also observed in response to vaccine administration (Figure 9H). These results suggest that the vaccine construct (V1) can generate immune response inside the human host.
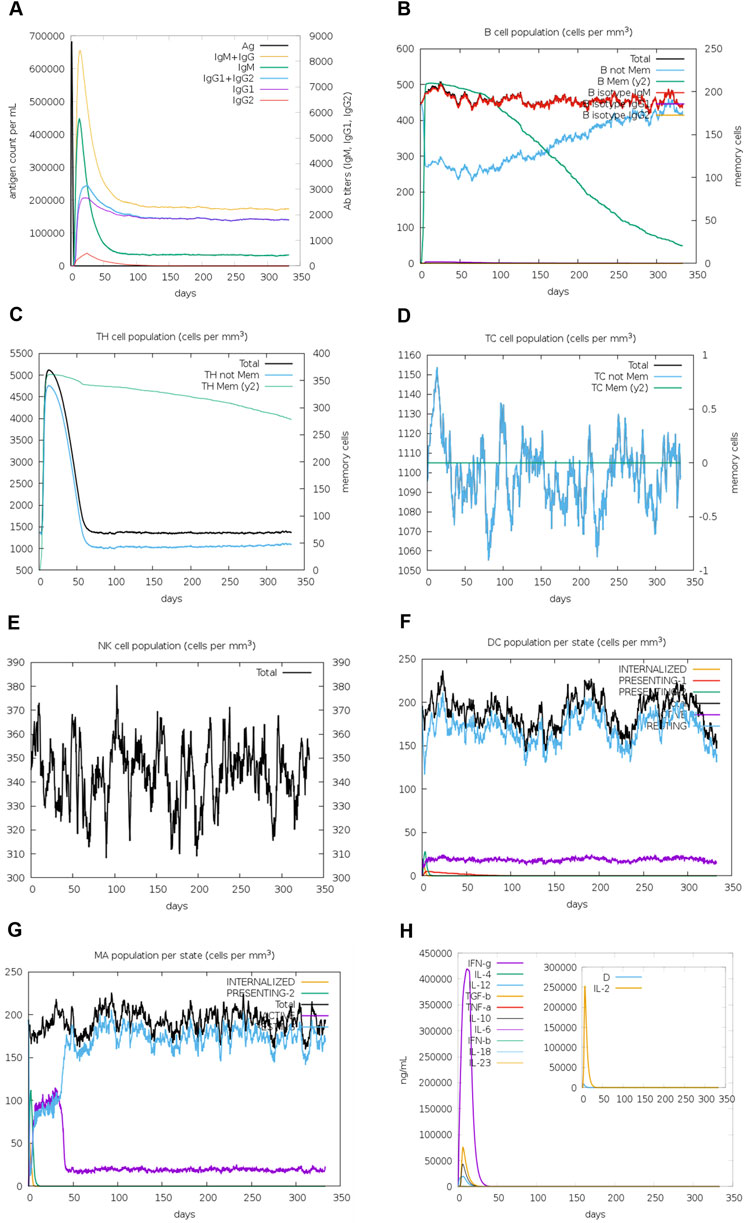
Figure 9. In silico immune simulation to predict the immunological potential of V1. (A) Increased level of immunoglobin antibodies with a decrease in antigen levels upon vaccine injections. (B) Rising B-cell populations after repeated exposure to antigen. (C, D) The increase in the population of T-cytotoxic and T-helper cells upon repeated antigen exposure. (E–G) The population increase of dendritic cells, macrophages, and natural killer cells during the immunization period. (H) Increased concentrations of cytokine and interleukin levels after the repeated antigen exposure. The inset plot shows the danger signal together with leukocyte growth factor IL-2.
Discussion
Monkeypox has emerged as global concern after its detection in the non-endemic countries in 2022. Due to similarities between monkeypox and smallpox, the smallpox vaccine is approved by the Food and Drug Administration (FDA) in September 2022 for prevention of smallpox and monkeypox disease in adults 18 years or older at high risk for smallpox or monkeypox infection. The vaccine has limited efficacy in children and people with underlying conditions (immune-compromised individuals). Hence, there is a need to develop vaccine that is specific to monkeypox and can be administered to all the individuals.
Recent advancement in the reverse vaccinology approaches and availability of genomic and proteomic data make it possible to design multi-epitope vaccines efficiently and timely manner. Vaccine constructs with the several epitope regions might improve both the antigenicity and immunogenicity of the vaccines (Parvizpour et al., 2020). As the multiepitope vaccines stimulate immune response by targeting conserved epitopes in the whole antigenic sequences so this avoids responses against unfavorable epitopes, which could potentially induce immunopathogenic or immune-modulating responses against the host (Vartak and Sucheck, 2016; Zhou et al., 2009). Currently, there is no specific treatment of monkeypox hence there is a need to design specific multi epitope vaccine against monkeypox to eradicate the infection. In the current study, immuneinformatic approaches were exploited to design multi epitope vaccine against monkeypox using different antigenic proteins. The complete genome of monkeypox sequenced from Pakistan encode 190 different proteins. All these proteins are not exposed and expressed in extracellular environment. The properties of a protein to be a good candidate of vaccine is to be secretory in nature, extracellular expression, antigenic, anti-allergic, and non-toxic. In the study the proteins are first shortlisted based on having signal peptide and expression on cell surface. The proteins with the signal peptide can be targeted to the secretory pathway (Hegde and Bernstein, 2006; Nakai, 2000). Additionally, signal peptide enhance protein secretion therefore can be employed in recombinant techniques to enhance protein secretion (Fonseca et al., 2018). A study conducted by Lee et al., 2019 showed that vaccination of mouse with secreted viral nonstructural protein (NS1) protect the mouse from murine norovirus (Lee et al., 2019). It has been reported that proteins with signal peptide as vaccine candidate provide high specificity and antigenicity (Kovjazin and Carmon, 2014). The proteins selected for analysis are antigenic with antigenic score of >0.4. It has been suggested that higher antigenicity value is associated with a stronger immune response in the host (Can et al., 2020). These antigenic proteins were subjected to B- and T-cell epitope identification. The six proteins were finalized that harbor the overlapping B-and T-cell epitopes to generate both the humoral and cell mediated immune response. B-cell epitopes have important role in antibody production (Cooper and Nemerow, 1984). Antigens have the capability to abate the humoral immune response by B cell alone, coupling with the T cell mediated immunity can change the response and can result in extended protection. (Bacchetta et al., 2005). Cytotoxic T lymphocytes restricts pathogens advance with specific the identification of virus and targeting them with specialized cytokines rendering it to their full elimination of infected cells (Garcia et al., 1999). The final vaccine construct composed of overlapping B- and T-cell epitopes from all six antigenic proteins of monkeypox along with linkers and adjuvant for long term immunity. The vaccines with all these properties perform well in vivo. Using the same approach there are vaccines that are in clinical trials (He et al., 2018; Jiang et al., 2017; Lu et al., 2017).
The epitopes are joined through EAAAK, KK, and GPGPG linkers. The KK and GPGPG linker known to produce junctional immunogenicity in vaccine construct while EAAAK linker contribute towards the enhanced bioactivity and stability of vaccine constructs (Bazhan et al., 2019). On the basis of different adjuvants, three vaccine constructs were designed and all of them found to be highly antigenic and non-allergic. The physicochemical properties also support that the designed vaccine constructs are stable and able to interact with water as suggested by GRAVY value. The high aliphatic index also suggestive of thermostability of vaccine construct.
In order to understand the interactions of vaccine construct with the immune molecules to elicit immune reaction, molecular docking of vaccine construct with the toll like receptor was performed. Different TLRs are expressed in macrophage cells, granulocytes, and monocytes and are able to generate adaptive immune responses (Vaure and Liu, 2014). Previously, the TLR4 has been shown to be involved in viral peptide recognition and subsequent production of inflammatory cytokines (Vaure and Liu, 2014; Compton et al., 2003).
The interactions of all the three vaccine constructs were studied with the TLR4. All the vaccine constructs bind to the active site of TLR4. On the basis of binding energy and interacting residues the best vaccine candidate was selected and V1 was found to be the best vaccine candidate on the basis of lowest binding energy (−19.2 kcal/mol) and large number of H-bonding and hydrophobic interactions with the TLR4. The interactions of V1 were also studied with TLR3 and TLR7 and results indicate that V1 have the ability to bind with the TLR3 and TLR7. Molecular dynamic simulations also support that the V1 is the stable vaccine construct.
The first step in validation of proposed vaccine is to test for immunoreactivity utilizing serological assessment (Gori et al., 2013). Hence, it is important to use suitable expression system to express the vaccine peptide into host. E.coli expression system was used to express the peptide. The expression systems based on E. coli has been rendered suitable to produce recombinant proteins (Chen, 2012; Rosano and Ceccarelli, 2014). There is high level of expression of vaccine peptide in E.coli on the basis of codon adaptability index of 1 and GC content of 48.4%. The immune simulation also confirms the similar responses as observed in the human body after administration of vaccine. There is production of memory B- and T-cells after vaccine administration. There is also an increase in the expression levels of cytokines, IFN-γ, and interleukins. The current study provides a multiepitope vaccine construct against monkeypox virus using immune informatics approaches. Although the in-silico approaches save time to design new vaccine, but it has one major limitation that all the results are based on prediction and it is important to validate these results in vitro to determine the stability, safety and immunological profile of designed vaccine. As the epitopes are predicted through in-silico approaches there may be chance that not all the epitopes elicit the desired immune response when validated in vitro. This should further be considered with context of diversifying host pathogen interaction complexity. Another important factor to consider is the population coverage although the population coverage predicted through in silico methods but there may be populations where vaccines may provide differing outcome. Moreover, there is also a challenge of large-scale production of vaccine that demands cost, technical expertise, and quality controls. The scale up process in vaccine development from transitioning thru in silico design development to laboratory testing and further to optimal commercial manufacturing is both time and resource intensive. After production another challenge is to maintain the cold chain for vaccine most of the vaccine are stable between 2°C and 8°C but it is difficult to maintain that temperature in countries with long hot and humid summers. The delivery of vaccine also faces legal and ethical challenges there are some people who did not inject vaccines.
The current study designs a multiepitope vaccine construct considering the monkeypox sequence from Pakistan. The selected proteins and the epitopes for vaccine construction are conserved among different monkeypox sequences reported around the globe and it has 99.8% population coverage worldwide that suggest the vaccine can generate immune responses in wide range of population. The V1 vaccine construct is highly antigenic, non-allergic, and able to generate immune responses within body. Moreover, the vaccine construct has highest binding affinity with TLR4 receptor that showed that it can generate adaptive immune response within body. However, further in-vitro and in-vivo studies will be required to study the safety and efficacy of vaccine.
Data availability statement
The original contributions presented in the study are included in the article/supplementary material, further inquiries can be directed to the corresponding author.
Author contributions
Conceptualization, ZR and AF; methodology, ZR, MI, and AF; formal analysis, ZR, AF, and MI; resources, AF and ZR; writing–original draft preparation, ZR and MI; writing–review and editing, ZR, AF, and MI. All authors contributed to the article and approved the submitted version.
Funding
The author(s) declare that no financial support was received for the research, authorship, and/or publication of this article.
Conflict of interest
The authors declare that the research was conducted in the absence of any commercial or financial relationships that could be construed as a potential conflict of interest.
Footnotes
1https://www.fda.gov/vaccines-blood-biologics/vaccines/key-facts-about-vaccines-prevent-mpox-disease
2http://crdd.osdd.net/raghava/ifnepitope/
3http://tools.iedb.org/population/
References
Bacchetta, R., Gregori, S., and Roncarolo, M. G. (2005). CD4+ regulatory T cells: Mechanisms of induction and effector function. Autoimmun. Rev. 4 (8), 491–496. doi:10.1016/j.autrev.2005.04.005
Ball, P. (2021). The lightning-fast quest for COVID vaccines - and what it means for other diseases. Nature 589 (7840), 16–18. doi:10.1038/d41586-020-03626-1
Bazhan, S. I., Antonets, D. V., Karpenko, L. I., Oreshkova, S. F., Kaplina, O. N., Starostina, E. V., et al. (2019). In silico designed ebola virus T-cell multi-epitope DNA vaccine constructions are immunogenic in mice. Vaccines (Basel) 7 (2), 34. doi:10.3390/vaccines7020034
Brinkmann, A., Kohl, C., Pape, K., Bourquain, D., Thürmer, A., Michel, J., et al. (2023). Extensive ITR expansion of the 2022 Mpox virus genome through gene duplication and gene loss. Virus Genes 59 (4), 532–540. doi:10.1007/s11262-023-02002-1
Bui, H. H., Sidney, J., Dinh, K., Southwood, S., Newman, M. J., and Sette, A. (2006). Predicting population coverage of T-cell epitope-based diagnostics and vaccines. BMC Bioinforma. 7, 153. doi:10.1186/1471-2105-7-153
Can, H., Köseoğlu, A. E., Erkunt Alak, S., Güvendi, M., Döşkaya, M., Karakavuk, M., et al. (2020). In silico discovery of antigenic proteins and epitopes of SARS-CoV-2 for the development of a vaccine or a diagnostic approach for COVID-19. Sci. Rep. 10 (1), 22387. doi:10.1038/s41598-020-79645-9
Chen, R. (2012). Bacterial expression systems for recombinant protein production: E. coli and beyond. Biotechnol. Adv. 30 (5), 1102–1107. doi:10.1016/j.biotechadv.2011.09.013
Chou, K. C., and Shen, H. B. (2008). Cell-PLoc: A package of web servers for predicting subcellular localization of proteins in various organisms. Nat. Protoc. 3 (2), 153–162. doi:10.1038/nprot.2007.494
Colovos, C., and Yeates, T. O. (1993). Verification of protein structures: Patterns of nonbonded atomic interactions. Protein Sci. 2 (9), 1511–1519. doi:10.1002/pro.5560020916
Compton, T., Kurt-Jones, E. A., Boehme, K. W., Belko, J., Latz, E., Golenbock, D. T., et al. (2003). Human cytomegalovirus activates inflammatory cytokine responses via CD14 and Toll-like receptor 2. J. Virol. 77 (8), 4588–4596. doi:10.1128/jvi.77.8.4588-4596.2003
Cooper, N. R., and Nemerow, G. R. (1984). The role of antibody and complement in the control of viral infections. J. Invest Dermatol 83 (1 Suppl. l), 121s–127s. doi:10.1111/1523-1747.ep12281847
Dhanda, S. K., Vir, P., and Raghava, G. P. (2013). Designing of interferon-gamma inducing MHC class-II binders. Biol. Direct 8, 30. doi:10.1186/1745-6150-8-30
Dimitrov, I., Bangov, I., Flower, D. R., and Doytchinova, I. (2014). AllerTOP v.2--a server for in silico prediction of allergens. J. Mol. Model 20 (6), 2278. doi:10.1007/s00894-014-2278-5
Dimitrov, I., Flower, D. R., and Doytchinova, I. (2013). AllerTOP--a server for in silico prediction of allergens. BMC Bioinforma. 14 (Suppl. 6), S4. doi:10.1186/1471-2105-14-s6-s4
Doytchinova, I. A., and Flower, D. R. (2007). VaxiJen: A server for prediction of protective antigens, tumour antigens and subunit vaccines. BMC Bioinforma. 8, 4. doi:10.1186/1471-2105-8-4
Du, Z., Su, H., Wang, W., Ye, L., Wei, H., Peng, Z., et al. (2021). The trRosetta server for fast and accurate protein structure prediction. Nat. Protoc. 16 (12), 5634–5651. doi:10.1038/s41596-021-00628-9
Elde, N. C., Child, S., Eickbush, M., Kitzman, J., Rogers, K., Shendure, J., et al. (2012). Poxviruses deploy genomic accordions to adapt rapidly against host antiviral defenses. Cell 150 (4), 831–841. doi:10.1016/j.cell.2012.05.049
Esposito, J. J., Knight, J. C., Shaddock, J. H., Novembre, F. J., and Baer, G. M. (1988). Successful oral rabies vaccination of raccoons with raccoon poxvirus recombinants expressing rabies virus glycoprotein. Virology 165 (1), 313–316. doi:10.1016/0042-6822(88)90692-7
Fleri, W., Paul, S., Dhanda, S. K., Mahajan, S., Xu, X., Peters, B., et al. (2017). The immune epitope Database and analysis resource in epitope discovery and synthetic vaccine design. Front. Immunol. 8, 278. doi:10.3389/fimmu.2017.00278
Fonseca, J. A., McCaffery, J. N., Caceres, J., Kashentseva, E., Singh, B., Dmitriev, I. P., et al. (2018). Inclusion of the murine IgGκ signal peptide increases the cellular immunogenicity of a simian adenoviral vectored Plasmodium vivax multistage vaccine. Vaccine 36 (20), 2799–2808. doi:10.1016/j.vaccine.2018.03.091
Frank, K., and Sippl, M. J. (2008). High-performance signal peptide prediction based on sequence alignment techniques. Bioinformatics 24 (19), 2172–2176. doi:10.1093/bioinformatics/btn422
Garcia, K. C., Teyton, L., and Wilson, I. A. (1999). Structural basis of T cell recognition. Annu. Rev. Immunol. 17, 369–397. doi:10.1146/annurev.immunol.17.1.369
Gigante, C. M., Korber, B., Seabolt, M. H., Wilkins, K., Davidson, W., Rao, A. K., et al. (2022). Multiple lineages of monkeypox virus detected in the United States, 2021-2022. Science 378 (6619), 560–565. doi:10.1126/science.add4153
Gori, A., Longhi, R., Peri, C., and Colombo, G. (2013). Peptides for immunological purposes: Design, strategies and applications. Amino Acids 45 (2), 257–268. doi:10.1007/s00726-013-1526-9
Grote, A., Hiller, K., Scheer, M., Munch, R., Nortemann, B., Hempel, D. C., et al. (2005). JCat: A novel tool to adapt codon usage of a target gene to its potential expression host. Nucleic Acids Res. 33, W526–W531. doi:10.1093/nar/gki376
Gupta, S., Kapoor, P., Chaudhary, K., Gautam, A., Kumar, R., and Raghava, G. P. S. (2015). Peptide toxicity prediction. Methods Mol. Biol. 1268, 143–157. doi:10.1007/978-1-4939-2285-7_7
He, R., Yang, X., Liu, C., Chen, X., Wang, L., Xiao, M., et al. (2018). Efficient control of chronic LCMV infection by a CD4 T cell epitope-based heterologous prime-boost vaccination in a murine model. Cell Mol. Immunol. 15 (9), 815–826. doi:10.1038/cmi.2017.3
Hegde, R. S., and Bernstein, H. D. (2006). The surprising complexity of signal sequences. Trends Biochem. Sci. 31 (10), 563–571. doi:10.1016/j.tibs.2006.08.004
Ismail, S., Abbasi, S. W., Yousaf, M., Ahmad, S., Muhammad, K., and Waheed, Y. (2022). Design of a multi-epitopes vaccine against hantaviruses: an immunoinformatics and molecular modelling approach. Vaccines (Basel) 10 (3), 378. doi:10.3390/vaccines10030378
Jiang, P., Cai, Y., Chen, J., Ye, X., Mao, S., Zhu, S., et al. (2017). Evaluation of tandem Chlamydia trachomatis MOMP multi-epitopes vaccine in BALB/c mice model. Vaccine 35 (23), 3096–3103. doi:10.1016/j.vaccine.2017.04.031
Jones, D. T. (1999). Protein secondary structure prediction based on position-specific scoring matrices 1 1Edited by G. Von Heijne. J. Mol. Biol. 292 (2), 195–202. doi:10.1006/jmbi.1999.3091
Kmiec, D., and Kirchhoff, F. (2022). Monkeypox: a new threat? Int. J. Mol. Sci. 23 (14), 7866. doi:10.3390/ijms23147866
Kovjazin, R., and Carmon, L. (2014). The use of signal peptide domains as vaccine candidates. Hum. Vaccin Immunother. 10 (9), 2733–2740. doi:10.4161/21645515.2014.970916
Kozakov, D., Hall, D. R., Xia, B., Porter, K. A., Padhorny, D., Yueh, C., et al. (2017). The ClusPro web server for protein-protein docking. Nat. Protoc. 12 (2), 255–278. doi:10.1038/nprot.2016.169
Lee, S., Liu, H., Wilen, C. B., Sychev, Z. E., Desai, C., Hykes, B. L., et al. (2019). A secreted viral nonstructural protein determines intestinal norovirus pathogenesis. Cell Host Microbe 25 (6), 845–857 e5. doi:10.1016/j.chom.2019.04.005
Lopez-Blanco, J. R., Aliaga, J. I., Quintana-Ortí, E. S., and Chacón, P. (2014). iMODS: internal coordinates normal mode analysis server. Nucleic Acids Res. 42 (Web Server issue), W271–W276. doi:10.1093/nar/gku339
Lovell, S. C., Davis, I. W., Arendall, W. B., de Bakker, P. I. W., Word, J. M., Prisant, M. G., et al. (2003). Structure validation by cα geometry: ϕ,ψ and cβ deviation. Proteins 50 (3), 437–450. doi:10.1002/prot.10286
Lozano, J. M., and Muller, S. (2023). Monkeypox: Potential vaccine development strategies. Trends Pharmacol. Sci. 44 (1), 15–19. doi:10.1016/j.tips.2022.10.005
Lu, I. N., Farinelle, S., Sausy, A., and Muller, C. P. (2017). Identification of a CD4 T-cell epitope in the hemagglutinin stalk domain of pandemic H1N1 influenza virus and its antigen-driven TCR usage signature in BALB/c mice. Cell Mol. Immunol. 14 (6), 511–520. doi:10.1038/cmi.2016.20
Magnan, C. N., Randall, A., and Baldi, P. (2009). SOLpro: Accurate sequence-based prediction of protein solubility. Bioinformatics 25 (17), 2200–2207. doi:10.1093/bioinformatics/btp386
Marennikova, S. S., Seluhina, E. M., Mal'ceva, N. N., Cimiskjan, K. L., and Macevic, G. R. (1972). Isolation and properties of the causal agent of a new variola-like disease (monkeypox) in man. Bull. World Health Organ 46 (5), 599–611.
Nakai, K. (2000). Protein sorting signals and prediction of subcellular localization. Adv. Protein Chem. 54, 277–344. doi:10.1016/s0065-3233(00)54009-1
Napolitano, F., Xu, X., and Gao, X. (2022). Impact of computational approaches in the fight against COVID-19: An AI guided review of 17 000 studies. Brief. Bioinform 23 (1), bbab456. doi:10.1093/bib/bbab456
Organization, W. H. (2022). Mpox (monkeypox) outbreak. Available at: https://www.who.int/emergencies/situations/monkeypox-oubreak-2022 (Accessed July 25, 2023).
Panicali, D., Davis, S. W., Weinberg, R. L., and Paoletti, E. (1983). Construction of live vaccines by using genetically engineered poxviruses: Biological activity of recombinant vaccinia virus expressing influenza virus hemagglutinin. Proc. Natl. Acad. Sci. U. S. A. 80 (17), 5364–5368. doi:10.1073/pnas.80.17.5364
Parvizpour, S., Pourseif, M. M., Razmara, J., Rafi, M. A., and Omidi, Y. (2020). Epitope-based vaccine design: A comprehensive overview of bioinformatics approaches. Drug Discov. Today 25 (6), 1034–1042. doi:10.1016/j.drudis.2020.03.006
Petersen, E., Kantele, A., Koopmans, M., Asogun, D., Yinka-Ogunleye, A., Ihekweazu, C., et al. (2019). Human monkeypox: epidemiologic and clinical characteristics, diagnosis, and prevention. Infect. Dis. Clin. North Am. 33 (4), 1027–1043. doi:10.1016/j.idc.2019.03.001
Rapin, N., Lund, O., and Castiglione, F. (2011). Immune system simulation online. Bioinformatics 27 (14), 2013–2014. doi:10.1093/bioinformatics/btr335
Reynisson, B., Alvarez, B., Paul, S., Peters, B., and Nielsen, M. (2020). NetMHCpan-4.1 and NetMHCIIpan-4.0: Improved predictions of MHC antigen presentation by concurrent motif deconvolution and integration of MS MHC eluted ligand data. Nucleic Acids Res. 48 (W1), W449–W454. doi:10.1093/nar/gkaa379
Rosano, G. L., and Ceccarelli, E. A. (2014). Recombinant protein expression in Escherichia coli: Advances and challenges. Front. Microbiol. 5, 172. doi:10.3389/fmicb.2014.00172
Saha, S., and Raghava, G. P. (2006). Prediction of continuous B-cell epitopes in an antigen using recurrent neural network. Proteins 65 (1), 40–48. doi:10.1002/prot.21078
Senkevich, T. G., Yutin, N., Wolf, Y. I., Koonin, E. V., and Moss, B. (2021). Ancient gene capture and recent gene loss shape the evolution of orthopoxvirus-host interaction genes. mBio 12 (4), e0149521. doi:10.1128/mbio.01495-21
Shuvo, M. H., Gulfam, M., and Bhattacharya, D. (2021). DeepRefiner: High-accuracy protein structure refinement by deep network calibration. Nucleic Acids Res. 49 (W1), W147–W152. doi:10.1093/nar/gkab361
Smith, G. L., Mackett, M., and Moss, B. (1983a). Infectious vaccinia virus recombinants that express hepatitis B virus surface antigen. Nature 302 (5908), 490–495. doi:10.1038/302490a0
Smith, G. L., Murphy, B. R., and Moss, B. (1983b). Construction and characterization of an infectious vaccinia virus recombinant that expresses the influenza hemagglutinin gene and induces resistance to influenza virus infection in hamsters. Proc. Natl. Acad. Sci. U. S. A. 80 (23), 7155–7159. doi:10.1073/pnas.80.23.7155
Taouk, M. L., Steinig, E., Taiaroa, G., Savic, I., Tran, T., Higgins, N., et al. (2023). Intra- and interhost genomic diversity of monkeypox virus. J. Med. Virol. 95 (8), e29029. doi:10.1002/jmv.29029
Vartak, A., and Sucheck, S. J. (2016). Recent advances in subunit vaccine carriers. Vaccines (Basel) 4 (2), 12. doi:10.3390/vaccines4020012
Vaure, C., and Liu, Y. (2014). A comparative review of toll-like receptor 4 expression and functionality in different animal species. Front. Immunol. 5, 316. doi:10.3389/fimmu.2014.00316
Wilkins, M. R., Gasteiger, E., Bairoch, A., Sanchez, J. C., Williams, K. L., Appel, R. D., et al. (1999). Protein identification and analysis tools in the ExPASy server. Methods Mol. Biol. 112, 531–552. doi:10.1385/1-59259-584-7:531
Keywords: monkeypox virus, antigenicity, reverse vaccinology, immune simulation, molecular dynamic simulation
Citation: Rehman Z, Fahim A and Irtash M (2025) Sculpting multi-epitope vaccine against Monkeypox viral strains using immunoinformatics. Acta Virol. 68:13542. doi: 10.3389/av.2024.13542
Received: 16 July 2024; Accepted: 13 December 2024;
Published: 20 January 2025.
Edited by:
Gnanavel Venkatesan, Indian Veterinary Research Institute (IVRI), IndiaReviewed by:
Nitin Atre, National Institute of Virology (ICMR), IndiaYang Li, Harbin Medical University, China
Copyright © 2025 Rehman, Fahim and Irtash. This is an open-access article distributed under the terms of the Creative Commons Attribution License (CC BY). The use, distribution or reproduction in other forums is permitted, provided the original author(s) and the copyright owner(s) are credited and that the original publication in this journal is cited, in accordance with accepted academic practice. No use, distribution or reproduction is permitted which does not comply with these terms.
*Correspondence: Zaira Rehman, emFpcmEucmVobWFuQHRpaC5vcmcucGs=