- 1Biomedical Research Center, Qatar University, Doha, Qatar
- 2College of Medicine, QU Health, Qatar University, Doha, Qatar
Background: Sulfonylureas have been a longstanding pharmacotherapy in the management of type 2 diabetes, with potential benefits beyond glycemic control. Although sulfonylureas are effective, interindividual variability exists in drug response. Pharmacometabolomics is a potent method for elucidating variations in individual drug response. Identifying unique metabolites associated with treatment response can improve our ability to predict outcomes and optimize treatment strategies for individual patients. Our objective is to identify metabolic signatures associated with good and poor response to sulfonylureas, which could enhance our capability to anticipate treatment outcome.
Methods: In this cross-sectional study, clinical and metabolomics data for 137 patients with type 2 diabetes who are taking sulfonylurea as a monotherapy or a combination therapy were obtained from Qatar Biobank. Patients were empirically categorized according to their glycosylated hemoglobin levels into poor and good responders to sulfonylureas. To examine variations in metabolic signatures between the two distinct groups, we have employed orthogonal partial least squares discriminant analysis and linear models while correcting for demographic confounders and metformin usage.
Results: Good responders showed increased levels of acylcholines, gamma glutamyl amino acids, sphingomyelins, methionine, and a novel metabolite 6-bromotryptophan. Conversely, poor responders showed increased levels of metabolites of glucose metabolism and branched chain amino acid metabolites.
Conclusion: The results of this study have the potential to empower our knowledge of variability in patient response to sulfonylureas, and carry significant implications for advancing precision medicine in type 2 diabetes management.
Introduction
Sulfonylureas, the oldest oral antidiabetic agents, remain one of the most commonly prescribed drugs for the treatment of type 2 diabetes (T2D), along with metformin [1]. Sulfonylureas are recommended as an add-on to metformin therapy in most international guidelines, and they can be used in combination with all classes of oral antidiabetics except glinides [2]. The use of sulfonylureas as a monotherapy has decreased over time, and it is mainly endorsed for patients who cannot use metformin [3]. However, sulfonylureas have been found to have pleiotropic effects beyond their use in diabetes management which opens up the possibility of repurposing these drugs [4]. Sulfonylureas can also be used as third-line agents for the management of uncontrolled diabetes with dual combination therapy [5].
Sulfonylureas are insulin secretagogues that function through stimulating insulin secretion from the pancreatic beta-cell (β-cell). Specifically, sulfonylureas bind to their specific receptors on β-cell and inhibit the ATP-sensitive potassium channels (KATP) on the membrane of β-cell, causing potassium efflux to decrease and the β-cell membrane to depolarize. This depolarization leads to calcium channels opening which results in calcium influx and increased intracellular calcium leading to a contraction of the filaments of actomyosin responsible for the exocytosis of insulin, and ultimately insulin secretion [6]. Sulfonylureas are usually well tolerated, however, they may cause side effects such as hypoglycemia and weight gain [7].
It is noteworthy that a significant proportion of patients do not respond optimally to sulfonylurea treatment [8]. Pharmacogenomic studies have revealed the influence of genetic factors on individual responses to sulfonylureas. Genetic variants in genes like KCNQ1, KCNJ11, ABCC8, CYP2C9, and TCF7L2 can influence the therapeutic response and adverse effects of sulfonylureas [9, 10]. However, research has shown conflicting results across various studies, highlighting the complexity of understanding how genetic factors contribute to sulfonylurea responses [9]. Metabolomics is a rapidly evolving field with the potential to significantly impact the future of precision medicine. It offers valuable insights into individualized treatment strategies, enhances our understanding of diseases, and enables the assessment of therapeutic efficacy [11].
The objective of this study is to identify specific metabolic signatures that are correlated with good and poor responses to sulfonylureas. This is being pursued through a retrospective cross-sectional study that is focusing on identifying blood metabolites linked to each category of sulfonylureas response. Comprehending the role of these metabolites can help tailor sulfonylurea therapy, thereby enhancing treatment effectiveness, and advancing the progressive shift toward precision medicine in diabetes management.
Materials and methods
Data source and study participants
This research gathered information from individuals recruited at Qatar Biobank (QBB). The QBB database encompasses a comprehensive profile of Qatari citizens or long-term residents (≥15 years residing in Qatar), aged 18 years and above, within the State of Qatar. Detailed baseline socio-demographic, clinical and behavioral phenotypic data, as well a wide range of biochemical parameters were assessed at the central laboratory of Hamad Medical Corporation (HMC), which is accredited by the College of American Pathologists. QBB data also included questionnaires related to their history of diabetes, medication usage, and metabolomics data for more than 1,000 metabolites. The research was approved by the Institutional Review Boards of the Qatar Biobank. All participants provided informed consent. Among the participants, a total of 137 patients with Type 2 Diabetes who were taking sulfonylurea in monotherapy or combination therapy and had available metabolic data were selected and included in this study. Patients with incomplete or inconsistent medication records were excluded. Among them, 41 patients were on sulfonylurea monotherapy (Gliclazide), 63 on dual therapy (59 combined with metformin, 2 combined with sitagliptin, and 2 combined with pioglitazone), and 33 on triple therapy (28 combined with both metformin and sitagliptin, and 5 combined with both metformin and pioglitazone). Daily doses of gliclazide modified release range from 30 mg to 120 mg, and for gliclazide immediate release from 80 mg to 320 mg. Patients were empirically dichotomized according to their HbA1C levels, which is the most widely used measure of glycemic control [12] into poor responders (HbA1C ≥ 7), and good responders (HbA1C < 7) in accordance with the American Diabetes Association guidelines and previous studies [13, 14].
Metabolomics
All participant serum samples were subjected to untargeted metabolomics using established protocols by Metabolon [15]. Metabolites measurement was performed using a Thermo Scientific Q-Exactive high resolution/accurate mass spectrometer (Thermo Fisher Scientific, Inc., Waltham, MA, United States) interfaced with a heated electrospray ionization (HESI-II) source and Orbitrap mass analyzer operated at 35,000 mass resolution along with Waters ACQUITY ultra-performance liquid chromatography (UPLC) (Waters Corporation, Milford, MA, United States). A thorough explanation of the process has already been provided [15]. Hits were matched with pre-existing library entries of over 3,300 pure standard chemicals to identify the compounds. Compounds were divided into several groups according to their sources. Internal standards and quality checks have been previously published [16]. In short, to adjust for discrepancies in sample preparation and instrument performance, a combination of stable isotope-labeled chemicals was utilized as internal standards. The stability and repeatability of the procedure were tracked over time using quality control samples. To reduce variability and guarantee the integrity of the samples, a systematic methodology was employed for pre-analytical sample management including sample collection, storage, and preparation.
Statistical analysis
The metabolomics data were inverse rank normalized. SIMCA® and R software were used to conduct multivariate analysis and linear models respectively. Linear regression analysis was performed to for each metabolite as y and good/poor responder grouping variable as x. The model also contained age, gender, body mass index (BMI), metformin usage (yes/no) and principal components 1 and 2 as confounders. To ensure the robustness of our findings, we have meticulously adjusted our analysis to account for any confounding effects of concurrent metformin treatment. This adjustment is crucial as it isolates the specific metabolic effects attributable to sulfonylureas, thereby providing a clearer understanding of the metabolomic variations associated with their response. The nominal p-values were adjusted using the multiple testing correction method (False Discovery Rate, FDR). Statistical significance was defined as FDR < 0.05. Functional enrichment analysis was performed on all nominally significant metabolites using Wilcoxon sum of ranks test and was followed by p-value adjustment using FDR method. Additional details of the statistical analysis has previously been provided [17].
Results
General characteristics of participants
One hundred and thirty-seven patients with T2D were categorized into “poor responders” (n = 85) and “good responders” (n = 52) based on their HbA1C levels. Table 1 reveals significantly higher levels of fasting blood glucose, HbA1C, homeostatic model assessment of insulin resistance (HOMA-IR), and gamma-glutamyl transferase (GGT) in the poor response group when compared to the good response one; whereas the good response group reveals significantly higher levels of C-peptide and chloride.
Multivariate analysis of metabolites differentiating poor and good sulfonylurea responders
Non-targeted metabolomics analysis was performed to investigate the metabolic signatures of 137 patients with Type 2 Diabetes (T2D) who were taking sulfonylureas. Orthogonal partial least squares discriminant analysis (OPLS-DA) was used to identify the best distinguishing components between poor and good responders as shown in Figure 1. OPLS-DA showed one predictive and two orthogonal components, with the discriminatory component accounting for 85.2% of the variance between poor and good responders. Figure 2C shows the list of metabolites with VIP > 1.5.
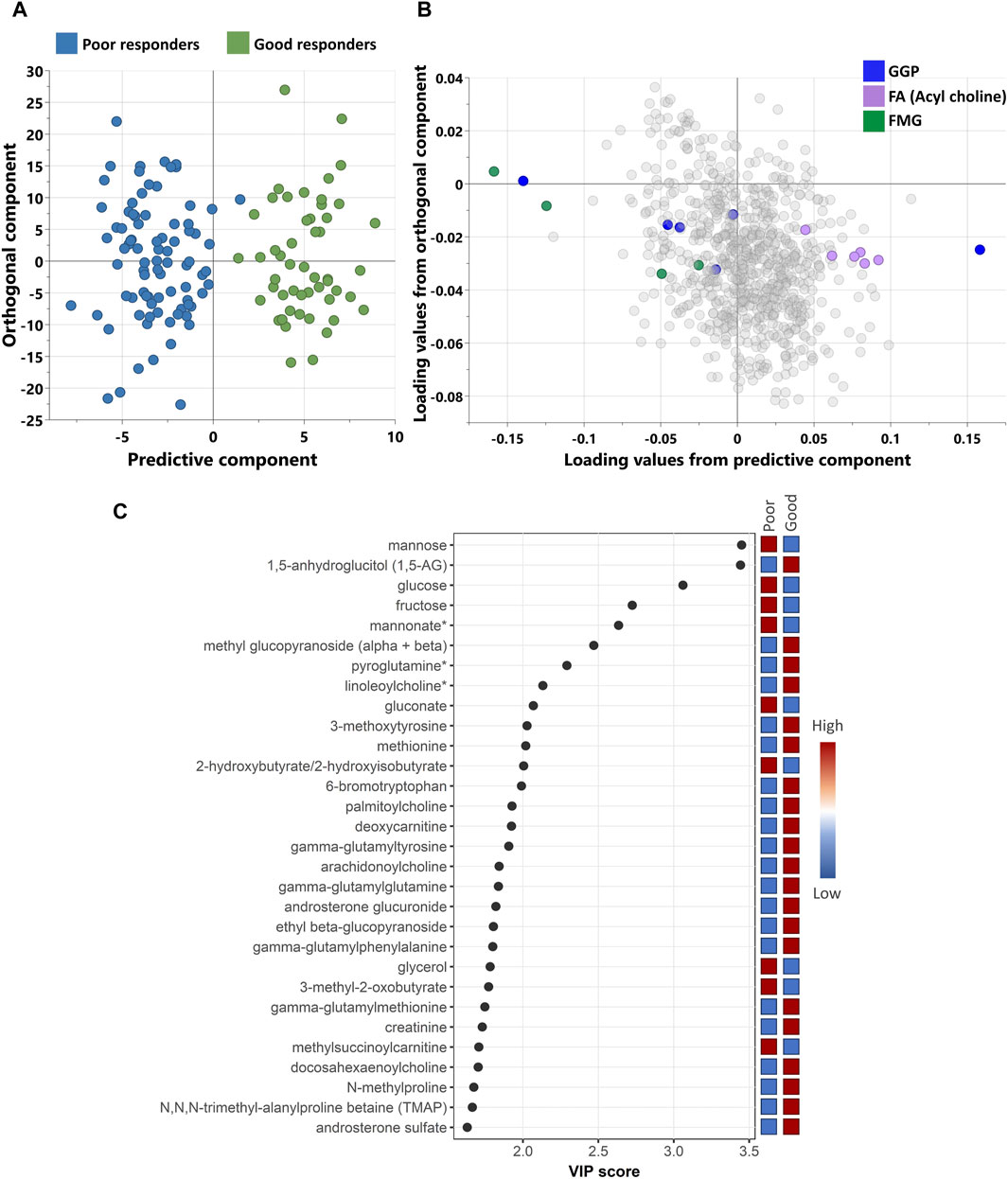
Figure 1. (A) scores and (B) loading plots from OPLS-DA between poor and good response. The model shows a good separation between the study groups with R2Y = 85.2% and Q2 = 49.1%. (C) A scatterplot of VIP score of the top 30 metabolites showing which metabolites are most useful in distinguishing between responders’ groups. (*) indicates a compound that has not been officially confirmed based on a standard but that Metabolon is confident in its identity. GGP, Glycolysis, Gluconeogenesis, and Pyruvate metabolism; FA, Fatty Acid metabolism; FMG, Fructose, Mannose and Galactose metabolism.
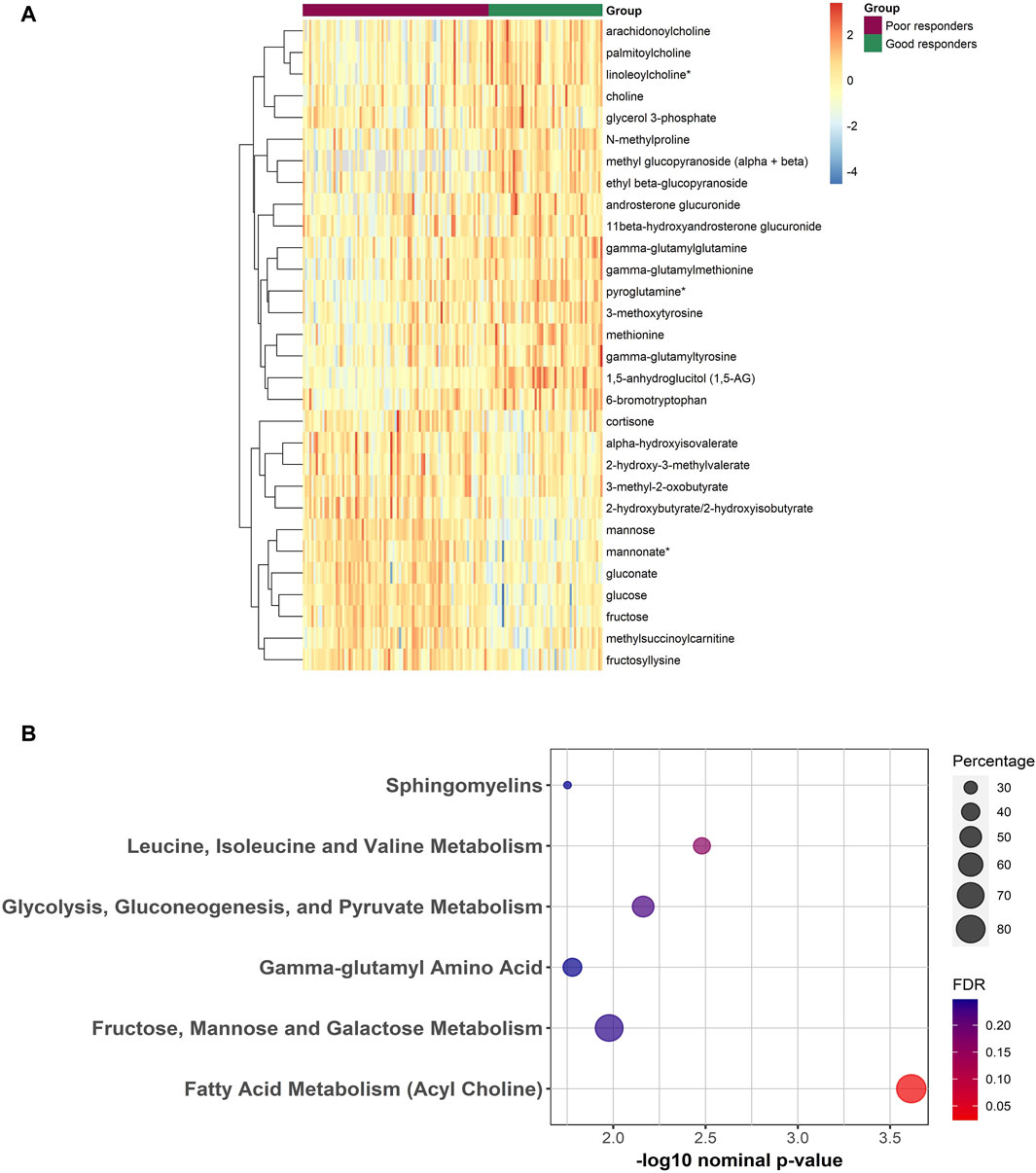
Figure 2. (A) Heatmap representing the abundance of FDR significant metabolites between poor and good responders from the linear regression analysis. Red/Blue correspond to more/less abundance, respectively. (B) Bubble plot depicting the enriched pathways from the functional enrichment analysis using Wilcoxon sum of ranks test. Percentage reflects the number of significantly different metabolites divided by total number of metabolites from that pathway.
Univariate analysis of metabolites differentiating poor and good sulfonylurea responders
Linear model analysis revealed a number of FDR (≤0.05) significant changes between the two studied groups (Table 2). This includes an increase in 1,5-anhydroglucitol, 6-bromotryptophan, 3-methoxytyrosine, methionine, pyroglutamine, ethyl and methyl glucopyranoside, gamma-glutamyl amino acids and acylcholines in the good responders group. Whereas an increase in glucose, mannose, fructose, mannonate, gluconate, fructosyl-lysine, and many branched chain amino acid metabolites was shown in the poor responders group. Supplementary Table S1 contains raw data values with standard deviations. The analysis conducted separately for males and females produced similar results except for 6-bromotryptophan which differentiate good responders from poor responders in males only (Supplementary Table S2).
Functional enrichment analysis
Results of functional enrichment analysis (Table 3) indicated significant differences in pathways of acylcholines, gamma-glutamyl amino acids, sphingomyelins, branched chain amino acid metabolites, fructose, mannose and galactose metabolism, and in glycolysis, gluconeogenesis, and pyruvate metabolism. Heatmap showing the top metabolites is also shown in Figure 2
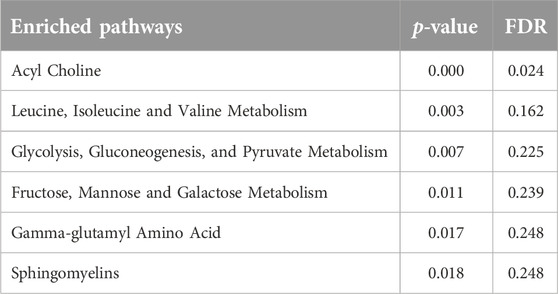
Table 3. Results from functional enrichment analysis based on metabolite ranks by p-value using the Fisher’s exact test.
Discussion
Sulfonylureas have been a longstanding option in the treatment armamentarium of T2D. Sulfonylureas exert their therapeutic effects on pancreatic beta cells by promoting calcium influx into these cells and ultimately increasing insulin secretion. Pharmacogenomics may offer some insight into the variable responses to sulfonylurea which lead to better or worse glycemic control. However, the current estimate suggests that genetics contributes to approximately 20%–40% of the individual variation in drug responses [18]. Pharmacometabolomics is sensitive to both genetic and environmental influences. Therefore, it is a potent method for elucidating variations in individual drug response. In this cross-sectional study, we have identified metabolic signatures associated with poor and good responders to sulfonylurea in a set of 137 samples from the Qatar Biobank. To the best of our knowledge, this study represents the first comparative analysis of the metabolic profiles between poor and good responders to sulfonylurea therapy.
Expectedly, good responders showed significant higher levels of 1,5-anhydroglucitol (1,5-AG), while poor responders showed higher levels of glucose, mannose, fructose, and fructosyl-lysine. These metabolites reflect the level of glycemia, and the regulated/dysregulated glucose metabolism in each response category [19]. Good responders exhibited significantly elevated levels of C-peptide, indicative of the effective functioning of pancreatic β-cells. Higher C-peptide levels have been associated with a better response to certain antidiabetics like metformin, sulfonylureas, and thiazolidinediones [20]. Poor responders showed higher levels of GGT; the increased concentrations of this enzyme has been associated with a poor glycemic control [21].
Remarkably, the good response group was associated with a reduction in branched-chain ketoacids (BCKA), and branched-chain alpha-hydroxy acids (BCHA) which are intermediates formed during the catabolism of branched chain amino acid (BCAA) [22]. These intermediates were associated with metabolic disorders [23], and recently have been shown to contribute to pathologic cardiac hypertrophy [24]. Given that the cardiovascular safety of sulfonylureas is still a topic of debate [25], it is important in future studies to consider the potential role of BCKA and BCHA in this context. BCKAs have also been shown to chronically suppress the serine/threonine kinase 2 (akt2) [26]. Strikingly, the activity of akt2 is essential for the proper functioning, survival, and adaptive growth of β-cells [27, 28]. Moreover, Sirtuin 4 (SIRT4) which plays a crucial role in the regulation of BCAA catabolism, is expressed in islets of Langerhans, and has been shown to control insulin secretion [29, 30]. This suggests a potential link between BCKA and BCHA with insulin secretion in the context of SU treatment, and opens up exciting new avenues for investigating their potential role in the health of β-cells.
Our emerging results showed an increase of many acylcholines in the sulfonylurea responsive group, supported by an FDR significant enriched metabolic pathway. Data on the biological activity of acylcholines remain very limited. Recently, they are discovered as endogenous modulators of the acetylcholine signaling system through the inhibition of the enzyme acetylcholinesterase [31]. Interestingly, sulfonylureas have been shown to inhibit acetylcholinesterase activity [4]. Acetylcholine is crucial for pancreatic beta cell function. It stimulates insulin secretion by acting on M3 muscarinic receptors on beta-cells [32], and thus increasing the concentration of cytoplasmic free calcium [33]. Considering that the M3 muscarinic receptors serve as promising targets for innovative antidiabetic medications, acylcholines could present novel opportunities in this context.
Interestingly, good responders to sulfonylureas exhibited higher levels of many gamma-glutamyl amino acids. These metabolites are dipeptides consisting of a C-terminal amino acid having a gamma-glutamyl residue attached at the N alpha-position. In recent years, gamma-glutamyl amino acids have caught researchers’ attention due to their ability to allosterically activate the calcium-sensing receptor (CaSR) [34]. CaSR is expressed in β-cells, and plays an important role in regulating its function by influencing expression and function of potassium and voltage-dependent calcium channels, and by controlling cell adhesion, coupling, and communication [35–37]. Further research is required to clarify the role of gamma-glutamyl amino acids in glucose homeostasis.
Our emerging results also showed that sphingomyelins metabolic pathway was nominally enriched in the responsive group. In fact, Sphingomyelins play a significant role in the function of pancreatic beta cells. They are involved in regulating beta-cell excitability and insulin exocytosis [38]. Griess et al. demonstrated that a β-cell-specific ablation of ceramide synthase 2, the enzyme necessary for generation of very-long-chain sphingolipids, selectively reduces insulin content and impairs insulin secretion [39]. Khan et al. reported that a downregulated sphingolipid impairs pancreatic β cell function [40]. Several phospholipids, particularly sphingomyelins, were shown to be significantly lower in the serum of children who later progress to T1DM [41]. Additionally, a beta-cell-specific antibody has been found to target unique sphingomyelin patches on the surface of live cells [42]. Interestingly, a deficiency in the enzyme sphingomyelin synthase 1 leads to a reduction in KCNQ1 expression, and the phospho-head group in sphingomyelin is essential for the proper gating of certain voltage-dependent K+ channels [43]. Sphingomyelins are also shown to be associated with ATP-binding cassette (ABC) proteins, and ABCA7 deficiency impairs sphingomyelin synthesis [44]. A review stipulated that lower sphingomyelin may be associated with higher risks of coronary heart disease and T2D [30]. We hypothesize that elevated sphingomyelin could indicate an improved β cell function reflecting a good response to sulfonylureas, which might be associated with a lower risk of heart disease.
Univariate analysis showed the association of gut-microbiota metabolites, namely, ethyl glucopyranoside, methyl glucopyranoside, mannonate and gluconate, with the response to sulfonylureas. While no study has yet explored the direct impact of sulfonylureas on the gut microbiota, recent research indicates that sulfonylureas may indeed have some interaction with the gut microbiota [45]. Research also highlights the role of gut microbiota-derived metabolites in influencing β-cell function by playing a crucial role in protecting β-cell and promoting insulin secretion [46]. Interestingly, ethyl and methyl glucopyranoside associated in our study with the good responders to sulfonylureas, were identified to be associated with better microbiome diversity and a healthier status [47]. While a review concluded the lack of an alteration in the gut microbiota by sulfonylureas [48], it is important to note that the interaction between sulfonylureas and gut microbiota may not be bidirectional. Our hypothesis suggests that a dysbiosis in the gut microbiota might be linked to a poor response to sulfonylureas.
Univariate analysis also showed the association of pyroglutamine, methionine, and 3-metoxytyrosine with the good response group. Indeed, pyroglutamine (also called 5-oxoproline) is involved in glutathione metabolism, which plays a role in maintaining redox homeostasis in pancreatic β-cells [49]. Methionine is known to regulate insulin secretion from pancreatic β-cells [50], and methionine accumulation in the pancreas has been reported to represent β-cell function [51]. The metabolite 3-metoxytyrosine is a product in the metabolism of dopamine, the latter has been reported to regulate pancreatic insulin secretion via adrenergic and dopaminergic receptors [52]. However, the data on the function of 3-metoxytyrosine remains very scarce, and much work still needs to be done to elucidate its exact functions and significance.
Strikingly, our results showed a significant association between a novel metabolite, namely, 6-bromotryptophan with the good response group especially in males. 6-bromotryptophan is a metabolite derived from the bromination of tryptophan. While it is believed that 6-bromotryptophan is a microbiome-derived metabolite, its formation and the localization of this reaction are not well understood. It is accepted that 6-bromotryptophan has a strong relationship with fat distribution [53], and recently, it has been associated with lower risk for chronic kidney progression in multiple studies [54]. Taking into consideration that low urinary chloride concentration was associated with a higher risk for CKD progression [55], our results are corroborated with the significant difference (p < 0.001) in chloride levels between good and poor responders. The BROMO clinical trial (NCT05971524) is currently underway to investigate the safety, pharmacokinetics, and efficacy of dietary supplement of 6-bromotryptophan in individuals with metabolic syndrome. Interestingly, the same clinical trial claims that 6-bromotryptophan was positively associated with C-peptide and preserved β-cell function. Relatedly, a study on the therapeutic applications of a 6-bromotryptophan-containing conotoxin demonstrated its bioactivity on insulin release in pancreatic β-cell [56]. Indeed, tryptophan is necessary in all KCNQ subunits to confer drug sensitivity [57]. Moreover, the variant rs7903146 in TCF7L2 gene has been associated with the therapeutic response to sulfonylureas. The rs7903146 T-allele conferred a higher risk for sulfonylurea treatment failure by impairing β-cell function [58, 59]. Interestingly, serotonin, synthesized solely from tryptophan, was identified as the top metabolite being increased in carriers of the rs7903146 risk allele [60]. It is important to note that an allosteric modulator of β-cell M3 muscarinic receptors (VU0119498) [61], which stimulates insulin release and improves glucose homeostasis, has a similar structure to 6-bromotryptophan. Taken together with the literature, we present evidence supporting the involvement of tryptophan metabolism in pancreatic β-cell function. The proxy metabolite 6-bromotryptophan may serve as an indicator of a positive response, or even as a candidate for drug development. Nevertheless, further research is essential to clarify the connection between 6-bromotryptophan and its related metabolites, and the response to sulfonylurea treatment.
Conclusion
This study offers the research community with a wealth of metabolic biomarkers associated with response to sulfonylurea, notably branched chain amino acid metabolites, sphingomyelins, acylcholines, gamma glutamyl amino acids, and the novel metabolite 6-bromotryptophan. The study’s findings support the development of biomarker-driven treatment strategies that can lead to more personalized and effective diabetes management, and we believe these findings can be translated into clinically relevant metabolic signatures, enabling further research into personalized therapeutic approaches.
While the confounding effect of metformin has been corrected in this study, we acknowledge the presence of some limitations. One limitation of this study is its cross-sectional design, which makes it challenging to determine if individuals with elevated HbA1C levels exhibited a poor response to sulfonylurea, or had more advanced diabetes prior to starting treatment. Consequently, certain metabolites may be linked to the progression or complications of diabetes rather than treatment response. Moreover, the QBB data lacks baseline metabolomics information, making it difficult to compare metabolic profiles before and after treatment. Additionally, the variability in sulfonylurea dosages across participants, and the absence of data regarding the duration of treatment, present another potential limitation. Future research involving longitudinal studies will thus be warranted to validate our identified biomarkers.
Data availability statement
The data analyzed in this study is subject to the following licenses/restrictions: The datasets used and/or analyzed during the current study are available from the corresponding author on reasonable request. Requests to access these datasets should be directed to bS5lbHJheWVzc0BxdS5lZHUucWE=
Ethics statement
The studies involving humans were approved by Institutional Review Boards of the Qatar Biobank (E-2023-QF-QBB-RES-ACC-00125-0222). The studies were conducted in accordance with the local legislation and institutional requirements. The participants provided their written informed consent to participate in this study.
Author contributions
Writing original draft, KN; Statistical analysis, NA; Review and editing, SB; Review and editing, supervision, project administration, funding acquisition, ME. All authors contributed to the article and approved the submitted version.
Funding
The authors declare financial support was received for the research, authorship, and/or publication of this article. This research was funded by Qatar Research, Development and Innovation (QRDI) Council, grant number PPM-06-0516-230030.
Acknowledgments
Authors would like to acknowledge Qatar Biobank for providing the data.
Conflict of interest
The authors declare that the research was conducted in the absence of any commercial or financial relationships that could be construed as a potential conflict of interest.
Supplementary material
The Supplementary Material for this article can be found online at: https://www.frontierspartnerships.org/articles/10.3389/jpps.2024.13305/full#supplementary-material
References
1. Thulé, PM, and Umpierrez, G. Sulfonylureas: a new look at old therapy. Curr Diab Rep (2014) 14:473. doi:10.1007/s11892-014-0473-5
2. Mohan, V, Saboo, B, Khader, J, Modi, KD, Jindal, S, Wangnoo, SK, et al. Position of sulfonylureas in the current ERA: review of national and international guidelines. Clin Med Insights: Endocrinol Diabetes (2022) 15:117955142210746. doi:10.1177/11795514221074663
3. Hemmingsen, B, Schroll, JB, Wetterslev, J, Gluud, C, Vaag, A, Sonne, DP, et al. Sulfonylurea versus metformin monotherapy in patients with type 2 diabetes: a cochrane systematic review and meta-analysis of randomized clinical trials and trial sequential analysis. CMAJ Open (2014) 2:E162–75. doi:10.9778/cmajo.20130073
4. Baruah, P, Das, A, Paul, D, Chakrabarty, S, Aguan, K, and Mitra, S. Sulfonylurea class of antidiabetic drugs inhibit acetylcholinesterase activity: unexplored auxiliary pharmacological benefit toward alzheimer’s disease. ACS Pharmacol and Translational Sci (2021) 4:193–205. doi:10.1021/acsptsci.0c00168
5. Kalra, S, Bahendeka, S, Sahay, R, Ghosh, S, Md, F, Orabi, A, et al. Consensus recommendations on sulfonylurea and sulfonylurea combinations in the management of type 2 diabetes mellitus - international task force. Indian J Endocrinol Metab (2018) 22:132–57. doi:10.4103/ijem.ijem_556_17
6. Lv, W, Wang, X, Xu, Q, and Lu, W. Mechanisms and characteristics of sulfonylureas and glinides. Curr Top Med Chem (2020) 20:37–56. doi:10.2174/1568026620666191224141617
7. Sola, D, Rossi, L, Schianca, GP, Maffioli, P, Bigliocca, M, Mella, R, et al. Sulfonylureas and their use in clinical practice. Arch Med Sci (2015) 11:840–8. doi:10.5114/aoms.2015.53304
8. Aquilante, CL. Sulfonylurea pharmacogenomics in Type 2 diabetes: the influence of drug target and diabetes risk polymorphisms. Expert Rev Cardiovasc Ther (2010) 8:359–72. doi:10.1586/erc.09.154
9. Karkhaneh, L, Tabatabaei-Malazy, O, Bandarian, F, Mohseni, S, and Larijani, B. Pharmacogenomics of sulfonylureas in type 2 diabetes mellitus; a systematic review. J Diabetes Metab Disord (2021) 21:863–79. doi:10.1007/s40200-021-00908-x
10. Zeng, Z, Huang, S-Y, and Sun, T. Pharmacogenomic studies of current antidiabetic agents and potential new drug targets for precision medicine of diabetes. Diabetes Ther (2020) 11:2521–38. doi:10.1007/s13300-020-00922-x
11. Mussap, M, Loddo, C, Fanni, C, and Fanos, V. Metabolomics in pharmacology - a delve into the novel field of pharmacometabolomics. Expert Rev Clin Pharmacol (2020) 13:115–34. doi:10.1080/17512433.2020.1713750
12. Spencer, CC, Bennett, AJ, Coleman, RL, Tavendale, R, Hawley, SA, Donnelly, LA, et al. Common variants near ATM are associated with glycemic response to metformin in type 2 diabetes. Nat Genet (2011) 43:117–20. doi:10.1038/ng.735
13. ElSayed, NA, Aleppo, G, Aroda, VR, Bannuru, RR, Brown, FM, Bruemmer, D, et al. 6. Glycemic targets: standards of care in diabetes—2023. Diabetes Care (2023) 46:S97–S110. doi:10.2337/dc23-s006
14. Soeters, MR, Hoekstra, JB, and de Vries, JH. HbA1c: target value should remain 7%. Ned Tijdschr Geneeskd (2010) 154:A2113.
15. Al-Khelaifi, F, Diboun, I, Donati, F, Botrè, F, Alsayrafi, M, Georgakopoulos, C, et al. A pilot study comparing the metabolic profiles of elite-level athletes from different sporting disciplines. Sports Med Open (2018) 4:2. doi:10.1186/s40798-017-0114-z
16. Evans, A, Bridgewater, B, Liu, Q, Mitchell, M, Robinson, R, Dai, H, et al. High resolution mass spectrometry improves data quantity and quality as compared to unit mass resolution mass spectrometry in high-throughput profiling metabolomics. Metabolomics (2014) 4. doi:10.4172/2153-0769.1000132
17. Naja, K, Anwardeen, N, Al-Hariri, M, Al Thani, AA, and Elrayess, MA. Pharmacometabolomic approach to investigate the response to metformin in patients with type 2 diabetes: a cross-sectional study. Biomedicines (2023) 11:2164. doi:10.3390/biomedicines11082164
18. Beger, RD, Schmidt, MA, and Kaddurah-Daouk, R. Current concepts in pharmacometabolomics, biomarker Discovery, and precision medicine. Metabolites (2020) 10:129. doi:10.3390/metabo10040129
19. Villena Chávez, JE, Neira Sánchez, ER, and Poletti Ferrara, LF. Dispersion of serum 1,5 anhydroglucitol values in patients with type 2 diabetes at goal of HbA1c. Diabetes Res Clin Pract (2023) 199:110668. doi:10.1016/j.diabres.2023.110668
20. Leighton, E, Sainsbury, CA, and Jones, GC. A practical review of C-peptide testing in diabetes. Diabetes Ther (2017) 8:475–87. doi:10.1007/s13300-017-0265-4
21. Gohel, MG, and Chacko, AN. Serum GGT activity and hsCRP level in patients with type 2 diabetes mellitus with good and poor glycemic control: an evidence linking oxidative stress, inflammation and glycemic control. J Diabetes Metab Disord (2013) 12:56. doi:10.1186/2251-6581-12-56
22. Neinast, M, Murashige, D, and Arany, Z. Branched chain amino acids. Annu Rev Physiol (2019) 81:139–64. doi:10.1146/annurev-physiol-020518-114455
23. Newgard, CB. Metabolomics and metabolic diseases: where do we stand? Cel Metab (2017) 25:43–56. doi:10.1016/j.cmet.2016.09.018
24. Walejko, JM, Christopher, BA, Crown, SB, Zhang, G-F, Pickar-Oliver, A, Yoneshiro, T, et al. Branched-chain α-ketoacids are preferentially reaminated and activate protein synthesis in the heart. Nat Commun (2021) 12:1680. doi:10.1038/s41467-021-21962-2
25. Wexler, DJ. Sulfonylureas and cardiovascular safety: the final verdict? JAMA (2019) 322:1147–9. doi:10.1001/jama.2019.14533
26. Zhao, H, Zhang, F, Sun, D, Wang, X, Zhang, X, Zhang, J, et al. Branched-chain amino acids exacerbate obesity-related hepatic glucose and lipid metabolic disorders via attenuating Akt2 signaling. Diabetes (2020) 69:1164–77. doi:10.2337/db19-0920
27. Elghazi, L, Balcazar, N, and Bernal-Mizrachi, E. Emerging role of protein kinase B/Akt signaling in pancreatic β-cell mass and function. The Int J Biochem and Cel Biol (2006) 38:689–95. doi:10.1016/j.biocel.2006.01.005
28. Blandino-Rosano, M, Alejandro, EU, Sathyamurthy, A, Scheys, JO, Gregg, B, Chen, AY, et al. Enhanced beta cell proliferation in mice overexpressing a constitutively active form of Akt and one allele of p21Cip. Diabetologia (2012) 55:1380–9. doi:10.1007/s00125-012-2465-9
29. Zaganjor, E, Vyas, S, and Haigis, MC. SIRT4 is a regulator of insulin secretion. Cel Chem Biol (2017) 24:656–8. doi:10.1016/j.chembiol.2017.06.002
30. Anderson, KA, Huynh, FK, Fisher-Wellman, K, Stuart, JD, Peterson, BS, Douros, JD, et al. SIRT4 is a lysine deacylase that controls leucine metabolism and insulin secretion. Cel Metab (2017) 25:838–55.e15. doi:10.1016/j.cmet.2017.03.003
31. Akimov, MG, Kudryavtsev, DS, Kryukova, EV, Fomina-Ageeva, EV, Zakharov, SS, Gretskaya, NM, et al. Arachidonoylcholine and other unsaturated long-chain acylcholines are endogenous modulators of the acetylcholine signaling system. Biomolecules (2020) 10:283. doi:10.3390/biom10020283
32. Zhu, L, Rossi, M, Doliba, NM, and Wess, J. Beta-cell M3 muscarinic acetylcholine receptors as potential targets for novel antidiabetic drugs. Int Immunopharmacology (2020) 81:106267. doi:10.1016/j.intimp.2020.106267
33. Rodriguez-Diaz, R, Dando, R, Jacques-Silva, MC, Fachado, A, Molina, J, Abdulreda, MH, et al. Alpha cells secrete acetylcholine as a non-neuronal paracrine signal priming beta cell function in humans. Nat Med (2011) 17:888–92. doi:10.1038/nm.2371
34. Guha, S, and Majumder, K. Comprehensive review of γ-glutamyl peptides (γ-GPs) and their effect on inflammation concerning cardiovascular health. J Agric Food Chem (2022) 70:7851–70. doi:10.1021/acs.jafc.2c01712
35. Squires, P, Jones, P, Younis, M, and Hills, C. The calcium-sensing receptor and β-cell function. Vitamins Horm (2014) 95:249–67. doi:10.1016/B978-0-12-800174-5.00010-7
36. Hodgkin, MN, Hills, CE, and Squires, PE. The calcium-sensing receptor and insulin secretion: a role outside systemic control 15 years on. J Endocrinol (2008) 199:1–4. doi:10.1677/joe-08-0261
37. Squires, PE, Jones, PM, Younis, MY, and Hills, CE. The calcium-sensing receptor and β-cell function. Vitam Horm (2014) 95:249–67. doi:10.1016/B978-0-12-800174-5.00010-7
38. Véret, J, Bellini, L, Giussani, P, Ng, C, Magnan, C, and Stunff, H. Roles of sphingolipid metabolism in pancreatic β cell dysfunction induced by lipotoxicity. J Clin Med (2014) 3:646–62. doi:10.3390/jcm3020646
39. Griess, K, Rieck, M, Müller, N, Karsai, G, Hartwig, S, Pelligra, A, et al. Sphingolipid subtypes differentially control proinsulin processing and systemic glucose homeostasis. Nat Cel Biol (2023) 25:20–9. doi:10.1038/s41556-022-01027-2
40. Khan, SR, Manialawy, Y, Obersterescu, A, Cox, BJ, Gunderson, EP, and Wheeler, MB. Diminished sphingolipid metabolism, a hallmark of future type 2 diabetes pathogenesis, is linked to pancreatic β cell dysfunction. iScience (2020) 23:101566. doi:10.1016/j.isci.2020.101566
41. Sen, P, Dickens, AM, López-Bascón, MA, Lindeman, T, Kemppainen, E, Lamichhane, S, et al. Metabolic alterations in immune cells associate with progression to type 1 diabetes. Diabetologia (2020) 63:1017–31. doi:10.1007/s00125-020-05107-6
42. Kavishwar, A, Medarova, Z, and Moore, A. Unique sphingomyelin patches are targets of a beta-cell-specific antibody. J Lipid Res (2011) 52:1660–71. doi:10.1194/jlr.m017582
43. Wu, M, Takemoto, M, Taniguchi, M, Takumi, T, Okazaki, T, and Song, WJ. Regulation of membrane KCNQ1/KCNE1 channel density by sphingomyelin synthase 1. Am J Physiology-Cell Physiol (2016) 311:C15–23. doi:10.1152/ajpcell.00272.2015
44. Iqbal, J, Suarez, MD, Yadav, PK, Walsh, MT, Li, Y, Wu, Y, et al. ATP-binding cassette protein ABCA7 deficiency impairs sphingomyelin synthesis, cognitive discrimination, and synaptic plasticity in the entorhinal cortex. J Biol Chem (2022) 298:102411. doi:10.1016/j.jbc.2022.102411
45. Whang, A, Nagpal, R, and Yadav, H. Bi-directional drug-microbiome interactions of anti-diabetics. EBioMedicine (2019) 39:591–602. doi:10.1016/j.ebiom.2018.11.046
46. Fernández-Millán, E, and Guillén, C. Multi-organ crosstalk with endocrine pancreas: a focus on how gut microbiota shapes pancreatic beta-cells. Biomolecules (2022) 12:104. doi:10.3390/biom12010104
47. Wilmanski, T, Rappaport, N, Earls, JC, Magis, AT, Manor, O, Lovejoy, J, et al. Blood metabolome predicts gut microbiome α-diversity in humans. Nat Biotechnol (2019) 37:1217–28. doi:10.1038/s41587-019-0233-9
48. Kant, R, Chandra, L, Verma, V, Nain, P, Bello, D, Patel, S, et al. Gut microbiota interactions with anti-diabetic medications and pathogenesis of type 2 diabetes mellitus. World J Methodol (2022) 12:246–57. doi:10.5662/wjm.v12.i4.246
49. Azarova, I, Polonikov, A, and Klyosova, E. Molecular genetics of abnormal redox homeostasis in type 2 diabetes mellitus. Int J Mol Sci (2023) 24:4738. doi:10.3390/ijms24054738
50. Newsholme, P, Brennan, L, and Bender, K. Amino acid metabolism, β-cell function, and diabetes. Diabetes (2006) 55:S39–S47. doi:10.2337/db06-s006
51. Steiner, E, Kazianka, L, Breuer, R, Hacker, M, Wadsak, W, Mitterhauser, M, et al. **-Postprandial pancreatic [(11)C]methionine uptake after pancreaticoduodenectomy mirrors basal beta cell function and insulin release. Eur J Nucl Med Mol Imaging (2017) 44:509–16. doi:10.1007/s00259-016-3451-0
52. Aslanoglou, D, Bertera, S, Sánchez-Soto, M, Benjamin Free, R, Lee, J, Zong, W, et al. Dopamine regulates pancreatic glucagon and insulin secretion via adrenergic and dopaminergic receptors. Transl Psychiatry (2021) 11:59. doi:10.1038/s41398-020-01171-z
53. Zheng, R, Michaëlsson, K, Fall, T, Elmståhl, S, and Lind, L. The metabolomic profiling of total fat and fat distribution in a multi-cohort study of women and men. Sci Rep (2023) 13:11129. doi:10.1038/s41598-023-38318-z
54. Sekula, P, Tin, A, Schultheiss, UT, Baid-Agrawal, S, Mohney, RP, Steinbrenner, I, et al. Urine 6-bromotryptophan: associations with genetic variants and incident end-stage kidney disease. Scientific Rep (2020) 10:10018. doi:10.1038/s41598-020-66334-w
55. Joo, YS, Kim, J, Park, CH, Yun, HR, Park, JT, Chang, TI, et al. Urinary chloride concentration and progression of chronic kidney disease: results from the Korean cohort study for Outcomes in patients with Chronic Kidney Disease. Nephrol Dial Transplant (2021) 36:673–80. doi:10.1093/ndt/gfz247
56. Lugo-Fabres, PH, Otero-Sastre, LM, Bernáldez-Sarabia, J, Camacho-Villegas, TA, Sánchez-Campos, N, Serrano-Bello, J, et al. Potential therapeutic applications of synthetic conotoxin s-cal14.2b, derived from californiconus californicus, for treating type 2 diabetes. Biomedicines (2021) 9:936. doi:10.3390/biomedicines9080936
57. Schenzer, A, Friedrich, T, Pusch, M, Saftig, P, Jentsch, TJ, Grötzinger, J, et al. Molecular determinants of KCNQ (Kv7) K+ channel sensitivity to the anticonvulsant retigabine. J Neurosci (2005) 25:5051–60. doi:10.1523/jneurosci.0128-05.2005
58. Holstein, A, Hahn, M, Körner, A, Stumvoll, M, and Kovacs, P. TCF7L2 and therapeutic response to sulfonylureas in patients with type 2 diabetes. BMC Med Genet (2011) 12:30. doi:10.1186/1471-2350-12-30
59. Cropano, C, Santoro, N, Groop, L, Dalla Man, C, Cobelli, C, Galderisi, A, et al. The rs7903146 variant in the TCF7L2 gene increases the risk of prediabetes/type 2 diabetes in obese adolescents by impairing β-cell function and hepatic insulin sensitivity. Diabetes Care (2017) 40:1082–9. doi:10.2337/dc17-0290
60. Leiherer, A, Muendlein, A, Saely, CH, Fraunberger, P, and Drexel, H. Serotonin is elevated in risk-genotype carriers of TCF7L2 - rs7903146. Scientific Rep (2019) 9:12863. doi:10.1038/s41598-019-49347-y
Keywords: sulfonylureas, type 2 diabetes, metabolic signatures, precision medicine, metabolomics
Citation: Naja K, Anwardeen N, Bashraheel SS and Elrayess MA (2024) Pharmacometabolomics of sulfonylureas in patients with type 2 diabetes: a cross-sectional study. J. Pharm. Pharm. Sci 27:13305. doi: 10.3389/jpps.2024.13305
Received: 26 May 2024; Accepted: 10 September 2024;
Published: 17 September 2024.
Edited by:
John Reyes Ussher, University of Alberta, CanadaCopyright © 2024 Naja, Anwardeen, Bashraheel and Elrayess. This is an open-access article distributed under the terms of the Creative Commons Attribution License (CC BY). The use, distribution or reproduction in other forums is permitted, provided the original author(s) and the copyright owner(s) are credited and that the original publication in this journal is cited, in accordance with accepted academic practice. No use, distribution or reproduction is permitted which does not comply with these terms.
*Correspondence: Mohamed A. Elrayess, bS5lbHJheWVzc0BxdS5lZHUucWE=