- 1Faculty of Informatics, Eötvös Loránd University, Budapest, Hungary
- 2University Research and Innovation Center (EKIK)/John von Neumann Faculty of Informatics (NIK), Óbuda University, Budapest, Hungary
- 3Department of Statistics, Corvinus University of Budapest, Budapest, Hungary
- 4School of Computing, Queens University, Kingston, ON, Canada
Context: In recent years, the focus on sustainable meat production in large-scale pig farming has increased. An essential element is acquiring and evaluating digital data on ecological, outdoor pig housing environments and individuals.
Objective: Some of the precision farming Internet of Things (IoT) tools that can be used in pasture-based, extensive pig production include those that have been proven in pasture-based ruminants and have been used in confined pig production for many years. These include Radio Frequency IDentification (RFID) technology, in which passive RFID tags attached to pigs’ ears capture signals from a reader equipped with an antenna. The reader can also be used to collect weather parameters.
Methods: In our on-farm research, which lasted 7 months and covered three seasons, we used RFID technology to investigate the effects of ambient temperature, humidity, air pressure, and hour of day on the appearance of breeding sows kept on pasture at the wallowing site.
Results and conclusion: We found that all three weather parameters and hour-of-day had a significant effect (p < 0.001) on the appearance of sows at the wallow. We calculated the THI (Temperature Humidity Index) and found days in summer and early autumn when there was a risk of heat stress. This is essential information for the pig farmer because heat stress has a strong negative effect on sow fertility. Our on-farm study found that RFID technology is suitable for detecting the presence of pigs in the pasture. However, the limitations of the technology, both in terms of the housing environment and the species specificity of the pigs, need to be taken into account.
Significance: In pasture-based pig production systems, the meat production process is performed under extensive conditions of natural animal-environment interaction. The negative impact on the natural environment is much lower, but animal welfare is higher than in intensive pig farming. It is also a profit-oriented activity, where the production of traceable and reliable animal products is a social and economic requirement. Collecting information from trusted sources using precision livestock farming (PLF) techniques is also feasible in this type of farming, providing significant added value for pig farmers and consumers.
Introduction
In the context of livestock farming, sustainability implies models that provide the farmer with a reasonable and stable income without significant negative side effects on the environment and that is acceptable to society by ensuring both animal and farmer welfare (ten Napel et al., 2011). The pig industry is characterized overwhelmingly by closed, intensive, and large-scale housing systems worldwide that can supply large meat processing facilities (Mason et al., 2021; Takacs et al., 2023). This highly human-controlled farming system allows the collection of a large amount of data describing the farming environment and monitoring the pigs individually (Banhazi and Black, 2009) by sensor, camera, and microphone systems that belong to IoT (Internet of Things) devices placed in the housing area and on the animals (Tzanidakis et al., 2021). The IoT is a network of interconnected devices that communicate, sense, and interact with internal and external environments via embedded technology (Lee and Lee, 2015). They are completed by the data analysis using machine learning and traditional statistical methods, which aim to achieve environmental and economic sustainability. Bercksman (Berckmans, 2014) summarised the critical features of Precision Livestock Farming (PLF) technologies, which focus on animal health and welfare. One of the less studied areas of PLF applications in pig farming is pasture-based pig management systems, where pigs are on pasture all year round or at specific periods of the year, both during breeding and fattening periods (Edwards, 2005). In this housing system, pigs have more opportunities to express their species-specific behaviors, improving animal welfare and allowing for sustainable pig production. This ecological housing fits into the five freedoms framework defined by the Farm Animal Welfare Council in 1979 (Anonymous, 1993) and also falls in line with the U.N. Sustainability Development Goals (Mai et al., 2022). In order to achieve sustainable management in these extensive pig production systems, the housing environment and continuous monitoring of the animals are important factors. However, heat stress can also be a problem in free-range conditions, especially in the summer.
In contrast to confined housing, outdoor pigs are kept in larger territory, close to nature, where they have more opportunities to protect themselves from weather extremes. One behavioral solution is wallowing, when pigs cover their bodies with mud or lie in the water to reduce their exposure to excessive heat and sunlight (Bracke, 2011). Under all conditions, the pig farmer has an outstanding need to get up-to-date information on the health of the pigs and the risk of heat stress. Pigs, unlike ruminants, use pasture primarily as a living space rather than a feed source. Their species-specific behaviors (rooting and wallowing) mean they heavily use the grazing land. At the same time, the premium meat quality of the free-range pig breeds (Mangalica, Iberian pig) is of gastronomic value (Horrillo et al., 2023). However, due to the species-specificity of pigs and the extensive keeping system, digital and IoT data collection devices are limited. Most of the digital tools provided for ruminants on pasture are not available for the pigs, although the demand for this technology from organic pig farmers is growing. One possible solution is Radio Frequency Identification (RFID) technology, which has been used for many years in confined pig farming (mainly to monitor eating and drinking habits and to sort pigs according to specific criteria (Reiners et al., 2009; Voulodimos et al., 2010) RFID technology is also used to monitor ruminants on pasture (Munak, 2006), and can therefore be adapted to some extent to outdoor pig farming.
The RFID technology is a powerful tool for tracking the location of objects in real-time. Identification is achieved using radio frequency waves, and the information is stored on a data carrier (electronic memory chip). RFID exists in a full range of systems and components which operate on the same technological principle (Umble et al., 2003). The reader transmits information in two directions between the medium and the receiver (Hou and Ma, 2010). The tag comprises a small chip, an antenna, and a memory. Some types of RFID tags allow repeated recording of data. Tags are attached to observed objects, either on the object’s surface or outside the object, for identification purposes (Drazic et al., 2010). This technology belongs to the group of automatic identification technologies. RFID technology works by contactless transmission of information from tags. The advantages of RFID technology include providing information over long distances (several meters) and ease and affordability of the way to identify, track, and monitor livestock, thus improving the traceability of animals along the supply chain and reading rates of more than 100 tags per second. The adoption of RFID technology in practical farm management has allowed the development of asset-tracking software, where daily records on individuals (e.g., medical treatments, growth values, pedigree, reproductive performance) are automatically collected and stored (Ruiz-Garcia and Lunadei, 2011). RFID readers are also capable of collecting climatic data. In large-scale pig farming, passive RFID tags are mainly placed in ear tags (Maselyne et al., 2016). The signals transmitted by the antenna are collected by the readers and transmitted using a 2G/3G/4G/5G network to a data storage server, where the data is analyzed. Information of interest to pig farmers can be displayed graphically (Alexy et al., 2019). RFID technology has several limitations in outdoor circumstances. One of the disadvantages is that the presence of animals outside the operational range of the readers cannot be recorded, extreme weather conditions affect the continuous transmission of data, tags placed in pigs’ ears can be lost or torn out of each other’s ears during social interactions (playing or fighting). These factors and events can make the output data of an RFID system unreliable.
In our seven-month-long study, we tested the applicability of passive RFID technology in free-range pig housing conditions, collecting data on sows’ appearance and weather parameters at the wallow.
Material and methods
The investigation was conducted on sows housed in an outdoor, pasture-based system on a private farm in Hungary between June 2020 and January 2021. Data collection devices were installed at the pilot site in June 2020, and data collection started in July 2020 (Alexy and Horvath, 2022). The free-range pig farm is primarily used for the reproduction of gilts and fattening pigs. It complies with the current E.U. [98/58/E.C.] legislation on the keeping of farm animals, animal welfare, and environmental protection, which is continuously monitored by the authorities (Hungarian State Treasury Agriculture and Rural Development Agency, National Food Chain Safety Office and Hungarian National Association of Mangalica Breeders).
Animals
The Mangalica is a native Hungarian pig race that was the most typical breed locally until the middle of the last century. It is a fat-type, curly-haired swine with relatively low reproductive capacity but strong motherliness and very good adaptability to extensive housing conditions (Egerszegi et al., 2003). During the research, RFID data was collected on twenty sows with an average age of 5 years (Figure 1).
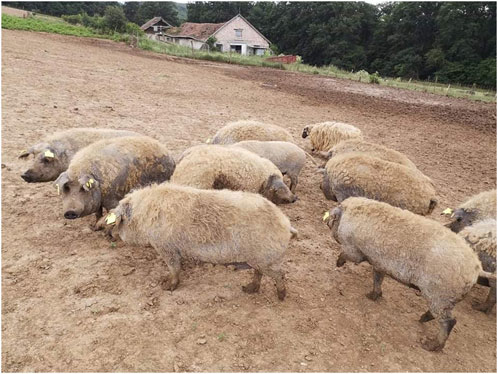
Figure 1. Mangalica breeding sows with passive RFID ear tags on pasture at the site of the experiment.
The sows farrow once a year, and the boars are with the sows all year round, except in September and October (to avoid winter farrowing). Therefore, the timing of first and subsequent insemination is challenging to determine. When the expected day of farrowing approaches, the farmer shepherds the sows to the enclosed farrowing building next to the pasture, where they farrow in individual boxes with concrete floors covered with straw and return to the pasture with their piglets two to 3 weeks after farrowing. The piglets are separated from the sows when they are 8–10 weeks old. Then, the sows are replaced in the paddock for regular keeping; the piglets go to the pasture section for fattening. At the beginning of our study, the sows were just after farrowing and were kept on the pasture for the entire experiment period. Data acquisition occurred from July 2020 to January 2021, so data on weather and sows’ presence on the pasture were collected for three seasons (summer, autumn, and winter).
Housing
In one group, the sows were kept on approximately 4 ha of fenced land, about 270 × 150 m, all year round. The layout of the land is shown in Figure 2.
The site had a 2.5 × 10 m wooden resting building and a drinking trough filled with a water tank. The four farrowing huts’ sizes were 2.5 × 2.5 m each and were located in the upper part of the pasture, close to the tree line. About 20% of the area was covered with shrubs and lower trees. In the central part of the area, there was a Jimsonweed (Datura stramonium L.) plant, which pigs used as a resting and walking place. The rest of the area was not covered with vegetation, leaving only the greenery that was not eaten or destroyed by the sows. Near the fence, from inside the area, a wallow was created by the pigs themselves, whose shape and depth varied according to their activity. Its largest size was in the autumn, with a 5 m length, 2.5 m width, and 45 cm depth. The farmer occasionally filled water into this pit, and rainwater flowed there too. When the wallow was full of water, the sows could sink entirely to the muddy water if they lay laterally, and a maximum of five sows could lay into the water at once. The wallow was far from the watering trough, the feeding area, and the resting place. The pasture had a slope of 5%. The fenced pasture had a free place for pigs to use; the sows could use the wallowing area at any time. In the early morning, animals were fed once a day with no commercial feed ingredients (fruits, vegetables, corn), and they had ad libitum access to drinking water. Farm workers entered the pasture only when it was necessary, so there was no human presence most of the time.
Data acquisition about the presence of sows and weather parameters
Environmental recording
The Hungarian Meteorological Service (OMSZ) provided relevant data from the nearest meteorological stations: solar radiation, precipitation, and wind data daily and cloud coverage data monthly. The precipitation data were obtained from Nagymaros (47°46′18″N, 18°56′44″E, altitude above mean sea level: 106.0 m; distance from the experimental site: 3.7 km) the solar radiation data were collected in Püspökszilágy (47°29′52.8″N, 19°19′36″ E, altitude above mean sea level: 239.0 m; distance from the experimental site: 31 km). Wind speed values were obtained from Nagy-Hideg Hill (47°56′11″N, 18°55′18″E, altitude above mean sea level: 854.8 m; distance from the experimental site: 22 km). Cloud cover data were obtained from the Pestszentlőrinc meteorological station (47°29′52.8″N 19°02′23.7″E, altitude above mean sea level: 125 m; distance from the experimental site: 35 km). Four RFID readers fixed to the fences near the wallow recorded hourly temperature, humidity, and air pressure values, resulting in 5040 values for each of the three parameters (15,120 records in total).
In order to describe environmental effects on animals, several indices, including the Temperature-Humidity Index (THI), have been developed and are widely applied (Wegner et al., 2016). We calculated the THI for each day of the study to estimate the level of potential heat stress. The aggregation of ambient temperature and relative humidity causes it:
where ambient temperature is in degrees Celsius and relative humidity is in % (Mader et al., 2006).
For descriptive purposes, the mean, maximum, minimum, and standard deviation of temperature, relative humidity (R.H.), and THI were calculated for all study days for all three seasons of the behavioral observation period (Table 1).
Sows’ presence in the wallowing area
Their passive RFID tags detected sows’ presence at the wallowing site at the pasture fence (Figure 2) 24 h/day. They recorded the arrival and leave of 20 sows, each with one RFID tag (diameter 30 mm, circle shape, frequency 850 MHz UHF). The detection distance of the RFID readers with antennas (type: SR450, square-shaped, 45 cm × 45 cm, Inpinj R2000 chip, model: CF-MU804, HDMI, USB 2.0, Ethernet, optional WLAN or 3G, Ethernet network connection, 10Mbit, Four 50 Ω/RPTNC connectors supporting four monostatic antennas, Read Rate: up to 750 tags/second using high-performance settings) was 4.5 m. The detection distance was tested in the field before the study’s launch. The readers were set up so the entire wallowing area could be observed. The size of the wallow varied during the experimental period, depending on the weather conditions. In total, the RFID readers recorded the presence of sows 9070 times during the study.
At the start of the study, after testing the RFID devices, plastic ear tags containing the passive RFID tags were attached to both ears of each 20 Mangalica sows. Data on sows’ presence at the wallow were collected for the entire study period, from 1 July 2020 to 26 January 2021. We added the weather parameters collected directly by the RFID readers to the data series on sows’ presence, The data collection period was divided into 10-min time intervals. A sow’s appearance was considered for the analysis if it spent at least this interval at the wallowing site. In addition, we cleaned the dataset of sows that appeared less than 200 times at the experimental site. Half the initial number of sows, ten sows, participated during the entire study. Thus, our dataset prepared for analysis recorded ten sows’ appearances representing at least 200 occasions over the entire study period.
Statistical analysis
As the presence of a pig at a certain time was a binary variable (yes/no indicator), logistic regression was used for its multivariable modeling, with temperature, air pressure, humidity, and hour-of-the-day as the covariates. All covariates were continuous variables, and they were expanded with splines to allow for a potential non-linear effect (hour-of-the-day was expanded with cyclic spline) (Harrell and Frank, 2015). Note that this model is static and is not corrected for autocorrelation.
To present the regression results, we selected 1 day from each of the three seasons (5 August, 22 October, and 10 January) when the seasonal weather could be considered typical. We fixed the values of independent variables except the hour of the day. All independent variables were fixed at the average values for the study period on the selected 3 days. We also presented the likewise partial effect of a few critical meteorological variables.
Calculations were carried out under the R statistical environment version 4.2.0 (R Core Team, 2022), using the mgcv package version 1.8–40 (Wood, 2017) and gratia version 0.7.3 (Simpson, 2022). The full analysis script is available at https://github.com/tamas-ferenci/MangalicaStatistics.
Results
Environmental conditions
The environmental conditions during the study are summarized in Table 1. The highest THI value (71.7 ± 8.6) was measured in the summer period, while in the autumn, the THI’s average value was 53.8 ± 12.7. However, the most considerable variation in THI values was observed in this season because the weather in early autumn was similar to the conditions in the summer season. At the same time, the temperature in November was much lower. During the winter period, we had the lowest THI values (38.2 ± 6.66) due to the low temperatures. The THI for the whole 7-month study was 54.7 ± 16.2.
Looking at the temperature data, the average was 22.4°C ± 7.3°C during the summer, but there were sweltering days with temperatures reaching 40°C. However, on cooler summer days, the temperature was 6°C in the morning hours. Four hot days were recorded during this season, with maximum temperatures between 37°C and 40°C (in mid-July and August). These days, the humidity was extremely low (between 0.56% and 12.6%). The lowest temperatures were recorded at night and dawn (between 3 and 6 a.m.). The average temperature during the autumn was 10.7°C ± 7.9°C. The measured daytime temperatures in early autumn were 34°C–35°C on 5 days. This was the so-called “Indian summer,” which is typical of the Hungarian climate in early/mid-September. These values were coupled with higher humidity values (between 8.5% and 11.8%) compared to the relative humidity of hot summer days. However, temperatures can drop drastically in a few days during this season. From mid-October to the end of November, there were 16 days when the daily average temperature fell below 0°C. In October, this was observed during the night, at dawn, and in November, even in the early evening. The average temperature during winter was 1.55°C ± 3.6°C, which was in line with the season. The daytime maximum and minimum temperatures were the lowest during this season (15.1 and −15.1°C, respectively). The lowest temperatures were recorded in January (between −15 and −12°C) at night and dawn.
The average humidity over the study period was 66.8% ± 25.7%. During the summer, the average humidity was 45.8% ± 24.3%, with the lowest recorded value of 0.5%. Humidity values between 0.5% and 5% belonged to temperatures above 35°C, with little cloud cover on these days (5, 10, 15, and 30 July and 1, 12, and 13 August, the values were 0.0, 0.5, 2.2, 2.2, 0.0, 1.4, 1.8%, respectively). However, on 3 days (11, 15, and 17 August), the air humidity reached 95%–99.7%. The cloud cover value on these days was significant (4.8, 4.5, and 4.5), and the amount of precipitation was also considerable (2.4, 19.2, and 16.9 mm). A summer thunderstorm caused the higher air humidity. During the autumn, the decreasing daily mean temperature was accompanied by increasing humidity values (71.4% ± 23.66), with a maximum value of 99.9% and a minimum value of 1.25%, similar to the summer period. On 34 days, the humidity values ranged between 95% and 99.9%, and the temperature was between −1.7°C and 12.1°C. There was 1 day with higher precipitation (13 October, 47.6 mm) when the daily temperature dropped from 6.1°C in the morning to 5.1°C at the end of the day. The warm weather of the autumn period gradually changed to early winter conditions, with humidity levels ranging from 95% to 99.9% on one-third of the autumn period (34 days out of ninety). The highest average humidity values (81.4% ± 13.9%) were recorded in winter, with a minimum value not falling below 30.9%. On twenty-five of the 60 days studied, the measured humidity was between 95% and 99.9%, with a gradual temperature decrease from 7.2°C to −4.4°C. On the coldest days, the temperature at night did not rise above −10°C (on 2 days, 17 and 18 January), with humidity ranging between 68.6% and 76.6%.
Patterns of the probability of sows’ appearance by the time of day and weather parameters
During the study period, we covered three seasons. Given that each season brought different weather conditions and day lengths, we examined the probability of sows’ appearance at wallowing sites on a selected day in each season, controlling for meteorological parameters (Figure 3). The graphs for each sow in Figure 3 show the effect of a given weather parameter on the appearance of the sows. The grey bar above and below the black line shows the confidence interval of the weather parameter’s effect on the sow’s appearance, within which the independent variable had an effect. We also examined the significance of the covariates in the logistic regression; Table 2 shows these for a few selected explanatory variables. The results for each independent variable were as follows.

Figure 3. Evolution of the daily activity curves for each sow at the wallow, effect of time-of-the-day, humidity, air pressure, and temperature.
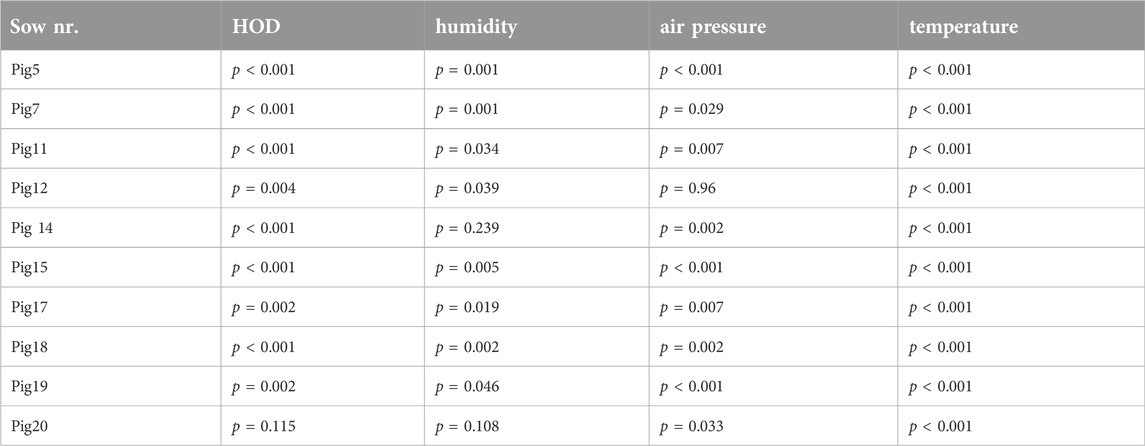
Table 2. The p-values of key explanatory variables in multivariable logistic regression (remaining covariates’ p-values are not shown in the table).
Analyzing the impact of hour-of-the-day, it can be concluded that the wallowing activity of each sow differed and that two or three daily activity peaks were observed. Two activity peaks were seen for sows 7, 11, 14, 15, 17, 18, 19, and 20; three were characteristic for sows 5 and 12. The first activity peak was observed in the early morning for all sows. It was related to the feed distribution time, around 6:30 a.m. daily. Afterward, the sows went to the wallow, where they spent approximately one hour. The second peak of their activity was observed in the afternoon (between 2 p.m. and 5 p.m.), except for sow no. 20, which returned to the observation area later than the others, rather in the evening. The effect of hour-of-day on the likelihood of appearing at the wallow showed a significant correlation for all sows, with no statistically significant values found for all except no. 20 (p = 0.115).
The first of the weather parameters to examine was the effect of air humidity on the probability of the appearance of individual sows at the wallowing site. The data clearly show that the probability of sows’ appearance at the study site decreases as air humidity increases. Analyzing the behavior individually, it can be concluded that there was a significant correlation between the probability of sows’ presence and the air humidity. A vital significance (p-value between 0.001 and 0.005) was observed for the probability of appearance of sows 5, 7, 15, and 18, with 5% significance values for the probability of appearance of sows 11, 12, 17, and 19. No significant effect was found between sows 14 and 20, nor was the air humidity value.
The following weather parameter was the air pressure, the effect of which was analyzed on the probability of sows’ appearance at the wallowing site. No significant effect was found between the probability of emergence of 12 sows and the air pressure values (p = 0.96). The effect for 7 and 20 sows and the air pressure was significant at a 5% significance level, while a p-value of 1% or below was found for seven sows. Referring to Figure 3, we found that air pressure values had less effect on the probability of sows appearing at the wallow.
The third weather parameter studied was temperature. We found a significant correlation between the effect of the temperature and the probability of sows being at the wallow for all sows (p < 0.001). Analyzing the graphs in Figure 3, we concluded that the probability of sows’ appearance at the wallowing site is reduced with increasing temperature. Although the relationship between sows’ appearance and temperature values was significant, the temperature had only minimal effect (for sows 7, 11, 12, and 20) on the probability of sows’ appearance at the wallowing site. Uniquely, the probability of the appearance of sow 14 increased with rising temperature. The temperature influenced the occurrence of five sows; they (sows 5, 15, 17, 18, and 19) visited the wallowing site most frequently between 0°C and 20°C.
Examining the effects of the four independent variables, we found that the effect of hour-of-day was observed for all sows studied. A trend in the effect of humidity on sow emergence can be observed for most sows: as humidity increases, sows’ appearance at the wallowing site decreases. No such trend in sow emergence is observed for air pressure or temperature.
Discussion
By analyzing the data, we determined whether the correlations and findings obtained would provide helpful information to the farmer regarding the group of free-range breeding sows. RFID technology allows the recording of animal appearance in the area monitored by the RFID reader; in this study, we did not assign any other data related to breeding and housing to the concerned sow. One of the objectives of this study was to confirm or refute the applicability of RFID technology in outdoor pig housing conditions and, by assigning weather parameters to the sows’ appearance dataset, to obtain information on whether a correlation can be established between the appearance of breeding sows at the wallowing site and the three studied weather parameters.
Our research confirmed that the risk of heat stress increases on hot days in summer and early autumn. It can be assessed by calculating the Temperature-Humidity Index, for which the required temperature and humidity values were recorded directly at the experimental site. We did not include the THI as an independent variable in our regression model since this indicator is calculated from the temperature and humidity values, and these two weather parameters were already included in the model as independent variables. A similar study was conducted by (Baert et al., 2022), who investigated the behavior of pregnant and lactating sows kept outdoors during the summer. Their experiment demonstrated that sows preferred to use the wallow in hot summer weather conditions (especially at high THI values). In our research for several days during the summer months, THI values ranged from 83.2 to 88.4, indicating a risk of heat stress. It could be hazardous for breeding animals because prolonged heat stress negatively affects their reproductive performance, which reduces the profitability of the pig farms (St.Pierre et al., 2003). Under free-range conditions, pigs can reduce their body temperature by seeking shade or wallowing. Wallowing is a species-specific behavior of pigs and plays a vital role in thermoregulation and defense against parasites and their social relationships. However, this behavior is not essential for subsistence but has a positive impact on the welfare of the pigs. Considering this aspect, we were curious to know at which time of day the sows visit this place. It was one of the reasons for placing the RFID readers around the wallowing area. The other parameters characterizing the current weather (solar radiation, cloud cover, precipitation, wind speed) were obtained from the meteorological stations nearest the research site. Given that the pig farm is located in the mountains and the meteorological stations are several kilometers away, these values could only be considered in our conclusions with some bias. Although they were included in the dataset model, this study did not investigate their impact.
In the data set analysis, we found that the most robust statistical relationship was between the presence of sows and the temperature (p < 0.001). However, the temperature did not affect the probability of being at the wallowing site for a subgroup of the sows. However, when examining the effect of hour-of-day, we found that the two peaks of activities reported in the literature were observed with these animals (Dailey and McGlone, 1997; Alexy, 2004). The first activity peak was likely due to the morning feed distribution, after which most sows visited the wallowing area. By analyzing the relationship of humidity values with sows’ attendance, it can be concluded that as humidity values increase, the probability of individual sows’ attendance at the wallow decreases. Examining the air pressure values, we found that although there was a significant relationship between the sow emergence and the air pressure values, this weather parameter was less influential.
Our research confirmed the literature sources on the limitations of RFID technology (Ruiz-Garcia and Lunadei, 2011). These include the limitation of the reading distance, which meant that we could only monitor sows for as long as the reader could detect the tag in their ears. Second, attention must be paid to replacing ear tags lost by animals. In our research, we placed ear tags in both ears of the sows to ensure that the animal could be identified when one was lost. In addition, stormy weather caused problems with data transmission twice, but the reader stored the data for 3 days, so there was no data loss.
Moreover, the RFID readers require electricity, which is not evident under free-range conditions. In our field research, this location was the wallowing area, where we supplied the readers with electricity most cost-effectively. One of the advantages of RFID technology is that it has worked reliably, with the readers withstanding the varying weather conditions for months (Morrison et al., 2001). Neither the ear tags nor the other elements of the RFID technology devices disturbed the sows in any noticeable way. Inserting the ear tags was a relatively straightforward process for the farmer, as it is required to identify breeding pigs uniquely. The RFID tags were the same size and material as conventional ear tags. Another possibility to be highlighted is to add to the RFID tag’s identification number additional husbandry and breeding data that the farmer considers essential, thus creating an even more complex dataset based on the unique identification of the animals and offering a helpful precision livestock technology.
Unlike cattle, the RFID tag used in pigs is primarily passive (Alexy and Haidegger, 2022). The reason for this is that the size and weight of the ear tag are determining factors in pigs. The pigs’ behavior is characterized by their daily social interactions, which can be either fighting or playing, where pigs can bite into each other’s ears with their teeth and tear out the ear tag. In free-range conditions, pigs are less frequently checked by their farmers, so individual identification, completed with digital data collection, is essential for monitoring the health of the pig herd.
Our study has several limitations that should be considered when conducting another similar study in the future. First, installing RFID technology in other parts of the pasture area used by pigs (resting area, watering area, feeding area) is worthwhile. On the other hand, installing cameras can provide more accurate information on the monitored sites. In this case, the cost of installing cameras and the infrastructure needed should be considered. Third, it is also worthwhile to associate breeding and husbandry data with the RFID identification of the sows.
In our future work, we aim to extend the study to monitor more animals in various settings. To this end, a combination of RFID and CCD-based monitoring technologies can be developed (Szabo and Alexy, 2022).
Conclusion
Providing animal welfare and increasing consumer expectations are critical elements of sustainable pig farming. Despite its limitations, RFID technology represents a step forward in using precision methods compared to current practices under sustainable outdoor pig farming circumstances.
With this research, we have achieved our goal of demonstrating that RFID technology can reliably collect individual data on free-range pigs, given its limitations, and that the data can be transmitted to the data analysis server via a 4G network without any problems. RFID technology should be considered one of the digital data collection methods for sustainable pig farming.
Data availability statement
The datasets presented in this study can be found in online repositories. The names of the repository/repositories and accession number(s) can be found below: https://github.com/tamas-ferenci/MangalicaStatistics.
Ethics statement
The manuscript presents research on animals that do not require ethical approval for their study.
Author contributions
The authors confirm contribution to the paper as follows: study conception and design: MA. Data collection: MA and RP. Analysis and interpretation of results: MA, RP, and TF. Draft manuscript preparation: MA, TF, and TH. All authors reviewed the results and approved the final version of the manuscript.
Funding
The author(s) declare that financial support was received for the research, authorship, and/or publication of this article. MA work is partially supported by the “Application Domain Specific Highly Reliable I.T. Solutions” project, which has been implemented with support provided from the National Research, Development, and Innovation Fund of Hungary, financed under the Thematic Excellence Programme TKP2020-NKA-06 (National Challenges Subprogramme) funding scheme.
Acknowledgments
We especially thank Róbert Lacko, a Hungarian private Mangalica farmer who can carry out the experiments on his farm and provided us with his pig herd. We also thank Simplexion Informatikai Kft for their cooperation, which provided the tools for digital data collection and continuous consultation assistance. We thank the president of the National Association of Mangalica Breeders for professional support.
Conflict of interest
The authors declare that the research was conducted in the absence of any commercial or financial relationships that could be construed as a potential conflict of interest.
References
Alexy, M. (2004). Results of pasture-based gilts and breeding sow housing system. PhD-thesis. University of Debrecen, Centre of Agricultural Sciences.
Alexy, M., and Haidegger, T. (2022). “Precision Solutions in Livestock Farming–feasibility and applicability of digital data collection,” in 2022 IEEE 10th jubilee international conference on computational cybernetics and cyber-medical systems (ICCC) (IEEE), 000233–000238.
Alexy, M., and Horvath, T. (2022). Tracing the local breeds in an outdoor system – a Hungarian example with Mangalica pig breed. Tracing Domest. Pig Work. Title. doi:10.5772/intechopen.101615
Alexy, M., Horvath, T., Reich, C., Felfoldi, J., and Tarcsi, A. (2019). “Adaption of data-intensive monitoring and tracking systems in outdoor pig production for better decision-making, literature review, and project idea,” in Precision livestock farming 2019-papers presented at the 9th European conference on precision livestock farming (ECPLF), 684–691.
Anonymous (1993). Second report on priorities for research and development in farm animal welfare. London, UK: Department of Environment, Food and Rural Affairs, Farm Animal Welfare Council FAWC.
Baert, S., Aubé, L., Haley, D. B., Bergeron, R., and Devillers, N. (2022). To wallow or nurse: sows housed outdoors have distinctive approaches to thermoregulation in gestation and lactation. Appl. Animal Behav. Sci. 248, 105575. doi:10.1016/j.applanim.2022.105575
Banhazi, T. M., and Black, J. L. (2009). Precision livestock farming: a suite of electronic systems to ensure the application of best practice management on livestock farms. Aust. J. Multi-Disciplinary Eng. 7 (1), 1–14. doi:10.1080/14488388.2009.11464794
Berckmans, D. (2014). Precision livestock farming technologies for welfare management in intensive livestock systems. Revue Sci. Tech. de l'OIE 33 (1), 189–196. PMID: 25000791. doi:10.20506/rst.33.1.2273
Bracke, M. B. M. (2011). Review of wallowing in pigs: description of the behaviour and its motivational basis. Appl. Animal Behav. Sci. 132, 1–13. doi:10.1016/j.applanim.2011.01.002
Dailey, J. W., and McGlone, J. J. (1997). Oral/nasal/facial and other behaviors of sows kept individually outdoors on pasture, soil, or indoors in gestation crates. Appl. Animal Behav. Sci. 52, 25–43. doi:10.1016/S0168-1591(96)01099-4
Drazic, I., Barisic, B., Mujakovic, N., and Čep, R. (2010). “Implementation of shishkin mesh in the modelling of spring-mass system,” in Sborník vědeckých prací Vysoké školy báňské - technické univerzity Ostrava. Řada strojní, Vysoká škola báňská – technická univerzita Ostrava, 49–52. ISSN 1210-0471.
Edwards, S. A. (2005). Product quality attributes associated with outdoor pig production. Livest. Prod. Sci. 94, 5–14. doi:10.1016/j.livprodsci.2004.11.028
Egerszegi, I., Ratky, J., Solti, L., and Brussow, K. P. (2003). Mangalica—an indigenous swine breed from Hungary (review). Arch. Anim. Breed. 46, 245–256. doi:10.5194/aab-46-245-2003
Harrell, J., and Frank, E. (2015). Regression modeling strategies: with applications to linear models, logistic and ordinal regression, and survival analysis. Springer.
Horrillo, A., Gaspar, P., Muñoz, Á., Escribano, M., and González, E. (2023). Fattening iberian pigs indoors vs. Outdoors: production performance and market value. Animals 13, 506. doi:10.3390/ani13030506
Hou, H., and Ma, L. (2010). The relationship management of information system outsourcing provider perspective. Industrial Eng. Eng. Manag. (IE&EM), 1760–1763. doi:10.1109/ICIEEM.2010.5645931
Lee, I., and Lee, K. (2015). The Internet of Things (IoT): applications, investments, and challenges for enterprises. Bus. Horizons 58, 431–440. doi:10.1016/j.bushor.2015.03.008
Mader, T. L., Davis, M. S., and Brown-Brandl, T. (2006). Environmental factors influencing heat stress in feedlot cattle. J. Animal Sci. 84, 712–719. doi:10.2527/2006.843712x
Mai, V., Vanderborght, B., Haidegger, T., Khamis, A., Bhargava, N., Boesl, D. B. O., et al. (2022). The role of robotics in achieving the united nations sustainable development goals—the experts’ meeting at the 2021 IEEE/RSJ IROS workshop [industry activities]. IEEE Robotics Automation Mag. 29, 92–107. doi:10.1109/mra.2022.3143409
Maselyne, J., Saeys, W., Briene, P., Mertens, K., Vangeyte, J., De Ketelaere, B., et al. (2016). Methods to construct feeding visits from RFID registrations of growing-finishing pigs at the feed trough. Comput. Electron. Agric. 128, 9–19. ISSN 0168-1699. doi:10.1016/j.compag.2016.08.010
Mason, A., Korostynska, O., Cordova-Lopez, L. E., Esper, I., Romanov, D., Ross, S., et al. (2021). “Meat Factory Cell: assisting meat processors address sustainability in meat production,” in 2021 IEEE 21st international symposium on computational intelligence and informatics (CINTI) (IEEE), 000103–000108.
Morrison, M. J., and Curkendall, L. D. (2001). in Apparatus and method for reading radio frequency identification transponders used for livestock identification and data collection. Editor U. S. Patent (United States: Aginfolink Holdings).
Munak, A. (2006). “Preface,” in CIGR handbook of agricultural engineering volume VI. Information technology (CIGR). Available from: https://elibrary.asabe.org/cigr_handbook.asp?confid=cigr2006.
R Core Team (2022). R: a language and environment for statistical computing. Vienna, Austria: R Foundation for Statistical Computing. Available from: https://www.R-project.org/.
Reiners, K., Hegger, A., Hessel, E. F., Böck, S., Wendl, G., and Van den Weghe, H. F. A. (2009). Application of RFID technology using passive H.F. transponders for the individual identification of weaned piglets at the feed trough. Comput. Electron. Agric. 68 (2), 178–184. doi:10.1016/j.compag.2009.05.010
Ruiz-Garcia, L., and Lunadei, L. (2011). The role of RFID in agriculture: applications, limitations and challenges. Comput. Electron. Agric. 79, 42–50. ISSN 0168-1699. doi:10.1016/j.compag.2011.08.010
Simpson, G. (2022). _gratia: graceful ggplot-Based Graphics and Other Functions for GAMs Fitted using mgcv_. R package version 0.7.3. Available from: https://gavinsimpson.github.io/gratia/.
St.Pierre, N. R., Cobanov, B., and Schnitkey, G. (2003). Economic losses from heat stress by US livestock industries. J. Dairy Sci. 86, E52–E77. ISSN 0022-0302. doi:10.3168/jds.S0022-0302(03)74040-5
Szabo, S., and Alexy, M. (2022). Practical aspects of weight measurement using image processing methods in waterfowl production. Agriculture 12 (11), 1869. doi:10.3390/agriculture12111869
Takacs, K., Mason, A., Cordova-Lopez, L. E., Alexy, M., Galambos, P., and Haidegger, T. (2023). Current safety legislation of Food processing smart robot systems–the red meat sector. Acta Polytech. Hung. 19, 249–267. doi:10.12700/aph.19.11.2022.11.13
ten Napel, J., van der Veen, A. A., Oosting, S. J., and Koerkamp, P. G. (2011). A conceptual approach to design livestock production systems for robustness to enhance sustainability. Livest. Sci. 139, 150–160. doi:10.1016/j.livsci.2011.03.007
Tzanidakis, C., Simitzis, P., Arvanitis, K., and Panagakis, P. (2021). An overview of the current trends in precision pig farming technologies. Livest. Sci. 249, 104530. ISSN 1871-1413. doi:10.1016/j.livsci.2021.104530
Umble, E. J., Haft, R. R., and Umble, M. M. (2003). Enterprise resource planning: “Implementation procedures and critical success factors”. Eur. J. Operational Res. 146 (2), 241–257. doi:10.1016/s0377-2217(02)00547-7
Voulodimos, A. S., Patrikakis, C. Z., Sideridis, A. B., Ntafis, V. A., and Xylouri, E. M. (2010). A complete farm management system based on animal identification using RFID technology. Comput. Electron. Agric. 70 (2), 380–388. doi:10.1016/j.compag.2009.07.009
Wegner, K., Lambertz, C., Das, G., Reiner, G., and Gauly, M. (2016). Effects of temperature and temperature-humidity index on the reproductive performance of sows during summer months under a temperate climate. Animal Sci. J. 87, 1334–1339. doi:10.1111/asj.12569
Keywords: outdoor pig production, IoT, RFID, machine learning, precision livestock farming
Citation: Alexy M, Pai RR, Ferenci T and Haidegger T (2024) The potential of RFID technology for tracking Mangalica pigs in the extensive farming system–a research from Hungary. Pastor. Res. Policy Pract. 14:12854. doi: 10.3389/past.2024.12854
Received: 15 February 2024; Accepted: 20 June 2024;
Published: 25 July 2024.
Edited by:
Carol Kerven, University College London, United KingdomCopyright © 2024 Alexy, Pai, Ferenci and Haidegger. This is an open-access article distributed under the terms of the Creative Commons Attribution License (CC BY). The use, distribution or reproduction in other forums is permitted, provided the original author(s) and the copyright owner(s) are credited and that the original publication in this journal is cited, in accordance with accepted academic practice. No use, distribution or reproduction is permitted which does not comply with these terms.
*Correspondence: Marta Alexy, abalord02@inf.elte.hu