- 1Department of Biomedical Engineering, University of California, Irvine, Irvine, CA, United States
- 2Department of Electrical Engineering and Computer Science, University of California, Irvine, Irvine, CA, United States
- 3Children’s Health Orange County, Orange, CA, United States
Classical models of the physiology of dystonia suggest that involuntary muscle contractions are caused by inappropriately low activity in Globus Pallidus internus (GPi) that fails to adequately inhibit thalamic inputs to cortex. We test this prediction in three children with primary dystonia undergoing depth electrode recording in basal ganglia and thalamus during selection of targets for deep brain stimulation (DBS) implantation. We compare muscle activity to the power in the spectrogram of the local field potential, as well as to counts of identified spikes in GPi, subthalamic nucleus (STN), and the Ventral oralis (VoaVop) and Ventral Anterior (VA) subnuclei of the thalamus, while subjects are at rest or attempting to make active voluntary arm or leg reaching movements. In all three subjects, both spectrogram power and spike activity in GPi, STN, VoaVop, and VA are significantly positively correlated with movement. In particular, GPi and STN both increase activity during attempted movement. These results contradict the classical rate model of the physiology of dystonia, and support more recent models that propose abnormalities in the detailed pattern of activity rather than the overall lumped activity of pallidum and thalamus.
Introduction
The classical “rate” model of basal ganglia physiology in dystonia developed by Albin and others has been built upon the known anatomical connections between striatum, pallidum, subthalamus, and thalamus (1–3). Inhibitory projection neurons originating in striatum and pallidum will hyperpolarize their targets in pallidum, subthalamus, and thalamus, while excitatory projection neurons in subthalamus and thalamus will depolarize their targets in pallidum and cortex. In primates, there is a division into direct and indirect pathways. The direct pathway includes inhibitory projections from striatum to Globus Pallidus internus (GPi) and from GPi to thalamus, followed by excitatory projections from thalamus to cortex. The indirect pathway includes inhibitory projections from striatum to Globus Pallidus externus (GPe), from GPe to subthalamic nucleus (STN), and from GPi to thalamus, with excitatory projections from STN to GPi. Modifications of this model over the past decades have recognized the important role of a “hyperdirect” pathway from cortex to STN, as well as pathways from STN to GPe, GPi to STN, and medial thalamic nuclei to striatum. In all models, the final common pathway linking basal ganglia to thalamus remains the inhibitory projection from GPi to thalamus including the ventral oralis anterior and posterior (VoaVop) and ventral anterior (VA) subnuclei (4, 5).
The patterns of excitatory and inhibitory connections suggest what has been referred to as the classical “rate” model. This simplicity of this model has led to widespread adoption, and it has proven useful in the prediction of the physiology of Parkinson’s disease, in which high firing rates in GPi are thought to cause inappropriate inhibition of thalamic outputs to cortex, thus leading to delayed movement initiation and decreased speed of movement (bradykinesia) (2). The finding of relatively lower firing rates in GPi during voluntary movement in some patients with dystonia has led to the suggestion that dystonia is due to the opposite problem: failure of inhibition of thalamic outputs to cortex (6), thus potentially leading to excessive involuntary muscle contractions (such as overflow, co-contraction, or hyperkinetic movements) (1, 7). This theory has been supported in recordings from healthy non-human primates showing that GPi activity is typically very high. It has also been supported by the observation that electrical stimulation in GPi can ameliorate symptoms in patients with dystonia.
However, important discrepancies in the application of the classical model to dystonia have been noted (7, 8). Perhaps the most significant is the observation that lesions in GPi also lead to amelioration of dystonia, whereas the model would predict that lesions would worsen dystonia. Another discrepancy is that if reduction in activity in GPi initiates movement in the healthy brain, then there is no mechanism for initiation of activity in a brain with baseline low activity in GPi. Another discrepancy is the observation that dystonic muscle activity is almost always caused by or worsened by active attempts at movement, whereas the classical model would predict the presence of dystonia at rest. And an important missing element of some models is the absence of a depolarizing (excitatory) input to thalamus (1); without such an input, thalamus will never activate and the hyperpolarizing (inhibitory) inputs from GPi will have no effect (3).
We examine the predictions of the classical rate model by making simultaneous recordings from high-impedance electrodes in GPi, STN, VoaVop, and VA in three children with primary dystonia. Recordings are performed as part of a previously-reported novel targeting method in which temporary externalized stereo electroencephalography (sEEG) depth electrodes are implanted in potential DBS targets, and children are tested with stimulation and recording while awake and unrestrained (9, 10). This method eliminates the effects of restrained movement (in the operating room with a headframe) and sedation and partial anesthesia, and permits prolonged testing with children awake, unrestrained, unmedicated, and performing routine tasks of daily living in a hospital room with parents and clinical staff present. Clinical outcomes from this targeting method have been reported elsewhere. Here we utilize recorded data obtained from the sEEG electrodes during voluntary arm or leg reaching movements in three children who previously underwent this procedure.
Between one and ten high-impedance (70–90 kΩ) contacts are present within each of the tested nuclei. Recordings are analyzed both in the frequency domain using the power spectral density (spectrogram) and in the time domain using analysis of the spike rate. Spectrogram power and spike rates are compared between rest and active movement.
Methods
Patient selection
Patients were selected for DBS surgery after being diagnosed with primary (genetic) dystonia by a pediatric movement disorder specialist (TDS) based on established criteria (11–13). All patients had confirmed failure of symptomatic medical therapies at adequate dosing, and potential stimulation target(s) were identifiable with magnetic resonance imaging (MRI). Patients or parents of minor patients provided Health Insurance Portability and Accountability Act (HIPAA) authorization for the research use of protected health information and written informed consent for surgical procedures conforming to standard hospital practice, and for research use of electrophysiological data before the procedure. The research use of data was approved by the institutional review board of the Children’s Health, Orange County (CHOC) hospital. All surgical procedures and experiments took place at Children’s Health Orange County (CHOC). In this study, we report data from three children with primary dystonia who have undergone DBS surgery in the Neuromodulation Unit (NMU). See Table 1 for subject demographics.
Depth recordings
Up to 10 temporary AdTech MM16C depth electrodes (AdTech Medical Instrument Corp., Oak Creek, WI, United States) were implanted in potential DBS targets using procedures reported previously (9). Potential stimulation targets were identified based on previous studies of clinical efficacy. Targets are named based on Hassler’s terminology, and include subthalamic nucleus (STN), globus pallidus internus (GPi), in basal ganglia, ventral intermediate nucleus (VIM), ventral oralis anterior/posterior (VoaVop), and ventral anterior nucleus (VA), in thalamus. Each implanted electrode has ten high-impedance (70–90 kΩ) micro contacts (50-µm diameter) that are arranged in groups of two or three, spaced evenly around the circumference of the electrode shaft in four rows. The leads were connected to Adtech Cabrio™ connectors that include a custom unity-gain preamplifier for each micro contact to reduce noise and motion artifacts. All data used in this study were recorded at the micro contacts and sampled at 24 kHz by a custom recording system consisting of a PZ5M 256-channel digitizer, RZ2 processor, and RS4 high speed data storage (TDT, Tucker-Davis Technologies Inc., Alachua, FL, United States).
In addition to local field potential (LFP) recordings, we recorded muscle activity from 16 surface electromyography (EMG) Trigno™ Avanti (Delsys Incorporated, Natick, MA) sensors, attached to upper and lower extremities. We recorded EMG signals from biceps, triceps, flexor carpi ulnaris, extensor carpi radialis, gastrocnemius, tibialis, quadriceps, and hamstrings bilaterally, to track the changes in muscles activity with the brain activity. All EMG signals were sampled at 2 kHz and the EMG system was synchronized with the TDT using a pulse generator.
Movement task
Patients were asked to perform a voluntary reach to target task with their less impaired upper limb while depth electrode and EMG signals were recorded. In all cases, dystonia was present in the tested limb, as evidenced by the presence of dystonic postures interfering with expected performance of the task. The reaching task consisted of a one-to-two-minute period of voluntary reaching followed by 30 seconds of rest, for a total of four to six consecutive trials. Movement and rest onset and offset times were marked during the experiment. All data analysis was performed in MATLAB R2020a (The MathWorks, Inc., Natick, MA, United States).
EMG signal processing
EMG signals were notch-filtered to eliminate 60 Hz interference and band-pass filtered between 20 and 500 Hz. They were then rectified and low pass filtered at 1 Hz to extract the envelope of the EMG signal. Fourth-order Butterworth filters were used throughout.
Time-frequency analysis of LFP recordings
The microelectrode LFP recordings were notch-filtered at 60 Hz and the first five harmonics. They were then high pass filtered at 1 Hz to remove drift. The voltage difference between each pair of micro contacts in each of the four rows of a depth electrode were computed to remove common noise from the signals and reveal the underlying activity (bipolar montage) which results in eight different recordings per lead.
To compute the spectrograms of the recorded non-stationary signals, each signal was divided into segments with sixty percent overlap using a Kaiser window with time resolution of 3 seconds, and 0.7 leakage factor. Next, signals were transformed into the frequency domain using a short-time Fourier transform. Finally, the spectra of each segment were computed to build the spectrograms. The spectrograms were computed in two frequency ranges of 1–13 Hz (delta, theta, and alpha) and 13–35 Hz (beta). This specific frequency ranges are chosen as they are of standard frequency bands used in frequency analysis of brain activity. Additionally, the alpha and beta bands are important in other movement disorders such as Parkinson’s disease, which involves abnormality in alpha and beta band oscillations (7).
Spike analysis of LFP recordings
Spike analysis was performed on the micro contact LFP recordings, using the bipolar montage, explained earlier. Signals are bandpass filtered between 350 and 3,000 Hz using an 8th order Butterworth filter. A non-linear energy operator (NEO) (14) was applied to the data to aid in spike detection by enhancing sharp peaks in bipolar LFP signals:
where x(t) is a sample of the waveform at time t and y(t) is the enhanced output. Spikes are characterized by localized high frequencies and sharp increases in instantaneous energy. These features are captured in the magnitude of the NEO, which is large only when the signal is high in power [
Peak detection was performed on the NEO, where the amplitudes of detected peaks were between three and seventy times the standard deviation of the noise. Following event detection, wavelet decomposition was used to extract features from detected events and a Gaussian Mixture Model (GMM) was used to cluster detected events. Events with low probability of belonging to any clusters were removed. Events associated with the same cluster were identified as a “spike” belonging to the same originating neuron(s), and average spike rates were determined within the relevant intervals (movement or rest).
Because the impedance of the recording contacts is less than 100 kΩ, it is likely that the identified spikes correspond to multiple neuron clusters rather than to single units that would be recorded with higher impedance electrodes. Additionally, the spike clusters are identified by consistent shapes. If there were variability in the cluster, the overall shape would vary as well. A spike cluster likely represents a stereotyped firing pattern from a group of nearby neurons. Nevertheless, the spike rates are likely to parallel the neural activity that would be obtained from higher impedance recordings.
Statistical analysis
To measure the effect of movement on: 1) the average power in two frequency bands, and 2) average spike rate, a linear mixed effects model with repeated measures was fitted to both spike firing rate and power in each band. Repeated measures for the time-frequency analysis are the average power in each frequency band for every 5 s of each period of voluntary reaching and rest. Repeated measures for the spike analysis are the average firing rates for each voluntary reaching and rest period.
The model for the time frequency analysis consists of condition (active versus rest), frequency range ([1–13] and [13–35] Hz), and their interactions as fixed effects. Subject intercept was included as a random effect, and by-subject random slopes were the frequency range.
The model for average spike rate analysis consists of the condition as the fixed effect, while the random effect was assumed to be the subject intercept. All the statistical analyses were done using the lme4 (15) and emmeans (16) packages in R-studio (R core team, 2021).
Results
Figure 1 shows time-frequency and spike analyses of LFP recordings for subject 1. Raw bipolar LFP recordings, spectrograms, and spike rasters are displayed from top to bottom for contralateral GPi, STN, VoaVop, and VA. Figure 1 also includes an example EMG recording from the left biceps to highlight the voluntary reaching and rest period. Results from the spectrogram analysis suggest a clear trend in power, where power is increased during the reaching task in frequencies from 1 to 35 Hz and decreased in rest periods in all target locations. In particular, power starts to increase as the patient starts one period of voluntary reaching and subsequently decreases as the patient is in rest. Additionally, raster plots in all target locations illustrate increased spiking activity in voluntary reaching task periods in comparison to resting states. The observations are consistent among all the three subjects.
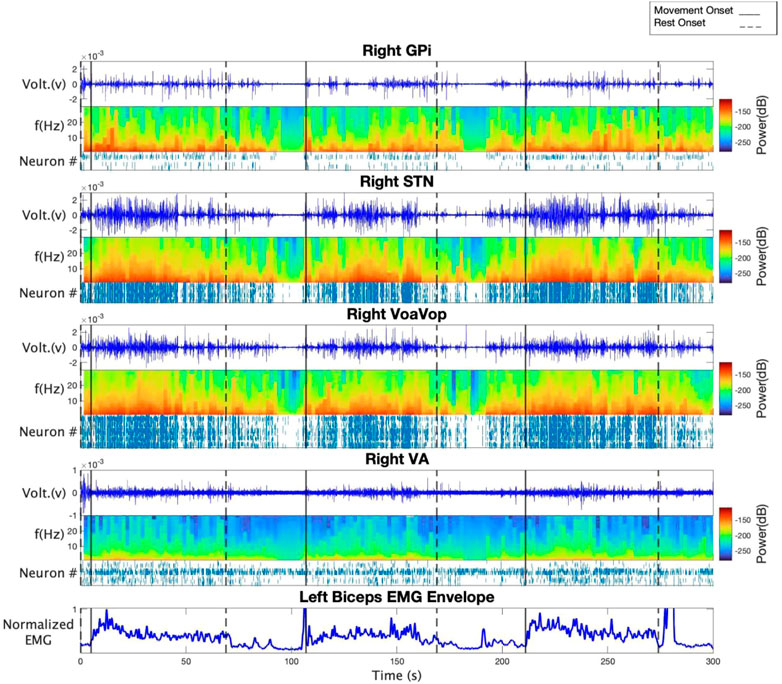
FIGURE 1. From top to bottom: raw LFP recording, spectrogram, and spike raster of GPi, STN, VoaVop, and VA for subject 1. The bottom plot demonstrates left biceps EMG recording as an example in order to highlight voluntary reaching task and rest periods. Spectrogram results show increased power in voluntary reaching task periods compared to resting periods. Spike rasters show increased neural activity in voluntary reaching task periods compared to resting periods.
The linear mixed effects model was fitted on data from each brain region separately for group analysis. The explained variance of the models are shown in Table 2. We performed a pairwise comparison between the rest versus the active state to test for significance of increases in firing rates and power for each frequency range. Analysis of variance (ANOVA) using type II Wald chi-square test, with 95% confidence interval was performed on the linear models, and showed a significant difference between the active versus the resting state (Pr(>chisq) < 0.0001) for all brain regions tested.
We then computed the log of the ratio between active and resting state powers for each frequency range. This measure is significantly different from zero for both frequency ranges. The results are shown in Figure 2 for each patient. The average spike rates in both active and resting states for all three patients are shown in Figure 3.
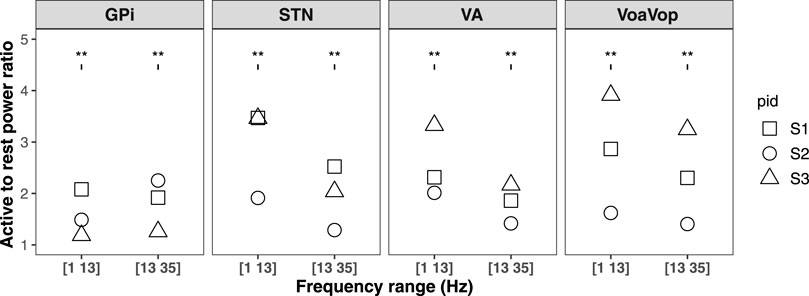
FIGURE 2. Ratio of active to rest average power in frequency ranges 1–13 Hz and 13–35 Hz for three patients are shown by different shapes in GPi, STN, VA, and VoaVop. The group analysis shows that log power ratio is significant for all of them.
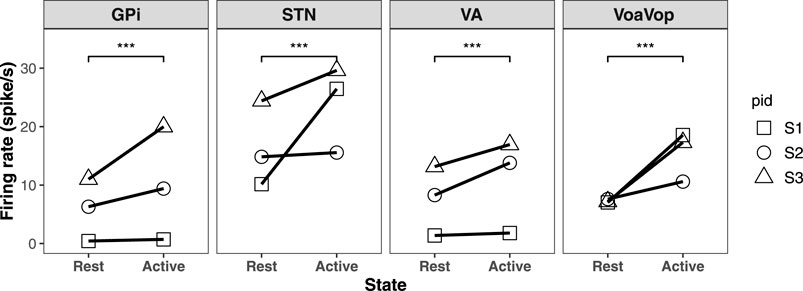
FIGURE 3. The average firing rate of resting versus voluntary reaching state for three subjects and the regression line in GPi, STN, VA, and VoaVop. The group analysis shows a significant increased firing rate during voluntary reaching period in GPi, STN, VA, and VoaVop.
Discussion
The classical rate model of basal ganglia function predicts that STN and GPi activity will be low and thalamic activity will be high during movement, and that STN and GPi activity will be high and thalamic activity will be low at rest (2, 17). While the predictions for VoaVop and VA subnuclei of the thalamus are supported by our results, the predictions for STN and GPi are contradicted. Our results are in agreement with prior results in monkeys showing increases in GPi activity from rest to movement, with maintained high GPi activity during movement and voluntary posture (18–21).
The rate model is known to have significant problems when applied to dystonia (7, 8), and we suggest several possible ways to address the results shown by our data. Most importantly, the classical model is incomplete because it does not posit a stimulatory (depolarizing) input to thalamus, and without excitation of thalamus, GPi inhibition would have no effect (There is no point in inhibiting a neuron that never fires.) Although GPi and GPe also receive primary inhibitory input from both striatum and pallidum, both structures also receive excitatory input from STN. The primary excitatory input to thalamus arises from cerebral cortex, and we suggest that augmenting the model with an excitatory cortical-thalamic-cortical loop provides an explanation for the increased thalamic firing during movement (7). In this case, the role of the GPi is to selectively inhibit this loop at the thalamus, effectively “sculpting” the thalamo-cortical loop. This mechanism is supported by lesion studies showing involuntary antagonist muscle activity resulting from lesions of GPi (22, 23). Dystonia could arise, similarly to the classical model, from insufficient inhibition of parts of this loop. In this augmented model, GPi would only need to inhibit the loop when thalamus is activated, consistent with our results. The deficit in dystonia would then be either insufficient inhibition (GPi increases rate with movement, but not enough) or an incorrect pattern of inhibition (7, 24) (GPi increases rate with movement but does not properly sculpt the thalamic activity). This proposal is consistent with the finding of abnormal sensory responses in thalamus, consistent with failure of inhibition of cortical inputs (25).
It is worth noting that the suggested dichotomy in which Parkinsonism represents decreased activity while dystonia represents increased activity is an oversimplification that may also be contributing to contradictions with the classical model (6). Although dystonia was originally classified as a hyperkinetic disorder, it shares features common to both hyperkinetic disorders (dyskinesia, Huntington’s chorea) and hypokinetic disorders (rigidity, bradykinesia) (7). In children, a combination of parkinsonism and dystonia is typically present in conditions of low dopamine (e.g., dopa-responsive dystonia) or diffuse hypoxic injury (e.g., dyskinetic cerebral palsy) (26). EMG recordings from both Parkinsonism and dystonia demonstrate slow rates of contraction and change in contraction levels (27, 28) and both Parkinsonism and dystonia have hyperkinetic components that can include tremor and dyskinesia. Therefore, while electrophysiological data continue to support increased activity in GPi in parkinsonism compared with dystonia, both disorders may include a failure of appropriate inhibition of thalamo-cortical loops that includes both insufficient inhibition of unwanted movement and insufficient disinhibition of intended movement. Such similarities would be consistent with the observation that DBS in either GPi or STN as well as dopaminergic medication can ameliorate symptoms in both disorders.
Our results appear to contradict recordings from human and non-human primate models of dystonia that typically show very high GPi activity at rest (1), inconsistent with the rate model. We suggest three hypotheses to explain this discrepancy. First, it is possible that non-human primates and humans have different patterns of activity in basal ganglia. Second, we only obtain recordings from human patients with severe disease, whereas the non-human primate recordings are typically made in healthy animals. Third, a non-human primate strapped into a recording apparatus with head immobilized may not be truly at rest, and ongoing isometric force exerted against the seating system and head immobilization system may not always be evident but could nevertheless lead to baseline activation of deep brain regions associated with movement or posture.
The results presented here include several important weaknesses. First, we tested only three children, and although all three have a genetic origin of their dystonia, the specific mutations are different. This suggests that the phenomena we observed are not confined to a single cause of primary dystonia, but whether this is a general feature of all patients (children and adults) with these or other forms of dystonia is not known. Second, we tested only reaching movements, and it is possible that patterns seen during other activities such as ambulation, speaking, eating, or isometric movements would be different. Third, the specific electrodes we use have impedances (70–90 kΩ) that are much less than typically used for extracellular recording (typically 1 MΩ or more) and thus could lead to different phenomena. In particular, the “spikes” that we observe probably do not represent single neuron depolarizations and are likely to be formed from stereotyped firing patterns of groups of neurons near the recording contacts. Fourth, we cannot determine the relationship between GPi firing and dystonic components of movement because it is not possible to separate dystonic from voluntary components of movement in our paradigm. From a theoretical point of view, our results do not shed light on the involvement of dopamine (29), sensory pathways (30–33), cerebellum (34–36), or other structures in the causes of dystonia.
Despite these limitations, our results support an overall increase in GPi firing from rest to movement in children with primary dystonia. This is an important observation for understanding the nature of dystonia and the mechanism of function of interventions including medications and DBS surgery. This finding is consistent with the hypothesis that it is the pattern of GPi outputs rather than the average firing rate that is abnormal in dystonia (7, 24), and that this pattern may include both inappropriate inhibition of desired thalamic activity as well as inappropriate disinhibition of unwanted thalamic activity. Confirmation of this hypothesis in a larger cohort of patients with more varied causes of dystonia will be important for further understanding and improved treatment of this debilitating disorder.
Data availability statement
The data analyzed in this study is subject to the following licenses/restrictions: The datasets were collected as part of DBS procedure for clinical and research purposes. They are considered protected health information under HIPAA. De-identified datasets may be available on request. Requests to access these datasets should be directed to Terence Sanger, dGVycnlAc2FuZ2VybGFiLm5ldA==.
Ethics statement
The studies involving human participants were reviewed and approved by CHOC Institutional Review Board—Research Institute. Written informed consent to participate in this study was provided by the participants’ legal guardian/next of kin.
Author contributions
MK, SJ, JN, SS, and TS contributed to data acquisition, data analysis, data interpretation, and writing the manuscripts. MK contributed to statistical analysis. TS conceived the study. All authors have read and agreed to the published version of the manuscript.
Funding
This study is funded by Cerebral Palsy Alliance Research Foundation (PG02518) and The Crowley-Carter Foundation (CCF-562490).
Conflict of interest
The authors declare that the research was conducted in the absence of any commercial or financial relationships that could be construed as a potential conflict of interest.
Acknowledgments
We thank our volunteers and their parents for participating in this study. We also thank Jennifer Maclean for her assistance with neurologic examinations, as well as Jessica Vidmark for helping in data collection. We would also like to thank the reviewers for their insights.
References
1. Vitek, JL, Chockkan, V, Zhang, JY, Kaneoke, Y, Evatt, M, DeLong, MR, et al. Neuronal activity in the basal ganglia in patients with generalized dystonia and hemiballismus. Ann Neurol (1999) 46:22–35. doi:10.1002/1531-8249(199907)46:1<22:aid-ana6>3.0.co;2-z
4. Devito, JL, and Anderson, MEE. An autoradiographic study of efferent connections of the globus pallidus in Macaca mulatta. Exp Brain Res (1982) 46:107–17. doi:10.1007/BF00238104
5. Penney, JB, and Young, AB. GABA as the pallidothalamic neurotransmitter: Implications for basal ganglia function. Brain Res (1981) 207:195–9. doi:10.1016/0006-8993(81)90693-4
6. Brüggemann, N. Contemporary functional neuroanatomy and pathophysiology of dystonia. J Neural Transm (2021) 128:499–508. –508 Preprint at. doi:10.1007/s00702-021-02299-y
7. Vitek, JL. Pathophysiology of dystonia: A neuronal model. Move Disord (2002) 17:S49–S62. Preprint at. doi:10.1002/mds.10142
8. Obeso, JA, and Lanciego, JL. Past, present, and future of the pathophysiological model of the basal ganglia. Front Neuroanat Preprint (2011) 5:39. doi:10.3389/fnana.2011.00039
9. Sanger, TD, Liker, M, Arguelles, E, Deshpande, R, Maskooki, A, Ferman, D, et al. Pediatric deep brain stimulation using awake recording and stimulation for target selection in an inpatient neuromodulation monitoring unit. Brain Sci (2018) 8:135. doi:10.3390/brainsci8070135
10. Sanger, TD, Robison, A, Arguelles, E, Ferman, D, and Liker, M. Case report: Targeting for deep brain stimulation surgery using chronic recording and stimulation in an inpatient neuromodulation monitoring unit, with implantation of electrodes in GPi and vim in a 7-year-old child with progressive generalized dystonia. J Child Neurol (2018) 33:776–83. doi:10.1177/0883073818787741
11. Albanese, A, Bhatia, K, Bressman, SB, Delong, MR, Fahn, S, Fung, VSC, et al. Phenomenology and classification of dystonia: A consensus update. Move Disord (2013) 28:863–73. –873 Preprint at. doi:10.1002/mds.25475
12. Sanger, TD, Delgado, MR, Gaebler-Spira, D, Hallett, M, and Mink, JW. Classification and definition of disorders causing hypertonia in childhood. Pediatrics (2003) 111(1):e89–e97. doi:10.1542/peds.111.1.e89
13. Sanger, TD, Chen, D, Fehlings, DL, Hallett, M, Lang, AE, Mink, JW, et al. Definition and classification of hyperkinetic movements in childhood. Move Disord (2010) 25:25 1538–49. Preprint at. doi:10.1002/mds.23088
14. Kaiser, JF. On a simple algorithm to calculate the ‘energy’ of a signal. In: ICASSP, IEEE international conference on acoustics, speech and signal processing - proceedings, 1 381–384. Publ by IEEE (1990).
15. Bates, D, Mächler, M, Bolker, BM, and Walker, SC. Fitting linear mixed-effects models using lme4. J Stat Softw (2015) 67. doi:10.18637/jss.v067.i01
16. Searle, SR, Speed, FM, and Milliken, GA. Population marginal means in the linear model: An alternative to least squares means. Am Statistician (1980) 34:216–21. doi:10.2307/2684063
17. Penney, JB, and Young, AB. Striatal inhomogeneities and basal ganglia function. Move Disord (1986) 1:3–15. –15 Preprint at. doi:10.1002/mds.870010102
18. Mink, JW, and Thach, T. Basal ganglia motor control. II. Late pallidal timing relative to movement onset and inconsistent pallidal coding of movement parameters. J Neurophysiol (1991) 65(2):301–29. doi:10.1152/jn.1991.65.2.301
19. Mink, JW, and Thach, T. Basal ganglia motor control. I. Nonexclusive relation of pallidal discharge to five movement modes. J Neurophysiol (1991) 65(2):273–300. doi:10.1152/jn.1991.65.2.273
20. Georgopoulos, AP, Delong, MR, and Crutcher3, MD. Relations between parameters of step-tracking movements and single cell discharge in the globus pallidus and subthalamic nucleus of the behaving monkey’. J Neurosci (1983) 3:1586–98. doi:10.1523/JNEUROSCI.03-08-01586.1983
21. Mitchell, SJ, Richardson, RT, Baker, FH, and Delong, MR. The primate nucleus basalis of meynert: Neuronal activity related to a visuomotor tracking task. Exp Brain Res (1987) 68:506–15. doi:10.1007/BF00249794
22. Mink, JW, and Thach, T. Basal ganglia intrinsic circuits and their role in behavior. Curr Opin Neurobiol (1993) 3:950–7. doi:10.1016/0959-4388(93)90167-w
23. Thach, WT. Basal ganglia motor control. III. Pallidal ablation: Normal reaction time, muscle cocontraction, and slow movement. JOURNALOF NEUROPHYSIOLOGY (2019) 65.
24. Mink, JW. The basal ganglia: Focused selection and inhibition of competing motor programs. Pergamorr Prog Neurobiol (1996) 50:381–425. doi:10.1016/s0301-0082(96)00042-1
25. Lenz, FA. Thalamic single neuron activity in patients with dystonia: Dystonia-related activity and somatic sensory reorganization (1999).
26. Sanger, TD. Annual review of neuroscience basic and translational neuroscience of childhood-onset dystonia: A control-theory perspective (2018). doi:10.1146/annurev-neuro-080317
27. Fujita, K, and Eidelberg, D. Imbalance of the direct and indirect pathways in focal dystonia: A balanced view. Brain (2017) 140:3075–7. doi:10.1093/brain/awx305
28. Malfait, N, and Sanger, TD. Does dystonia always include co-contraction? A study of unconstrained reaching in children with primary and secondary dystonia. Exp Brain Res (2007) 176:206–16. doi:10.1007/s00221-006-0606-4
29. Ribot, B, Aupy, J, Vidailhet, M, Mazere, J, Pisani, A, Bezard, E, et al. Dystonia and dopamine: From phenomenology to pathophysiology. Prog Neurobiol (2019) 182:101678. Preprint at. doi:10.1016/j.pneurobio.2019.101678
30. Conte, A, Defazio, G, Hallett, M, Fabbrini, G, and Berardelli, A. The role of sensory information in the pathophysiology of focal dystonias. Nat Rev Neurol (2019) 15:224–33. –233 Preprint at. doi:10.1038/s41582-019-0137-9
31. Sanger, TD, and Kukke, SN. Abnormalities of tactile sensory function in children with dystonic and diplegic cerebral palsy. J Child Neurol (2007) 22:289–93. doi:10.1177/0883073807300530
32. Sanger, TD, Pascual-Leone, A, Tarsy, D, and Schlaug, G. Nonlinear sensory cortex response to simultaneous tactile stimuli in writer’s cramp. Move Disord (2002) 17:17 105–11. Preprint at. doi:10.1002/mds.1237
33. Sanger, TD, Tarsy, D, and Pascual-Leone, A. Abnormalities of spatial and temporal sensory discrimination in writer’s cramp. Move Disord (2001) 16 94–9. Preprint at. doi:10.1002/1531-8257(200101)16:1<94:AID-MDS1020>3.0.CO;2-O
34. Fung, WKW, and Peall, KJ. What is the role of the cerebellum in the pathophysiology of dystonia? J Neurol (2019) 266:1549–51. doi:10.1007/s00415-019-09344-7
35. Shakkottai, VG, Batla, A, Bhatia, K, Dauer, WT, Dresel, C, Niethammer, M, et al. Current opinions and areas of consensus on the role of the cerebellum in dystonia. Cerebellum (2017) 16 577:577–94. –594 Preprint at. doi:10.1007/s12311-016-0825-6
Keywords: deep brain stimulation, basal ganglia, thalamus, primary dystonia, electrophysiology, rate model
Citation: Kasiri M, Javadzadeh S, Nataraj J, Seyyed Mousavi SA and Sanger T (2023) Correlated activity in globus pallidus and thalamus during voluntary reaching movement in three children with primary dystonia. Dystonia 2:11117. doi: 10.3389/dyst.2023.11117
Received: 09 December 2022; Accepted: 13 February 2023;
Published: 23 February 2023.
Edited by:
Aasef Shaikh, Case Western Reserve University, United StatesCopyright © 2023 Kasiri, Javadzadeh, Nataraj, Seyyed Mousavi and Sanger. This is an open-access article distributed under the terms of the Creative Commons Attribution License (CC BY). The use, distribution or reproduction in other forums is permitted, provided the original author(s) and the copyright owner(s) are credited and that the original publication in this journal is cited, in accordance with accepted academic practice. No use, distribution or reproduction is permitted which does not comply with these terms.
*Correspondence: Terence Sanger, dGVycnlAc2FuZ2VybGFiLm5ldA==