- 1Division of Gastrointestinal and Minimally Invasive Surgery, Department of Surgery, Carolinas Medical Center, Charlotte, NC, United States
- 2Department of Plastic and Reconstructive Surgery, Ohio State University Wexner Medical Center, Columbus, OH, United States
- 3Department of Computer Science, University of North Carolina at Charlotte, Charlotte, NC, United States
Objective: Hernia recurrence and surgical site infection (SSI) are grave complications in Abdominal Wall Reconstruction (AWR). This study aimed to develop multicenter deep learning models (DLMs) developed for predicting surgical complexity, using Component Separation Technique (CST) as a surrogate, and the risk of surgical site infections (SSI) in AWR, using preoperative computed tomography (CT) images.
Methods: Multicenter models were created using deidentified CT images from two tertiary AWR centers. The models were developed with ResNet-18 architecture. Model performance was reported as accuracy and AUC.
Results: The CST model underperformed with an AUC of 0.569, while the SSI model exhibited strong performance with an AUC of 0.898.
Conclusion: The study demonstrated the successful development of a multicenter DLM for SSI prediction in AWR, highlighting the impact of patient factors over surgical practice variability in predicting SSIs with DLMs. The CST model’s prediction remained challenging, which we hypothesize reflects the subjective nature of surgical decisions and varying institutional practices. Our findings underscore the potential of AI-enhanced surgical risk calculators to risk stratify patients and potentially improve patient outcomes.
Introduction
Recent advances in artificial intelligence (AI) have demonstrated remarkable capabilities in the diagnosis and characterization of pathologies through computed tomography (CT) images, underscoring its potential as an indispensable tool in the surgical decision-making process [1–4]. Particularly in abdominal wall reconstruction (AWR), AI’s predictive power promises to enhance operative planning and patient counseling, thus potentially improving the overall quality of care. In prior research on AWR, our team successfully developed and internally validated image-based deep learning models (DLMs) designed to anticipate the level of surgical complexity and the risk of surgical site infections (SSI) [5]. This innovation was the first of its kind, utilizing preoperative CT imaging to foresee the likelihood of requiring a component separation technique (CST), which is a proxy for operative complexity, and predicting surgical site infection (SSI).
The AI model’s proficiency in drawing from preoperative imaging to predict intraoperative events and postoperative outcomes signals a leap toward personalized surgical risk assessment and precision medicine that has been lacking in the field [1, 2, 6, 7]. First, AI in AWR will help surgeons identify patients who are at risk for a complex surgical operation in addition to postoperative complications. Successful implementation of such a model will allow appropriate triage of the patient to the proper surgeon, whether that is local to them, or at a tertiary hernia center. Additionally, the surgeon will be able to evaluate each patient’s preoperative risk of complications, including SSI, and therefore be better able to counsel patients, obtain preoperative optimization, and prepare for intraoperative decision making. Particularly in AWR, this means accomplishing a low recurrence rate and low rate of postoperative surgical site occurences. Achieving these outcomes not only benefits the patient but also the hospital system as a whole [8]. The financial cost of complications in AWR is staggering, and reducing recurrence rates by 1% was estimated to save $139.9 million annually [8–10]. Given the annual incidence of around 611,000 AWR cases, optimization of outcomes has the potential to greatly reduce hospital resource utilization in the United States [9–12]. As previously discussed, the push for establishing AWR tertiary centers is ongoing [13–16], but empowering community general surgeons and equipping specialists alike with tools to optimize outcomes will have far reaching benefits.
The true test of any AI-based model’s utility and generalizability lies in its ability to obtain external validity [17]. This is the foundation to evaluate the transferability and reliability of the DLMs predictions to external cohorts and ensures that the models perform well when confronted with the variability inherent to different surgical practices and patient populations [7]. Therefore, the aim of the current study was to construct a multicenter model and test its performance.
Methods
Study Design
Study design and result reporting were based on the Transparent Reporting of a Multivariable Prediction Model for Individual Prognosis or Diagnosis (TRIPOD) reporting guidelines [18]. With institutional review board approval and a joint data sharing agreement, a multicenter DLM was developed. One center used the original CST and SSI images employed by Elhage et al [5] in the development of an internally validated model. The other center’s images were obtained from a cohort of 75 patients, who were treated by an AWR specialist at a tertiary center in a different region of the United States. Both patient groups underwent preoptimization including smoking cessation for a minimum of 4 weeks, preoperative weight-loss, and reduction of HgbA1c to less than 7.2 mg/dL [19, 20]. Patients whose CT scans with scatter (secondary to orthopedic prosthetics, for example,) that limited the algorithm’s interpretation of the image were excluded from model training. Additionally, those who had a chemical component relaxation with botulinum toxin A injection were excluded, as this would alter the rate of CST performed on large, loss of domain, hernias. A CST was either an anterior or poster myofascial release that was either unilateral or bilateral. CST technique and algorithm varied between institutions [21, 22]. Both institutions perform a step-up approach of an anterior or posterior CST. The patients were reported as having a CST if any portion of the CST procedure was performed, even if a full musculofascial release was not performed. SSI was defined as a deep or superficial wound infection. A deep infection included a deep space or mesh infection, whereas a superficial infection included a subcutaneous infection or cellulitis [23].
Development and Validation of DLM
CST and SSI prediction models were built from the original internal dataset with the established ResNet-18 architecture using PyTorch software version 1.13.1 [24]. The model architecture is comprised of 18 unique layers that include the initial convolutional layer, four sets of four convolutional layers of similar filter size, and finally a fully connected layer. ResNet-18 architecture uses the stochastic gradient descent optimizer and the sparse binary cross-entropy loss function for model training [25]. Finally, transfer learning was performed using pretrained model weights for ResNet-18 on the ImageNet database.
Model consistency was assessed using Leave-One-Out Cross-Validation (LOOCV) and k-fold cross-validation across multiple training runs, which provides less biased assessment than the traditional test:train split [26]. Specifically, LOOCV involves a series of training runs that equals the number of events. The model sequentially leaves one event out, trains the model on the other events, and tests the newly trained model on the left-out event. This is repeated until all events are tested. The results of the predictions are then averaged. This was performed for the CST and SSI models separately.
DLM Predictions and Evaluation
Statistical analysis was performed using Python version 3.7.1 by a data scientist. For internal validation, an 80:20 train:validation split was used. The models were assessed for discernibility and compared by training and validation accuracy, as well as the validation AUC score, across five training runs [27].
Results
Cohort Description
The internal CST sample had 297 patients (97 underwent CST). The internal SSI sample had 362 patients (77 with an SSI). The external cohort had 75 patients. Of which, 48 patients underwent CST, and 13 patients developed an SSI.
Leave-One-Out Cross-Validation
To build the DLMs with the ResNet-18 Architecture, the patients were divided into cohorts CST and SSI as described. LOOCV revealed that both models showed good performance. The CST model had an overall classification accuracy of 75% of cases. SSI performed better with 94.65% accuracy across the dataset.
Pooled Multicenter Cross-Validation
The internal and external combined cohort had 297 patients in the CST model and 362 patients in the SSI model. The CST model consisted of 237 internal patients and 60 external patients, with 77 and 38 CSTs in each group, respectively. The SSI model consisted of 300 internal patients and 62 external patients, with 64 and 11 SSIs in each group, respectively (Table 1).
For internal validation, after an 80:20 train:test split, the CST pooled cohort had training accuracy of 91.26%, validation accuracy of 39.53%, and an AUC of 0.569 (Figure 1). the sensitivity was 41.94% and specificity of 67.77%. The SSI performed better with training accuracy of 97.92%, validation accuracy of 88.61%, AUC of 0.898 (Figure 2), sensitivity of 55.56%, and specificity of 95.65%.
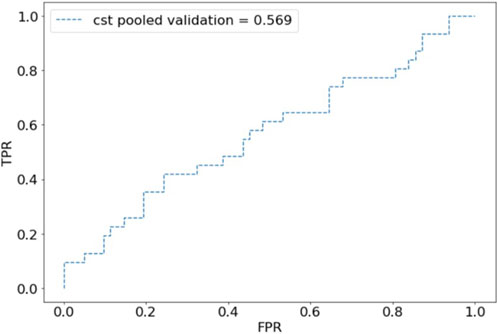
Figure 1. Receiver operating characteristic (ROC) plot for component separation technique (CST) predictions of pooled validation group.
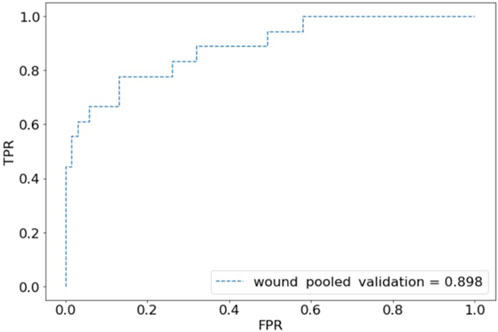
Figure 2. Receiver operating characteristic (ROC) plot for wound complications predictions of pooled validation group.
Discussion
This study describes the first known efforts to create and validate multicenter DLMs using AI to predict surgical complexity and postoperative outcomes. The results show proof of concept for multicenter development of image-based DLMs. While we have previously developed and demonstrated DLMs’ ability to predict intraoperative and postoperative outcomes, external validation has not been performed [5, 28]. A multicenter model was developed to evaluate whether pooled training and analysis would improve the models’ performance. While the CST model showed poor performance with a validation accuracy of 39.53% and an AUC of 0.568, the SSI model was more promising with a validation accuracy of 88.61% and an AUC of 0.879.
In general, external validation of predictive models is rarely described in the literature with only 5% of the approximately 85,000 prediction model publications on PubMed including some form of external validation [17, 29]. Specifically, many commonly used AWR risk stratification tools lack external validation [7]. To temper the recent excitement of using AI in surgical decision-making, Loftus et al recently called for more rigorous external validation, especially for AI prediction models [1]. This study was conducted to help address this evident gap in the literature.
Creating an externally validated DLM has many benefits, namely, its ability to become an advanced surgical risk calculator to provide personalized and informed patient counseling. There are currently several surgical risk calculators for AWR [7, 30]. The group at Carolinas Medical Center has previously published work aimed at predicting outcomes and patient centered care through the Carolinas Equation for Determining Associated Risk (CeDAR) application, which identifies patients that are at risk of wound complications after AWR along with their predicted costs [7, 31]. Unlike DLMs, this app requires human input to estimate risk [31]. Our group has also used volumetric assessment of CT scans to estimate surgical risk [32, 33]. The limitation to this method is the time and labor involved, as well as the subjectivity in data collection. DLMs can improve a surgeon’s predictive ability and aid in surgical planning and patient counseling [1, 3]. The end goal of DLMs is not to replace a surgeon’s clinical judgment, but rather augment it [1, 2].
The CST model performed poorly. While achieving fascial closure is the goal in AWR, techniques to achieve this vary [21, 34, 35]. The decision to perform a CST is complex and subjective, and practices often differ from institution to institution as well as patient to patient [21, 34]. There is a difference in practice and patient population, between the institutions, which is evident in the frequency of CST in each cohort [21]. While the authors attempted to propensity match the internal and external groups, this further limited the sample size. Therefore, the decision was made to continue without propensity matching. As a result though, differences in patient factors, such as hernia size or BMI, could contribute to the differences in rate of component separation. Another potential contributor to the poor performance of the CST model is the inability to predict tissue compliance. Past medical history and imaging do not capture compliance, as it is a difficult component to measure, but we suspect this too played a role in the model’s performance.
Additionally, CST is a broad term that can be used for many specific procedures. While some surgeons may choose to do a posterior component separation, or Transversus Abdominus Release (TAR), others may choose an anterior approach. While both techniques have their advantages, individual patient differences may lead a surgeon to perform one technique over the other [21, 22, 34]. The surgeons of the internal cohort choose to perform an anterior or posterior CST based on defect size [21]. The surgeon of the external cohort also performs both anterior and posterior CST, but typically performs anterior CST for larger defects. Given the varied practice patterns, it is difficult to train a reliable and predictive model that will perform on external data [17, 29]. Even with pooled training and analysis the poor performance of the model is likely explained by the nuanced practice difference between AWR centers.
On the other hand, the SSI model was found to have excellent predictive ability. An explanation for this finding may be that patient factors such as obesity and predisposing comorbidities, rather than institutional differences in surgical practice, are more likely determinants of developing SSIs [8, 9, 20, 36, 37]. Factors such as the amount of subcutaneous adipose tissue, as a surrogate for BMI, are evident on the CT scans and may contribute to the model’s ability to predict outcomes [32, 33, 38–42]. Predicting and preventing SSIs is vital for successful AWR. SSIs have been shown to increase a patient’s risk of developing a hernia recurrence by three to five times [8, 43, 44]. Additionally, superficial wound complications increase a patient’s likelihood of a mesh infection, which is a feared complication of AWR, that will likely lead to further operations in the future [43, 45].
Not only are SSIs responsible for poor patient outcomes, but also for increased healthcare spending [8, 9, 11]. The cost of complications has been explored in prior work [9]. The difference in outpatient charges between patients with and without a complication is $6,200 ± 13,800 and $1,400 ± 7,900, respectively, with more than four more office visits [9]. Determining which patients are at an increased risk for postoperative wound complications allows surgeons to intervene and decrease the risk of complications. Optimization of patients’ outcomes could either be preoperative, in the form of preoptimization, intraoperative, or postoperative. Intraoperatively, maintaining strict sterility, judicious handling of the skin and soft tissues, as well as electing to use closing protocols can decrease the rate of SSI [20, 37, 46]. Postoperative options include the decision to perform a delayed primary closure (DPC) or apply a closed incision negative pressure wound therapy vacuum [19, 47, 48].
This study is not without limitations. A pooled multicenter analysis was performed, yet again, the CST model did not perform well. An explanation for the initial model’s poor performance is the skewed nature of the datasets. The external cohort was limited with 75 patients. The external cohort also had different proportions of CST procedures performed. This is due to different AWR practice models. The internal group often uses botulinum toxin injections as a means to prevent the need for CST. This may differ from the practice algorithm of the external validation group or even other practices that may use techniques such as progressive pneumoperitoneum. This inherently is a limitation with comparing different medical centers and practices and may make our study less generalizable. Further, models developed with ResNet-18 are known to perform better with skewed data sets, like this study. Knowing the skewed nature of the datasets allows the model to be scaled appropriately. While training and validating a model based on pooled data seems promising, it is likely that a multi-institution model would need to be developed to account for the vast difference in practice patterns in CST among AWR surgeons.
This study is the first of its kind demonstrating techniques to externally validate a predictive surgical model. We demonstrated that while CST is challenging to predict, the SSI model performed well in a multicenter setting. This study indicates that models can predict outcomes where patient factors are readily evident in the data but are limited where there is subjectivity in surgical management. Future directions for study should look to train AI models on large multicenter databases to account for variations in surgical practice.
Data Availability Statement
The raw data supporting the conclusions of this article will be made available by the authors, without undue reservation.
Ethics Statement
The studies involving humans were approved by Carolinas Medical Center and Ohio State University Medical Center institutional review boards. The studies were conducted in accordance with the local legislation and institutional requirements. The participants provided their written informed consent to participate in this study.
Author Contributions
All authors participated in the design, interpretation of the studies, and analysis of the data; SK, HW, SA, and BM performed and oversaw data collection; BS, KM, and GS led the computer science and statistical analysis; WL, AH, BS, BH, and JJ wrote the manuscript and participated in review of the manuscript; BH and JJ oversaw the entire project. All authors contributed to the article and approved the submitted version.
Funding
The author(s) declare that financial support was received for the research and/or publication of this article.
Conflict of Interest
The authors declare that the research was conducted in the absence of any commercial or financial relationships that could be construed as a potential conflict of interest.
Generative AI Statement
The authors declare that no Generative AI was used in the creation of this manuscript.
Publisher’s Note
All claims expressed in this article are solely those of the authors and do not necessarily represent those of their affiliated organizations, or those of the publisher, the editors and the reviewers. Any product that may be evaluated in this article, or claim that may be made by its manufacturer, is not guaranteed or endorsed by the publisher.
References
1. Loftus, TJ, Tighe, PJ, Filiberto, AC, Efron, PA, Brakenridge, SC, Mohr, AM, et al. Artificial Intelligence and Surgical Decision-Making. JAMA Surg (2020) 155(2):148–58. doi:10.1001/jamasurg.2019.4917
2. Shortliffe, EH, and Sepúlveda, MJ. Clinical Decision Support in the Era of Artificial Intelligence. JAMA - J Am Med Assoc (2018) 320(21):2199–200. doi:10.1001/jama.2018.17163
3. Nundy, S, Montgomery, T, and Wachter, RM. Promoting Trust between Patients and Physicians in the Era of Artificial Intelligence. JAMA - J Am Med Assoc (2019) 322(6):497–8. doi:10.1001/jama.2018.20563
4. Matheny, ME, Whicher, D, and Thadaney Israni, S. Artificial Intelligence in Health Care: A Report from the National Academy of Medicine. JAMA - J Am Med Assoc (2020) 323(6):509–10. doi:10.1001/jama.2019.21579
5. Elhage, SA, Deerenberg, EB, Ayuso, SA, Murphy, KJ, Shao, JM, Kercher, KW, et al. Development and Validation of Image-Based Deep Learning Models to Predict Surgical Complexity and Complications in Abdominal Wall Reconstruction. JAMA Surg (2021) 156(10):933–40. doi:10.1001/jamasurg.2021.3012
6. Kanters, AE, Krpata, DM, Blatnik, JA, Novitsky, YM, and Rosen, MJ. Modified Hernia Grading Scale to Stratify Surgical Site Occurrence after Open Ventral Hernia Repairs. J Am Coll Surg (2012) 215(6):787–93. doi:10.1016/j.jamcollsurg.2012.08.012
7. Bernardi, K, Adrales, GL, Hope, WW, Keith, J, Kuhlens, H, Martindale, RG, et al. Abdominal Wall Reconstruction Risk Stratification Tools: A Systematic Review of the Literature. Plast Reconstr Surg (2018) 142(3S):9S–20S. doi:10.1097/PRS.0000000000004833
8. Holihan, JL, Alawadi, Z, Martindale, RG, Roth, JS, Wray, CJ, Ko, TC, et al. Adverse Events after Ventral Hernia Repair: The Vicious Cycle of Complications Abstract Presented at the Abdominal Wall Reconstruction Conference, Washington, DC, June 2014. J Am Coll Surg (2015) 221(2):478–85. doi:10.1016/j.jamcollsurg.2015.04.026
9. Cox, TC, Blair, LJ, Huntington, CR, Colavita, PD, Prasad, T, Lincourt, AE, et al. The Cost of Preventable Comorbidities on Wound Complications in Open Ventral Hernia Repair. J Surg Res (2016) 206(1):214–22. doi:10.1016/j.jss.2016.08.009
10. Schlosser, KA, Renshaw, SM, Tamer, RM, Strassels, SA, and Poulose, BK. Ventral Hernia Repair: An Increasing Burden Affecting Abdominal Core Health. Hernia (2023) 27(2):415–21. doi:10.1007/s10029-022-02707-6
11. Poulose, BK, Shelton, J, Phillips, S, Moore, D, Nealon, W, Penson, D, et al. Epidemiology and Cost of Ventral Hernia Repair: Making the Case for Hernia Research. Hernia (2012) 16(2):179–83. doi:10.1007/s10029-011-0879-9
12. Decker, MR, Dodgion, CM, Kwok, AC, Hu, YY, Havlena, JA, Jiang, W, et al. Specialization and the Current Practices of General Surgeons. J Am Coll Surg (2014) 218(1):8–15. doi:10.1016/j.jamcollsurg.2013.08.016
13. Shulkin, JM, Mellia, JA, Patel, V, Naga, HI, Morris, MP, Christopher, A, et al. Characterizing Hernia Centers in the United States: What Defines a Hernia Center? Hernia (2022) 26(1):251–7. doi:10.1007/s10029-021-02411-x
14. Williams, KB, Belyansky, I, Dacey, KT, Yurko, Y, Augenstein, VA, Lincourt, AE, et al. Impact of the Establishment of a Specialty Hernia Referral Center. Surg Innov (2014) 21(6):572–9. doi:10.1177/1553350614528579
15. Seaman, AP, Schlosser, KA, Eiferman, D, Narula, V, Poulose, BK, and Janis, JE. Building a Center for Abdominal Core Health: The Importance of a Holistic Multidisciplinary Approach. J Gastrointest Surg (2022) 26(3):693–701. doi:10.1007/s11605-021-05241-5
16. Köckerling, F, Berger, D, and Jost, JO. What Is a Certified Hernia Center? The Example of the German Hernia Society and German Society of General and Visceral Surgery. Front Surg (2014) 1(July):26–4. doi:10.3389/fsurg.2014.00026
17. Ramspek, CL, Jager, KJ, Dekker, FW, Zoccali, C, and Van Diepen, M. External Validation of Prognostic Models: What, Why, How, When and Where? Clin Kidney J (2021) 14(1):49–58. doi:10.1093/ckj/sfaa188
18. Collins, GS, Reitsma, JB, Altman, DG, and Moons, KGM. Transparent Reporting of a Multivariable Prediction Model for Individual Prognosis or Diagnosis (TRIPOD): The TRIPOD Statement. BMJ (Online) (2015) 350(January):g7594–9. doi:10.1136/bmj.g7594
19. Janis, JE, and Khansa, I. Evidence-Based Abdominal Wall Reconstruction. Plast Reconstr Surg (2015) 136(6):1312–23. doi:10.1097/PRS.0000000000001831
20. Joslyn, NA, Esmonde, NO, Martindale, RG, Hansen, J, Khansa, I, and Janis, JE. Evidence-based Strategies for the Prehabilitation of the Abdominal Wall Reconstruction Patient. Plast Reconstr Surg (2018) 142(3S):21S-29S–29S. doi:10.1097/PRS.0000000000004835
21. Maloney, SR, Schlosser, KA, Prasad, T, Kasten, KR, Gersin, KS, Colavita, PD, et al. Twelve Years of Component Separation Technique in Abdominal Wall Reconstruction. Surgery (United States) (2019) 166(4):435–44. doi:10.1016/j.surg.2019.05.043
22. Khansa, I, and Janis, JE. The 4 Principles of Complex Abdominal Wall Reconstruction. Plast Reconstr Surg Glob Open (2019) 7(12):E2549. doi:10.1097/GOX.0000000000002549
23. Ban, KA, Minei, JP, Laronga, C, Harbrecht, BG, Jensen, EH, Fry, DE, et al. American College of Surgeons and Surgical Infection Society: Surgical Site Infection Guidelines, 2016 Update. J Am Coll Surgeons (2017) 224:59–74. doi:10.1016/j.jamcollsurg.2016.10.029
24. Paszke, A, Gross, S, Massa, F, Lerer, A, Bradbury, J, Chanan, G, et al. PyTorch: An Imperative Style, High-Performance Deep Learning Library. Adv Neural Inf Process Syst (2019) 32(NeurIPS).
25. Géron, A. Hands-on Machine Learning with Scikit-Learn, Keras, and TensorFlow: Concepts, Tools, and Techniques to Build Intelligent Systems. 2nd ed. Sebastopol, CA O’Reilly Media, Inc. (2019).
26. Meijer, RJ, and Goeman, JJ. Efficient Approximate K-fold and Leave-One-Out Cross-Validation for Ridge Regression. Biometrical J (2013) 55(2):141–55. doi:10.1002/bimj.201200088
27. Carter, JV, Pan, J, Rai, SN, and Galandiuk, S. ROC-Ing along: Evaluation and Interpretation of Receiver Operating Characteristic Curves. Surgery (United States) (2016) 159(6):1638–45. doi:10.1016/j.surg.2015.12.029
28. Ayuso, SA, Elhage, SA, Zhang, Y, Aladegbami, BG, Gersin, KS, Fischer, JP, et al. Predicting Rare Outcomes in Abdominal Wall Reconstruction Using Image-Based Deep Learning Models. Surgery (United States) (2023) 173(3):748–55. doi:10.1016/j.surg.2022.06.048
29. Siontis, GCM, Tzoulaki, I, Castaldi, PJ, and Ioannidis, JPA. External Validation of New Risk Prediction Models Is Infrequent and Reveals Worse Prognostic Discrimination. J Clin Epidemiol (2015) 68(1):25–34. doi:10.1016/j.jclinepi.2014.09.007
30. Talpai, T, Râmboiu, DS, Pîrvu, CA, Pantea, S, Șelaru, M, Cârțu, D, et al. A Comparison of Open Ventral Hernia Repair Risk Stratification Systems: A Call for Consensus. J Clin Med (2024) 13(22):6692. doi:10.3390/jcm13226692
31. Augenstein, VA, Colavita, PD, Wormer, BA, Walters, AL, Bradley, JF, Lincourt, AE, et al. CeDAR: Carolinas Equation for Determining Associated Risks. J Am Coll Surg (2015) 221(4):S65–6. doi:10.1016/j.jamcollsurg.2015.07.145
32. Schlosser, KA, Maloney, SR, Prasad, T, Colavita, PD, Augenstein, VA, and Heniford, BT. Three-dimensional Hernia Analysis: The Impact of Size on Surgical Outcomes. Surg Endosc (2020) 34(4):1795–801. doi:10.1007/s00464-019-06931-7
33. Schlosser, KA, Maloney, SR, Prasad, T, Colavita, PD, Augenstein, VA, and Heniford, BT. Too Big to Breathe: Predictors of Respiratory Failure and Insufficiency after Open Ventral Hernia Repair. Surg Endosc (2020) 34(9):4131–9. doi:10.1007/s00464-019-07181-3
34. Ayuso, SA, Colavita, PD, Augenstein, VA, Aladegbami, BG, Nayak, RB, Davis, BR, et al. Nationwide Increase in Component Separation without Concomitant Rise in Readmissions: A Nationwide Readmissions Database Analysis. Surgery (United States) (2022) 171(3):799–805. doi:10.1016/j.surg.2021.09.012
35. Booth, JH, Garvey, PB, Baumann, DP, Selber, JC, Nguyen, AT, Clemens, MW, et al. Primary Fascial Closure with Mesh Reinforcement Is Superior to Bridged Mesh Repair for Abdominal Wall Reconstruction. J Am Coll Surg (2013) 217(6):999–1009. doi:10.1016/j.jamcollsurg.2013.08.015
36. Bosanquet, DC, Ansell, J, Abdelrahman, T, Cornish, J, Harries, R, Stimpson, A, et al. Systematic Review and Meta-Regression of Factors Affecting Midline Incisional Hernia Rates: Analysis of 14 618 Patients. PLoS One (2015) 10(9):e0138745–19. doi:10.1371/journal.pone.0138745
37. Khansa, I, and Janis, JE. Management of Skin and Subcutaneous Tissue in Complex Open Abdominal Wall Reconstruction. Hernia (2018) 22(2):293–301. doi:10.1007/s10029-017-1662-3
38. Santhanam, P, Nath, T, Peng, C, Bai, H, Zhang, H, Ahima, RS, et al. Artificial Intelligence and Body Composition. Diabetes Metab Syndr Clin Res Rev (2023) 17(3):102732. doi:10.1016/j.dsx.2023.102732
39. Lee, YS, Hong, N, Witanto, JN, Choi, YR, Park, J, Decazes, P, et al. Deep Neural Network for Automatic Volumetric Segmentation of Whole-Body CT Images for Body Composition Assessment. Clin Nutr (2021) 40(8):5038–46. doi:10.1016/j.clnu.2021.06.025
40. Kim, YJ, Lee, SH, Kim, TY, Park, JY, Choi, SH, and Kim, KG. Body Fat Assessment Method Using Ct Images with Separation Mask Algorithm. J Digit Imaging (2013) 26(2):155–62. doi:10.1007/s10278-012-9488-0
41. Yoo, HJ, Kim, YJ, Hong, H, Hong, SH, Chae, HD, and Choi, JY. Deep Learning–Based Fully Automated Body Composition Analysis of Thigh CT: Comparison with DXA Measurement. Eur Radiol (2022) 32(11):7601–11. doi:10.1007/s00330-022-08770-y
42. Mai, DVC, Drami, I, Pring, ET, Gould, LE, Lung, P, Popuri, K, et al. A Systematic Review of Automated Segmentation of 3D Computed-Tomography Scans for Volumetric Body Composition Analysis. J Cachexia Sarcopenia Muscle (2023) 14(May):1973–86. doi:10.1002/jcsm.13310
43. Cobb, WS, Warren, JA, Ewing, JA, Burnikel, A, Merchant, M, and Carbonell, AM. Open Retromuscular Mesh Repair of Complex Incisional Hernia: Predictors of Wound Events and Recurrence. J Am Coll Surg (2015) 220(4):606–13. doi:10.1016/j.jamcollsurg.2014.12.055
44. Katzen, MM, Kercher, KW, Sacco, JM, Ku, D, Scarola, GT, Davis, BR, et al. Open Preperitoneal Ventral Hernia Repair: Prospective Observational Study of Quality Improvement Outcomes over 18 Years and 1,842 Patients. Surgery (United States) (2023) 173(3):739–47. doi:10.1016/j.surg.2022.07.042
45. Kao, AM, Arnold, MR, Augenstein, VA, and Heniford, BT. Prevention and Treatment Strategies for Mesh Infection in Abdominal Wall Reconstruction. Plast Reconstr Surg (2018) 142(3S):149S-155S–155S. doi:10.1097/PRS.0000000000004871
46. Lorenz, WR, Polcz, ME, Holland, AM, Ricker, AB, Scarola, GT, Kercher, KW, et al. The Impact of a Closing Protocol (CP) on Wound Morbidity in Abdominal Wall Reconstruction (AWR) with Mesh. Surg Endosc. (2025) 39(2):1283–1289. doi:10.1007/s00464-024-11420-7
47. Ayuso, SA, Elhage, SA, Okorji, LM, Kercher, KW, Colavita, PD, Heniford, BT, et al. Closed-Incision Negative Pressure Therapy Decreases Wound Morbidity in Open Abdominal Wall Reconstruction with Concomitant Panniculectomy. Ann Plast Surg (2022) 88(4):429–33. doi:10.1097/SAP.0000000000002966
Keywords: artificial intelligence, ventral hernia repair, quality improvement, prediction model, component separation, deep learning model
Citation: Lorenz WR, Holland AM, Sarac BA, Kerr SW, Wilson HH, Ayuso SA, Murphy K, Scarola GT, Mead BS, Heniford BT and Janis JE (2025) Development of Multicenter Deep Learning Models for Predicting Surgical Complexity and Surgical Site Infection in Abdominal Wall Reconstruction, a Pilot Study. J. Abdom. Wall Surg. 4:14371. doi: 10.3389/jaws.2025.14371
Received: 20 January 2025; Accepted: 02 April 2025;
Published: 14 April 2025.
Copyright © 2025 Lorenz, Holland, Sarac, Kerr, Wilson, Ayuso, Murphy, Scarola, Mead, Heniford and Janis. This is an open-access article distributed under the terms of the Creative Commons Attribution License (CC BY). The use, distribution or reproduction in other forums is permitted, provided the original author(s) and the copyright owner(s) are credited and that the original publication in this journal is cited, in accordance with accepted academic practice. No use, distribution or reproduction is permitted which does not comply with these terms.
*Correspondence: Jeffrey E. Janis, amVmZnJleS5qYW5pc0Bvc3VtYy5lZHU=